Collaborative Alerts Ranking for Anomaly Detection.
CIKM(2018)
摘要
Given a large number of low-quality heterogeneous categorical alerts collected from an anomaly detection system, how to characterize the complex relationships between different alerts and deliver trustworthy rankings to end users? While existing techniques focus on either mining alert patterns or filtering out false positive alerts, it can be more advantageous to consider the two perspectives simultaneously in order to improve detection accuracy and better understand abnormal system behaviors. In this paper, we propose CAR, a collaborative alert ranking framework that exploits both temporal and content correlations from heterogeneous categorical alerts. CAR first builds a hierarchical Bayesian model to capture both short-term and long-term dependencies in each alert sequence. Then, an entity embedding-based model is proposed to learn the content correlations between alerts via their heterogeneous categorical attributes. Finally, by incorporating both temporal and content dependencies into a unified optimization framework, CAR ranks both alerts and their corresponding alert patterns. Our experiments-using both synthetic and real-world enterprise security alert data-show that CAR can accurately identify true positive alerts and successfully reconstruct the attack scenarios at the same time.
更多查看译文
关键词
Anomaly detection, Alert ranking, Temporal dependency modeling, Content dependency modeling, Entity embedding, Enterprise security system
AI 理解论文
溯源树
样例
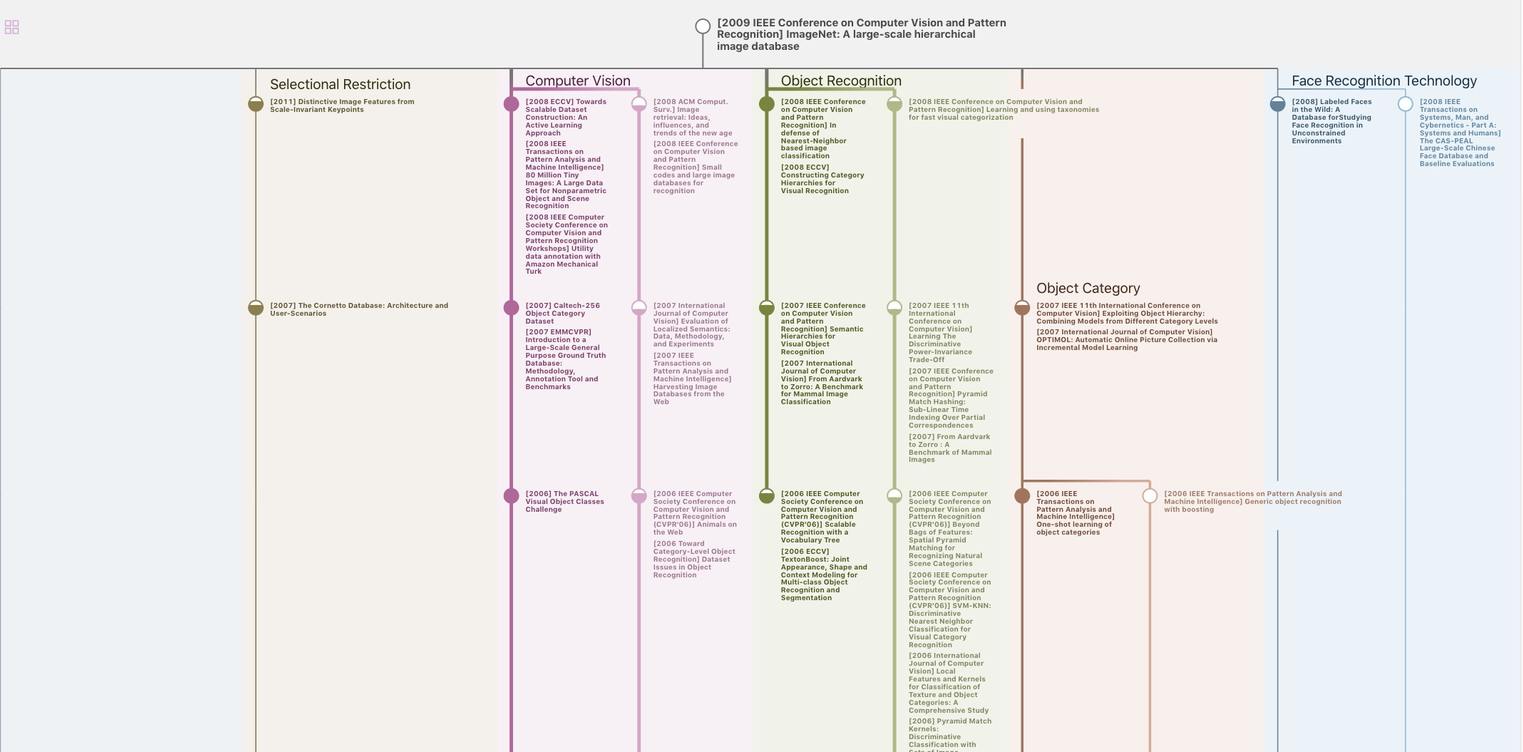
生成溯源树,研究论文发展脉络
Chat Paper
正在生成论文摘要