Butterfly Effect: Peeling Bipartite Networks.
arXiv: Social and Information Networks(2016)
摘要
Affiliation, or two-mode, networks, such as actor-movie, document-keyword, or user-product are prevalent in a lot of applications. The networks can be most naturally modeled as bipartite graphs, but most graph mining algorithms and implementations are designed to work on the classic, unipartite graphs. Subsequently, studies on affiliation networks are conducted on the co-occurrence graphs (e.g., co-authors and co-purchase networks), which projects the bipartite structure to a unipartite structure by connecting two entities if they share an affiliation. Despite their convenience, co-occurrence networks come at a cost of loss of information and an explosion in graph sizes. In this paper, we study the dense subgraph discovery problem on bipartite graphs. We propose peeling algorithms to find many dense substructures and a hierarchy among them. Our peeling algorithms are based on the butterfly subgraphs (2,2-bicliques). Experiments show that we can identify much denser structures compared to the state-of-the-art techniques on co-occurrence graphs. Our algorithms are also memory efficient, since they do not suffer from the explosion in the number of edges of the co-occurrence graph. An in-depth analysis on the author-paper network of the top database conferences highlights the richer structure that can be identified by working on bipartite graphs, which is otherwise lost in a co-occurrence network.
更多查看译文
AI 理解论文
溯源树
样例
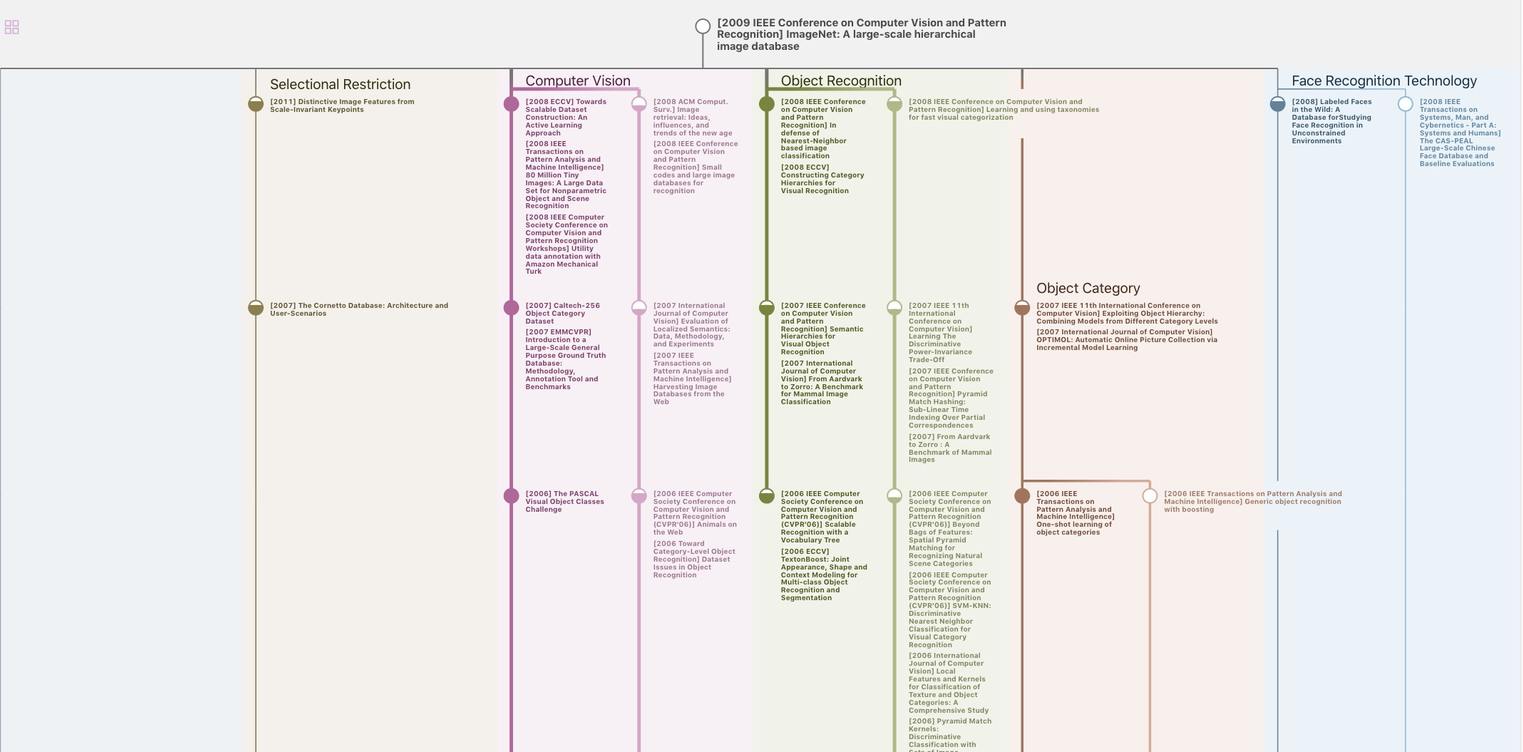
生成溯源树,研究论文发展脉络
Chat Paper
正在生成论文摘要