Two-Stage Focused Inference for Resource-Constrained Minimal Collision Navigation.
IEEE Trans. Robotics(2017)
摘要
The operation of mobile robots in unknown environments typically requires building maps during exploration. As the exploration time and environment size increase, the amount of data collected and the number of variables required to represent these maps both grow, which is problematic since all real robots have finite resources. The solution proposed in this paper is to only retain the variables and measurements that are most important to achieve the robot's task. The variable and measurement selection approach is demonstrated on the task of navigation with a low risk of collision. Our approach has two stages: first, a subset of the variables is selected that is most useful for minimizing the uncertainty of navigation (termed the “focused variables”). And second, a task-agnostic method is used to select a subset of the measurements that maximizes the information over these focused variables (“focused inference”). Detailed simulations and hardware experiments show that the two-stage approach constrains the number of variables and measurements. It can generate much sparser maps than existing approaches in the literature, while still achieving a better task performance—in this case (fewer collisions). An incremental and iterative approach is further presented, in which the two-stage procedure is performed on subsets of the data, and thus, avoids the necessity of performing a resource-intensive batch selection on large datasets.
更多查看译文
关键词
Navigation,Collision avoidance,Uncertainty,Simultaneous localization and mapping,Roads,Mobile robots
AI 理解论文
溯源树
样例
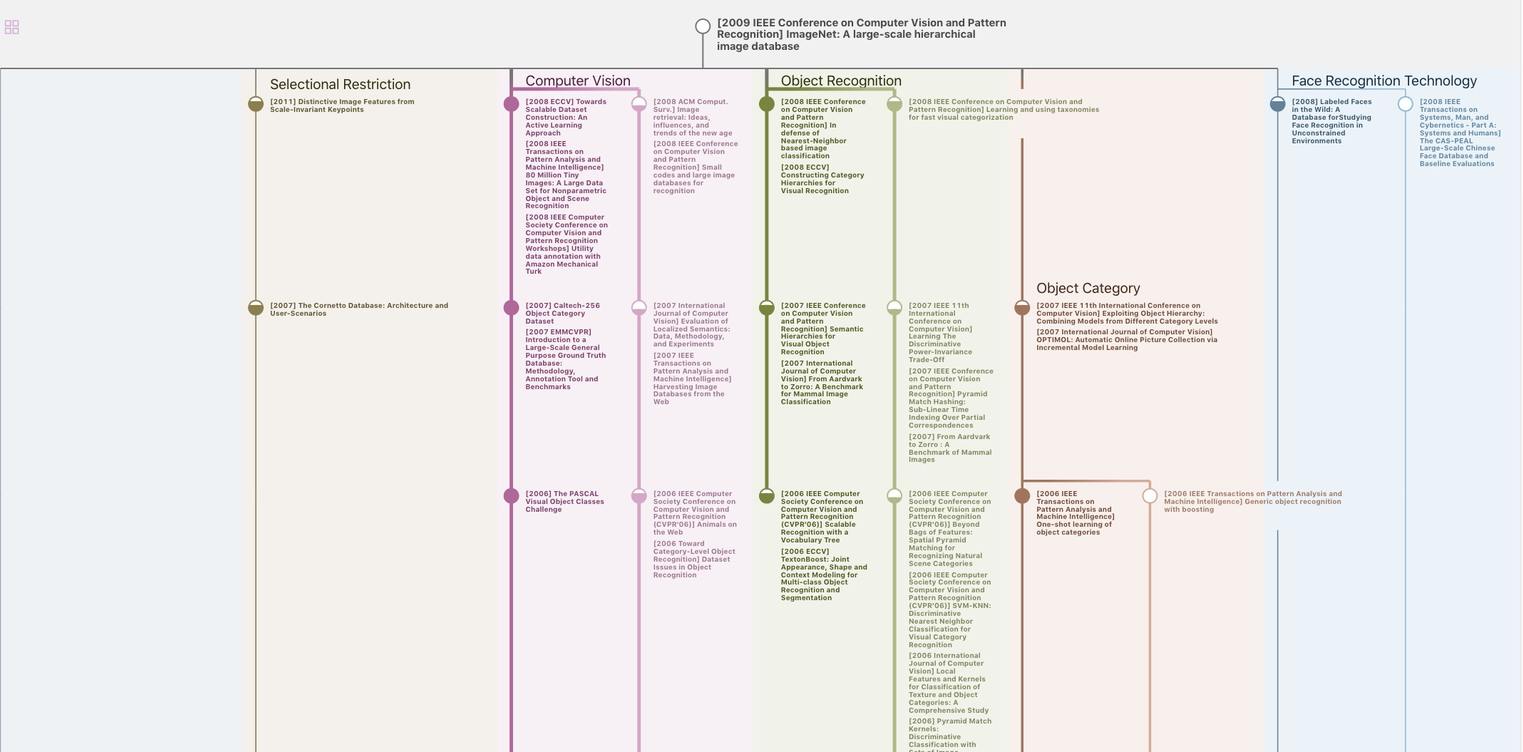
生成溯源树,研究论文发展脉络
Chat Paper
正在生成论文摘要