OctNet: Learning Deep 3D Representations at High Resolutions
CVPR(2017)
摘要
We present OctNet, a representation for deep learning with sparse 3D data. In contrast to existing models, our representation enables 3D convolutional networks which are both deep and high resolution. Towards this goal, we exploit the sparsity in the input data to hierarchically partition the space using a set of unbalanced octrees where each leaf node stores a pooled feature representation. This allows to focus memory allocation and computation to the relevant dense regions and enables deeper networks without compromising resolution. We demonstrate the utility of our OctNet representation by analyzing the impact of resolution on several 3D tasks including 3D object classification, orientation estimation and point cloud labeling.
更多查看译文
关键词
deep 3D representations,high resolutions,deep learning,sparse 3D data,3D convolutional networks,deep resolution,unbalanced octrees,memory allocation,deeper networks,OctNet representation,3D object classification,feature representation,orientation estimation,point cloud labeling
AI 理解论文
溯源树
样例
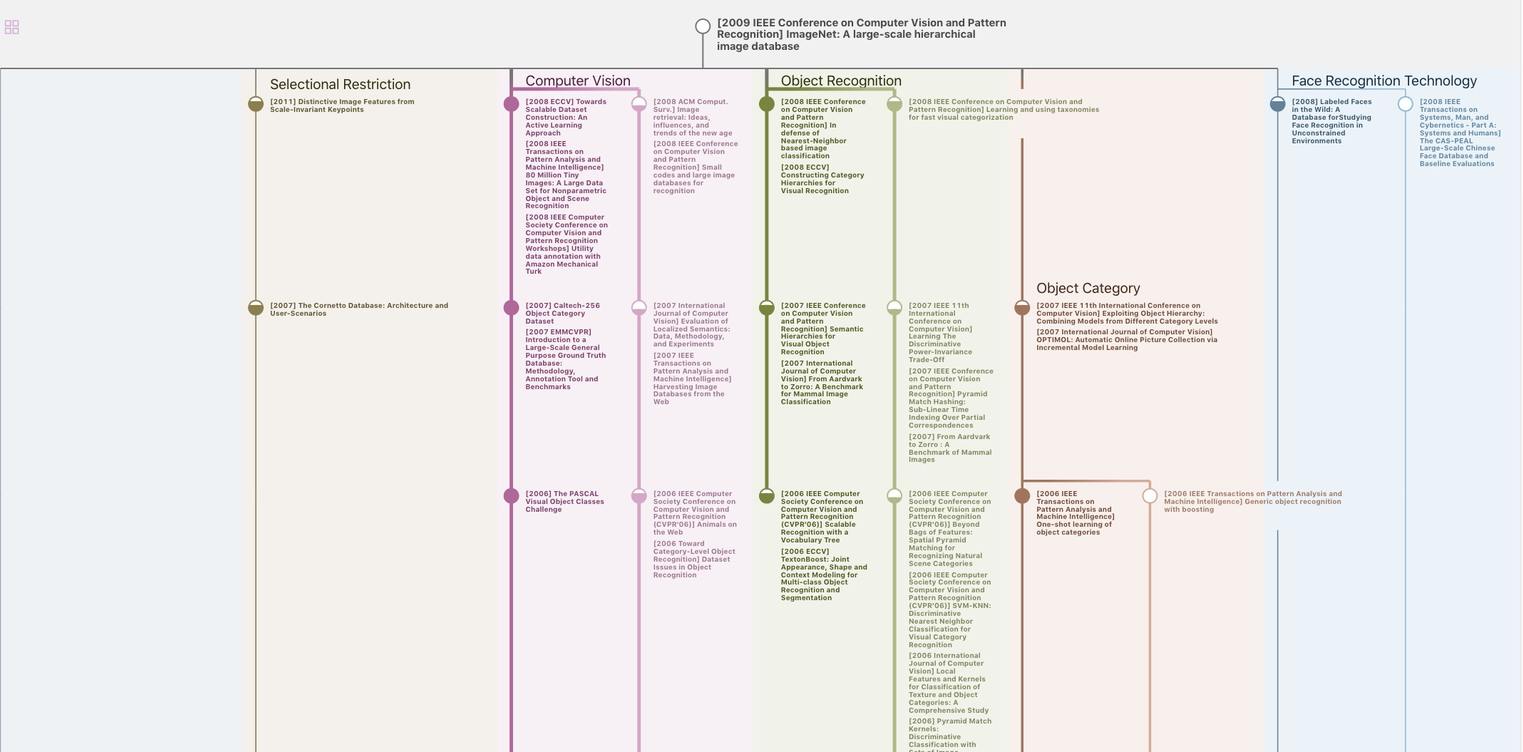
生成溯源树,研究论文发展脉络
Chat Paper
正在生成论文摘要