Distributed Gaussian Learning over Time-varying Directed Graphs
2016 50th Asilomar Conference on Signals, Systems and Computers(2016)
摘要
We present a distributed (non-Bayesian) learning algorithm for the problem of parameter estimation with Gaussian noise. The algorithm is expressed as explicit updates on the parameters of the Gaussian beliefs (i.e. means and precision). We show a convergence rate of $O(1/k)$ with the constant term depending on the number of agents and the topology of the network. Moreover, we show almost sure convergence to the optimal solution of the estimation problem for the general case of time-varying directed graphs.
更多查看译文
关键词
distributed Gaussian learning,nonBayesian learning algorithm,parameter estimation,Gaussian noise,Gaussian beliefs,network topology,time-varying directed graphs
AI 理解论文
溯源树
样例
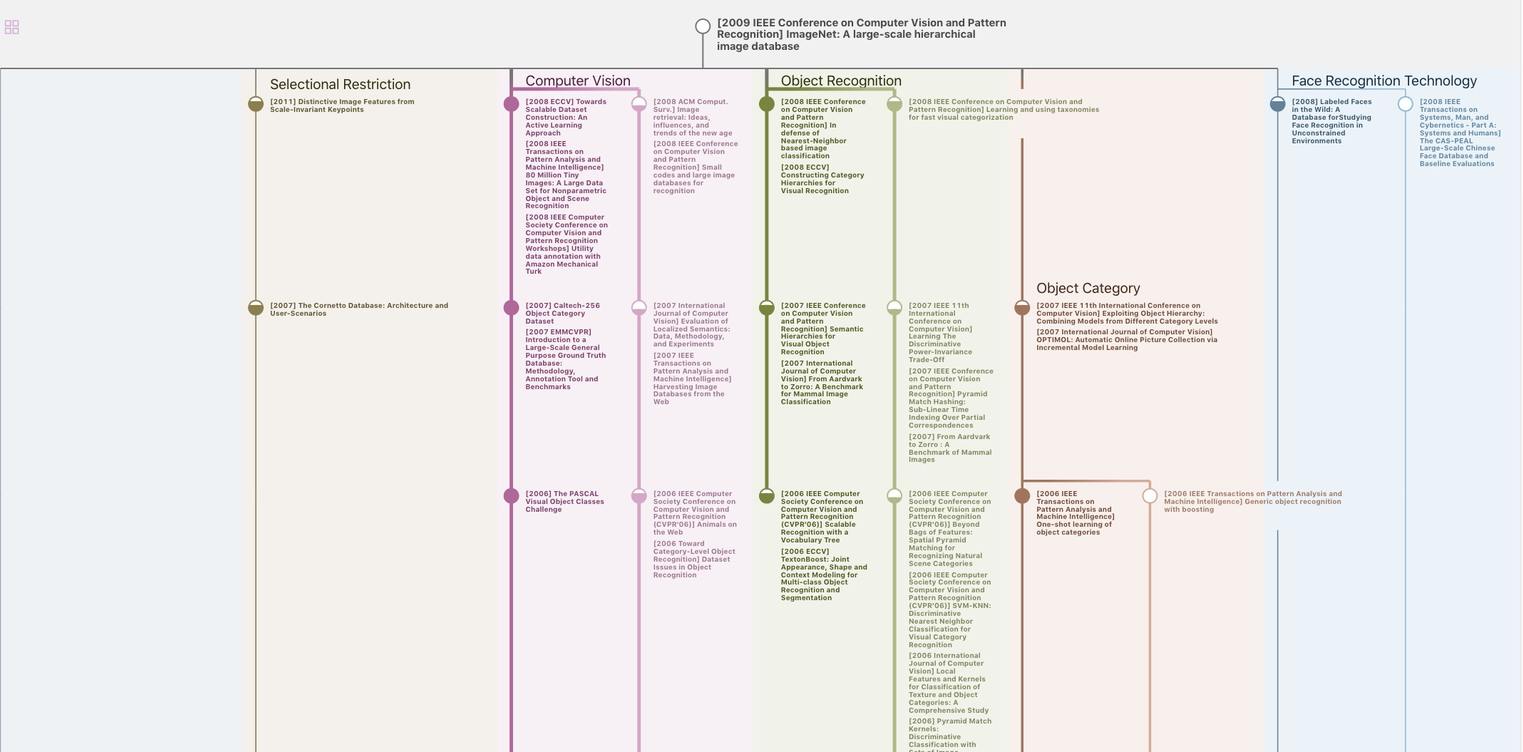
生成溯源树,研究论文发展脉络
Chat Paper
正在生成论文摘要