Rate Optimal Estimation And Confidence Intervals For High-Dimensional Regression With Missing Covariates
Journal of Multivariate Analysis(2019)
摘要
We consider the problems of estimation and of constructing component-wise confidence intervals in a sparse high-dimensional linear regression model when some covariates of the design matrix are missing completely at random. We analyze a variant of the Dantzig selector for estimating the regression model and we use a de-biasing argument to construct component-wise confidence intervals. We also complement our mathematical study in the supplementary materials with extensive simulations on synthetic and semisynthetic data that show the accuracy of our asymptotic predictions for finite sample sizes. (C) 2019 Published by Elsevier Inc.
更多查看译文
关键词
Dantzig selector,De-biasing,High-dimensional regression,Missing data,Confidence intervals,Minimax rates
AI 理解论文
溯源树
样例
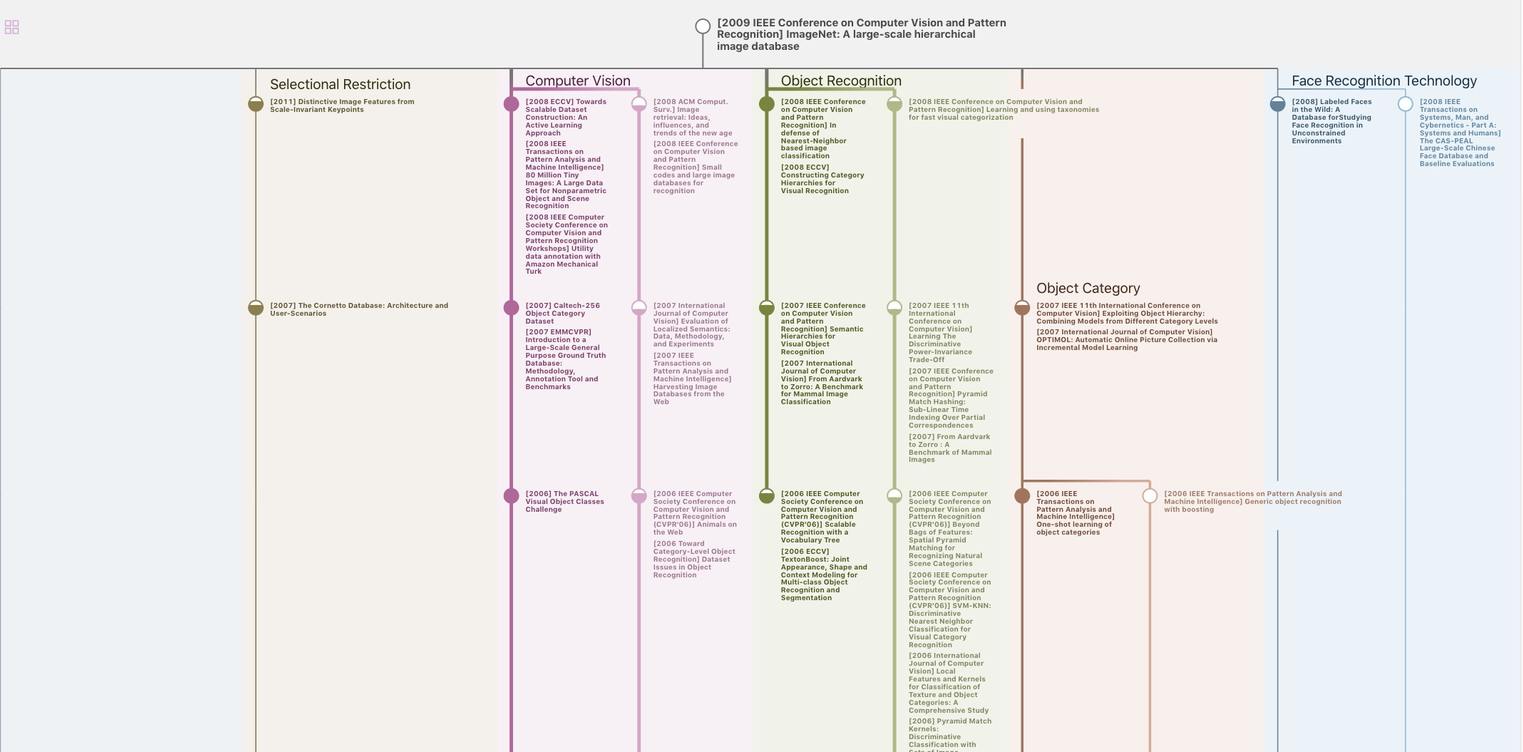
生成溯源树,研究论文发展脉络
Chat Paper
正在生成论文摘要