Novel iterative approach using generative and discriminative models for classification with missing features.
Neurocomputing(2017)
摘要
Missing feature is a common problem in real-world data classification. Therefore, a robust classification method is required when classifying data with missing features. In this study, we propose an iterative algorithm composed of a generative model that works in conjunction with a discriminative model in a cycle. The Gaussian mixture model (GMM) and the multilayer perceptron (MLP) (or the support vector machine (SVM)) present the generative and discriminative parts of the proposed algorithm, respectively. This study conducted two experiments using UC Irvine datasets. One is to show the superiority of the proposed method through its higher classification accuracy compared with previous classification methods including with respect to marginalization, mean imputation, conditional mean imputation, and zero-mean imputation. The other is to compare classification accuracy of the proposed method with that of conventional the state-of-the-art GMM-based approaches to the missing data problem.
更多查看译文
关键词
Missing data imputation,Generative and discriminative model
AI 理解论文
溯源树
样例
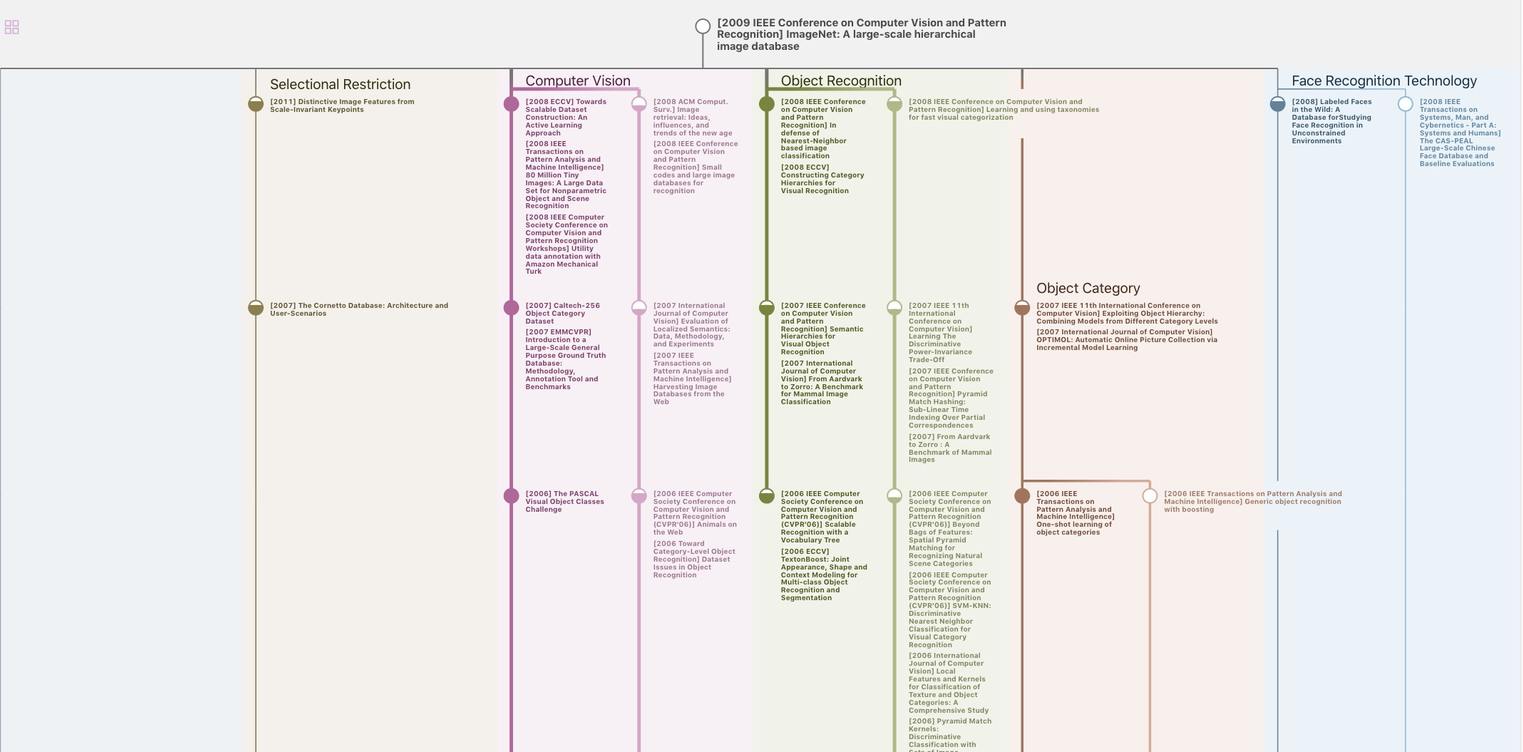
生成溯源树,研究论文发展脉络
Chat Paper
正在生成论文摘要