Matrix-Based Dynamic Updating Rough Fuzzy Approximations For Data Mining
Knowledge Based Systems(2017)
摘要
In a dynamic environment, the data collected from real applications varies not only with the amount of objects but also with the number of features, which will result in continuous change of knowledge over time. The static methods of updating knowledge need to recompute from scratch when new data are added every time. This makes it potentially very time-consuming to update knowledge, especially as the dataset grows dramatically. Calculation of approximations is one of main mining tasks in rough set theory, like frequent pattern mining in association rules. Considering the fuzzy descriptions of decision states in the universe under fuzzy environment, this paper aims to provide an efficient approach for computing rough approximations of fuzzy concepts in dynamic fuzzy decision systems (FDS) with simultaneous variation of objects and features. We firstly present a matrix-based representation of rough fuzzy approximations by a Boolean matrix associated with a matrix operator in FDS. While adding the objects and features concurrently, incremental mechanisms for updating rough fuzzy approximations are introduced, and the corresponding matrix-based dynamic algorithm is developed. Unlike the static method of computing approximations by updating the whole relation matrix, our new approach partitions it into sub-matrices and updates each sub-matrix locally by utilizing the previous matrix information and the interactive information of each sub-matrix to avoid unnecessary calculations. Experimental results on six UCI datasets shown that the proposed dynamic algorithm achieves significantly higher efficiency than the static algorithm and the combination of two reference incremental algorithms. (C) 2016 Elsevier B.V. All rights reserved.
更多查看译文
关键词
Rough fuzzy set,Incremental learning,Matrix,Rough approximations
AI 理解论文
溯源树
样例
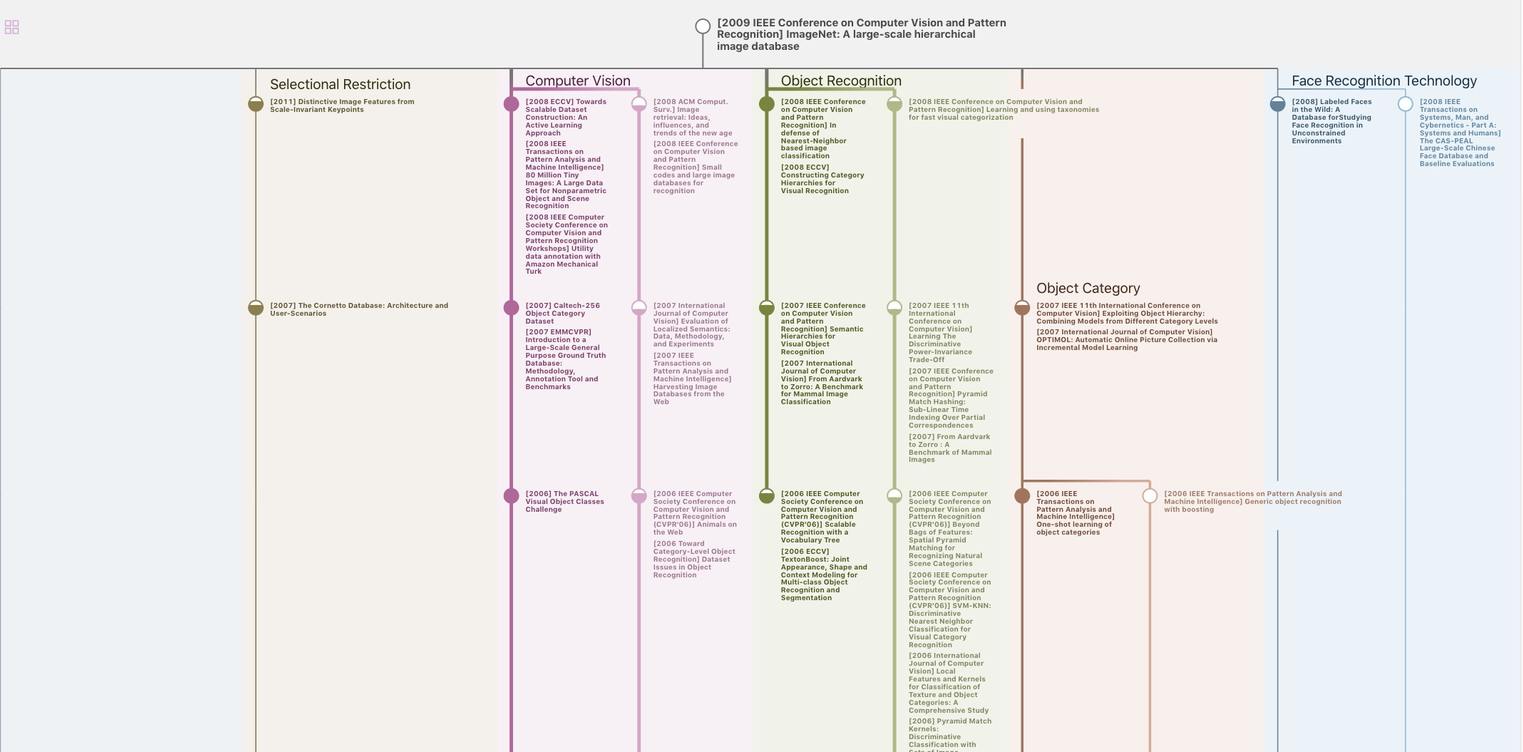
生成溯源树,研究论文发展脉络
Chat Paper
正在生成论文摘要