Web Content Classification Using Distributions Of Subjective Quality Evaluations
ACM Transactions on The Web(2016)
摘要
Machine learning algorithms and recommender systems trained on human ratings are widely in use today. However, human ratings may be associated with a high level of uncertainty and are subjective, influenced by demographic or psychological factors. We propose a new approach to the design of object classes from human ratings: the use of entire distributions to construct classes. By avoiding aggregation for class definition, our approach loses no information and can deal with highly volatile or conflicting ratings. The approach is based the concept of the Earth Mover's Distance (EMD), a measure of distance for distributions. We evaluate the proposed approach based on four datasets obtained from diverse Web content or movie quality evaluation services or experiments. We show that clusters discovered in these datasets using the EMD measure are characterized by a consistent and simple interpretation. Quality classes defined using entire rating distributions can be fitted to clusters of distributions in the four datasets using two parameters, resulting in a good overall fit. We also consider the impact of the composition of small samples on the distributions that are the basis of our classification approach. We show that using distributions based on small samples of 10 evaluations is still robust to several demographic and psychological variables. This observation suggests that the proposed approach can be used in practice for quality evaluation, even for highly uncertain and subjective ratings.
更多查看译文
关键词
Web content quality,rating distribution,earth mover's distance,classification design,sample composition,robustness
AI 理解论文
溯源树
样例
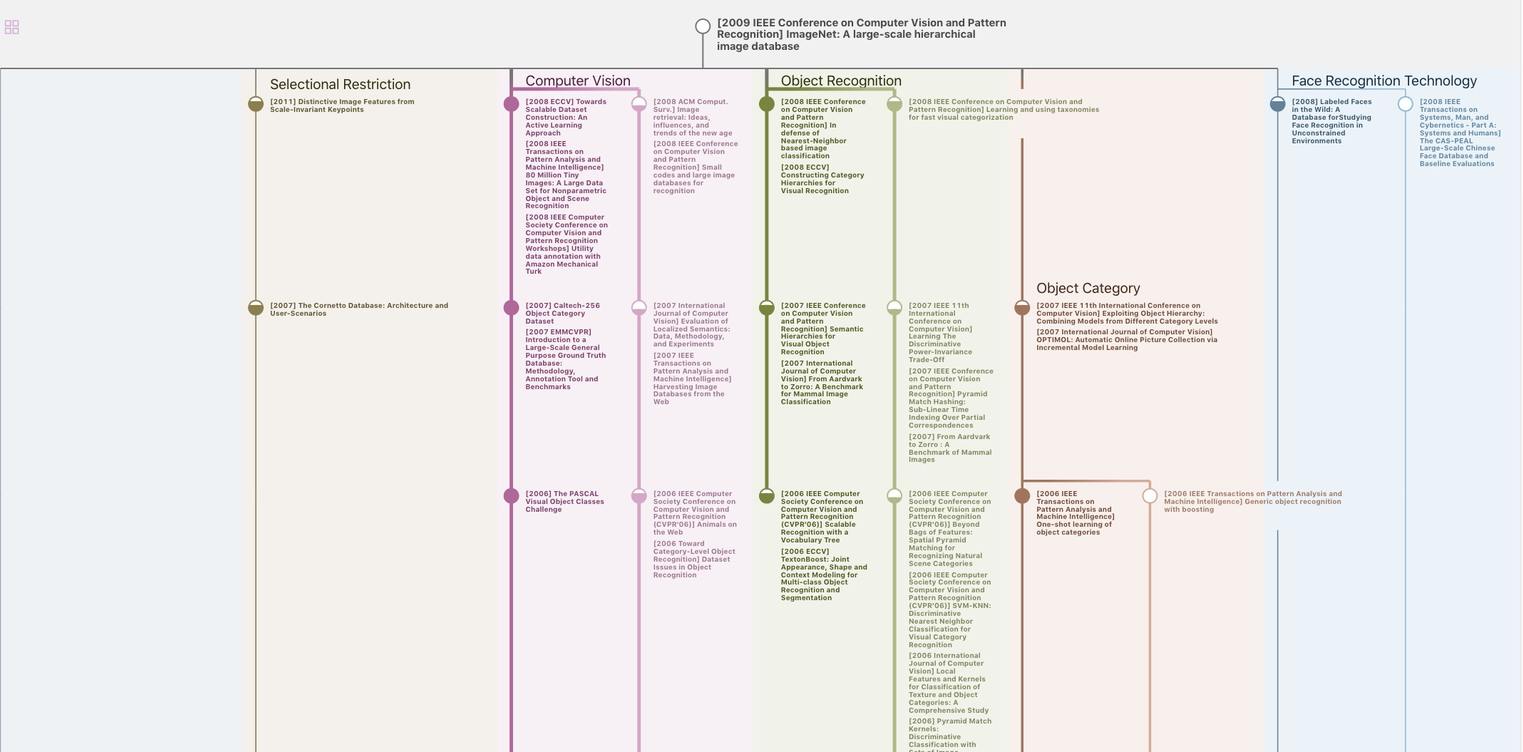
生成溯源树,研究论文发展脉络
Chat Paper
正在生成论文摘要