A novel artificial fish swarm algorithm for pattern recognition with convex optimization
2016 International Conference on Communication and Electronics Systems (ICCES)(2016)
摘要
Image pattern recognition is an important area in digital image processing. An efficient pattern recognition algorithm should be able to provide correct recognition at a reduced computational time. Off late amongst the machine learning pattern recognition algorithms, Artificial fish swarm algorithm is one of the swarm intelligence optimization algorithms that works based on population and stochastic search. In order to achieve acceptable result, there are many parameters needs to be adjusted in AFSA. Among these parameters, visual and step are very significant in view of the fact that artificial fish basically move based on these parameters. In standard AFSA, these two parameters remain constant until the algorithm termination. Large values of these parameters increase the capability of algorithm in global search, while small values improve the local search ability of the algorithm. In this paper, we empirically study the performance of the AFSA and different approaches to balance between local and global exploration have been tested based on the adaptive modification of visual and step during algorithm execution. The proposed approaches have been evaluated based on the four well-known benchmark functions. Experimental results show considerable positive impact on the performance of AFSA. A Convex optimization has been integrated into the proposed work to have an ideal segmentation of the input image which is a MR brain image.
更多查看译文
关键词
Image segmentation,fish school algorithms,convex optimization,recognition rates
AI 理解论文
溯源树
样例
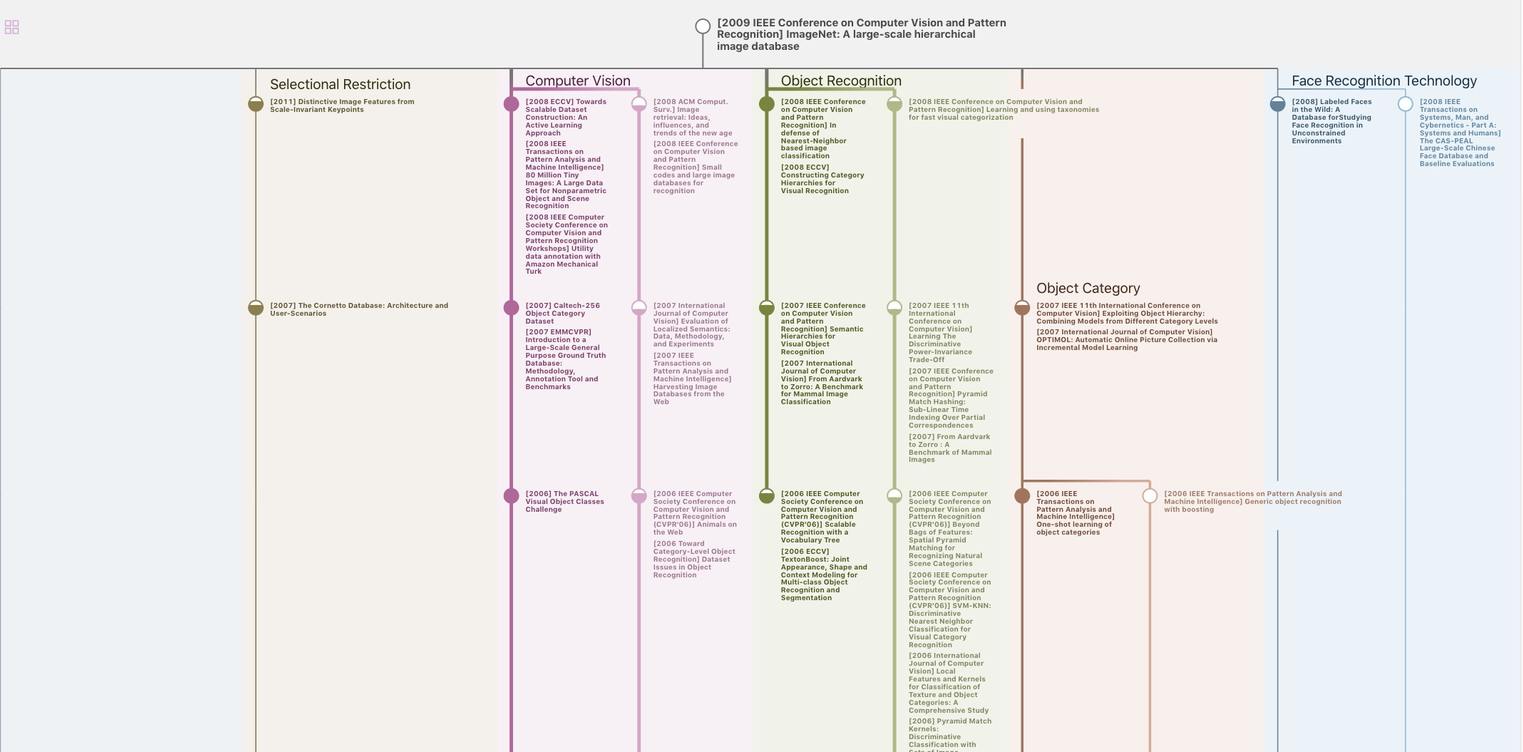
生成溯源树,研究论文发展脉络
Chat Paper
正在生成论文摘要