Deep Reinforcement Learning for Multi-Domain Dialogue Systems
arXiv: Artificial Intelligence(2016)
摘要
Standard deep reinforcement learning methods such as Deep Q-Networks (DQN) for multiple tasks (domains) face scalability problems. We propose a method for multi-domain dialogue policy learning—termed NDQN, and apply it to an information-seeking spoken dialogue system in the domains of restaurants and hotels. Experimental results comparing DQN (baseline) versus NDQN (proposed) using simulations report that our proposed method exhibits better scalability and is promising for optimising the behaviour of multi-domain dialogue systems.
更多查看译文
关键词
deep reinforcement learning,multidomain dialogue systems,deep Q-networks,scalability problems,search spaces,three-stage method,multidomain dialogue policy learning,NDQN method,information seeking spoken dialogue system,multipolicy learning,DQN agents network,state representations,agent behaviour
AI 理解论文
溯源树
样例
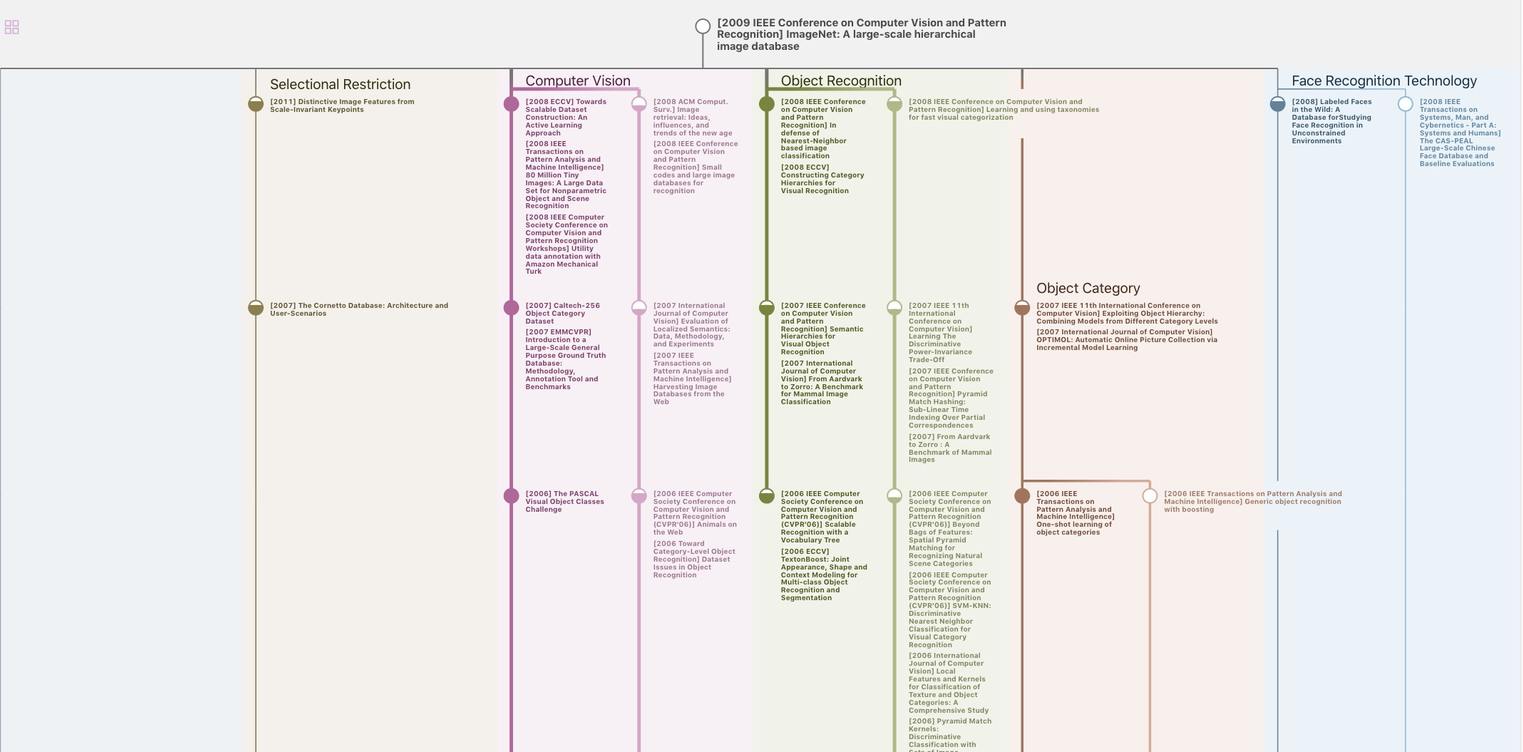
生成溯源树,研究论文发展脉络
Chat Paper
正在生成论文摘要