Adversarial Networks for the Detection of Aggressive Prostate Cancer.
arXiv: Computer Vision and Pattern Recognition(2017)
摘要
Semantic segmentation constitutes an integral part of medical image analyses for which breakthroughs in the field of deep learning were of high relevance. The large number of trainable parameters of deep neural networks however renders them inherently data hungry, a characteristic that heavily challenges the medical imaging community. Though interestingly, with the de facto standard training of fully convolutional networks (FCNs) for semantic segmentation being agnostic towards the `structureu0027 of the predicted label maps, valuable complementary information about the global quality of the segmentation lies idle. In order to tap into this potential, we propose utilizing an adversarial network which discriminates between expert and generated annotations in order to train FCNs for semantic segmentation. Because the adversary constitutes a learned parametrization of what makes a good segmentation at a global level, we hypothesize that the method holds particular advantages for segmentation tasks on complex structured, small datasets. This holds true in our experiments: We learn to segment aggressive prostate cancer utilizing MRI images of 152 patients and show that the proposed scheme is superior over the de facto standard in terms of the detection sensitivity and the dice-score for aggressive prostate cancer. The achieved relative gains are shown to be particularly pronounced in the small dataset limit.
更多查看译文
关键词
adversarial networks,aggressive prostate cancer,prostate cancer
AI 理解论文
溯源树
样例
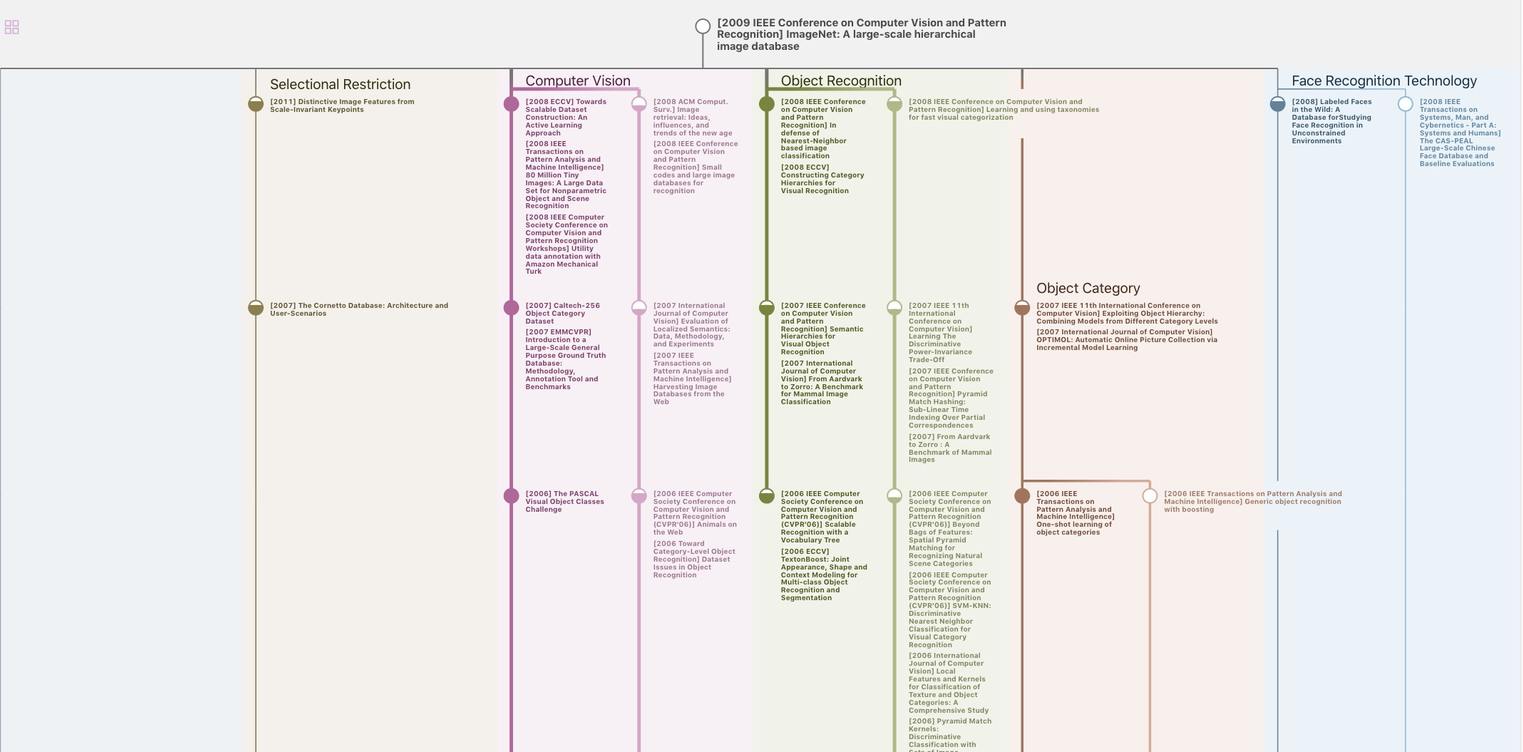
生成溯源树,研究论文发展脉络
Chat Paper
正在生成论文摘要