Towards Modeling False Memory With Computational Knowledge Bases.
TOPICS IN COGNITIVE SCIENCE(2017)
摘要
One challenge to creating realistic cognitive models of memory is the inability to account for the vast common-sense knowledge of human participants. Large computational knowledge bases such as WordNet and DBpedia may offer a solution to this problem but may pose other challenges. This paper explores some of these difficulties through a semantic network spreading activation model of the Deese-Roediger-McDermott false memory task. In three experiments, we show that these knowledge bases only capture a subset of human associations, while irrelevant information introduces noise and makes efficient modeling difficult. We conclude that the contents of these knowledge bases must be augmented and, more important, that the algorithms must be refined and optimized, before large knowledge bases can be widely used for cognitive modeling.
更多查看译文
关键词
False memory,Spreading activation,WordNet,DBpedia,Knowledge base,Cognitive architecture
AI 理解论文
溯源树
样例
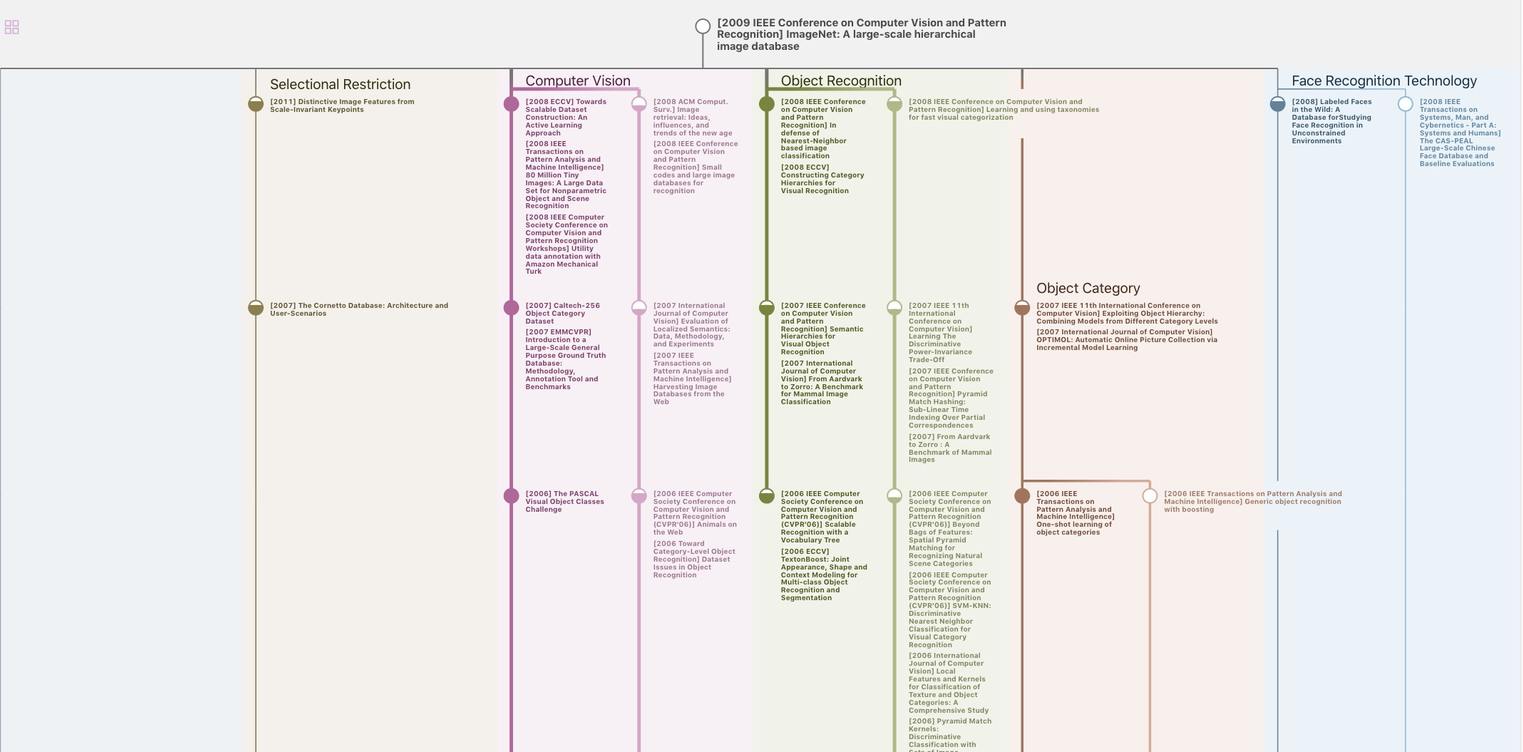
生成溯源树,研究论文发展脉络
Chat Paper
正在生成论文摘要