Evolutionary Multiobjective Optimization for Adaptive Dataflow-based Digital Predistortion Architectures.
EAI Endorsed Trans. Cognitive Communications(2017)
摘要
In wireless communication systems, high-power transmitters suffer from nonlinearities due to power amplifier (PA) characteristics, I/Q imbalance, and local oscillator (LO) leakage. Digital Predistortion (DPD) is an effective technique to counteract these impairments. To help maximize agility in cognitive radio systems, it is important to investigate dynamically reconfigurable DPD systems that are adaptive to changes in the employed modulation schemes and operational constraints. To help maximize effectiveness, such reconfiguration should be performed based on multidimensional operational criteria. With this motivation, we develop in this paper a novel evolutionary algorithm framework for multiobjective optimization of DPD systems. We demonstrate our framework by applying it to develop an adaptive DPD architecture, called the adaptive, dataflow-based DPD architecture (ADDA), where Pareto-optimized DPD parameters are derived subject to multidimensional constraints to support efficient predistortion across time-varying operational requirements and modulation schemes. Through extensive simulation results, we demonstrate the effectiveness of our proposed multiobjective optimization framework in deriving efficient DPD configurations for run-time adaptation.
更多查看译文
关键词
Digital predistortion,multiobjective optimization,evolutionary algorithms
AI 理解论文
溯源树
样例
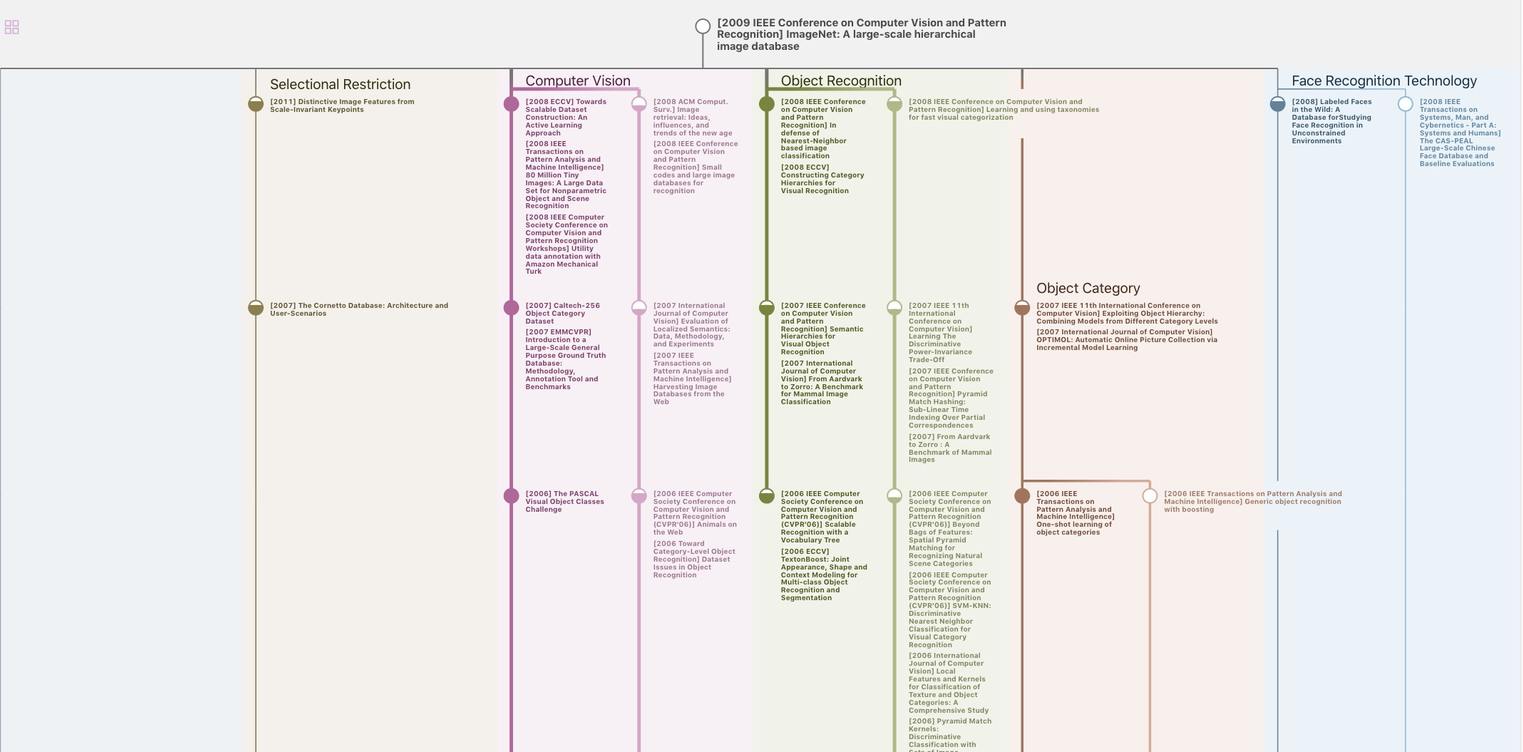
生成溯源树,研究论文发展脉络
Chat Paper
正在生成论文摘要