An improved multiple birth support vector machine for pattern classification.
Neurocomputing(2017)
摘要
Multiple birth support vector machine is a novel machine learning algorithm for multi-class classification, which is considered as an extension of twin support vector machine. Compared with training speeds of other multi-class classifiers based on twin support vector machine, the training speed of multiple birth support vector machine is faster, especially when the number of class is large. However, one of the disadvantages of multiple birth support vector machine is that when used to deal with some datasets such as \"Cross planes\" datasets, multiple birth support vector machine is likely to get bad results. In order to deal with this, we propose an improved multiple birth support vector machine. We add a modified item into multiple birth support vector machine to make the variance of the distances from each samples of a given class to their hyperplanes as small as possible. To predict a new sample, our method first determines an interval for each class depending on the distances between training samples and their hyperplanes, and then classifies the new sample depending on the distances between hyperplanes and the new sample which are in the corresponding intervals. In addition, smoothing technique is applied on our model, the first time it was used in multi-class twin support vector machine. The experimental results on artificial datasets and UCI datasets show that the proposed algorithm is efficient and has good classification performance.
更多查看译文
关键词
Support vector machine,Twin support vector machine,Multiple birth support vector machine,Multi-class classification,Smoothing technique
AI 理解论文
溯源树
样例
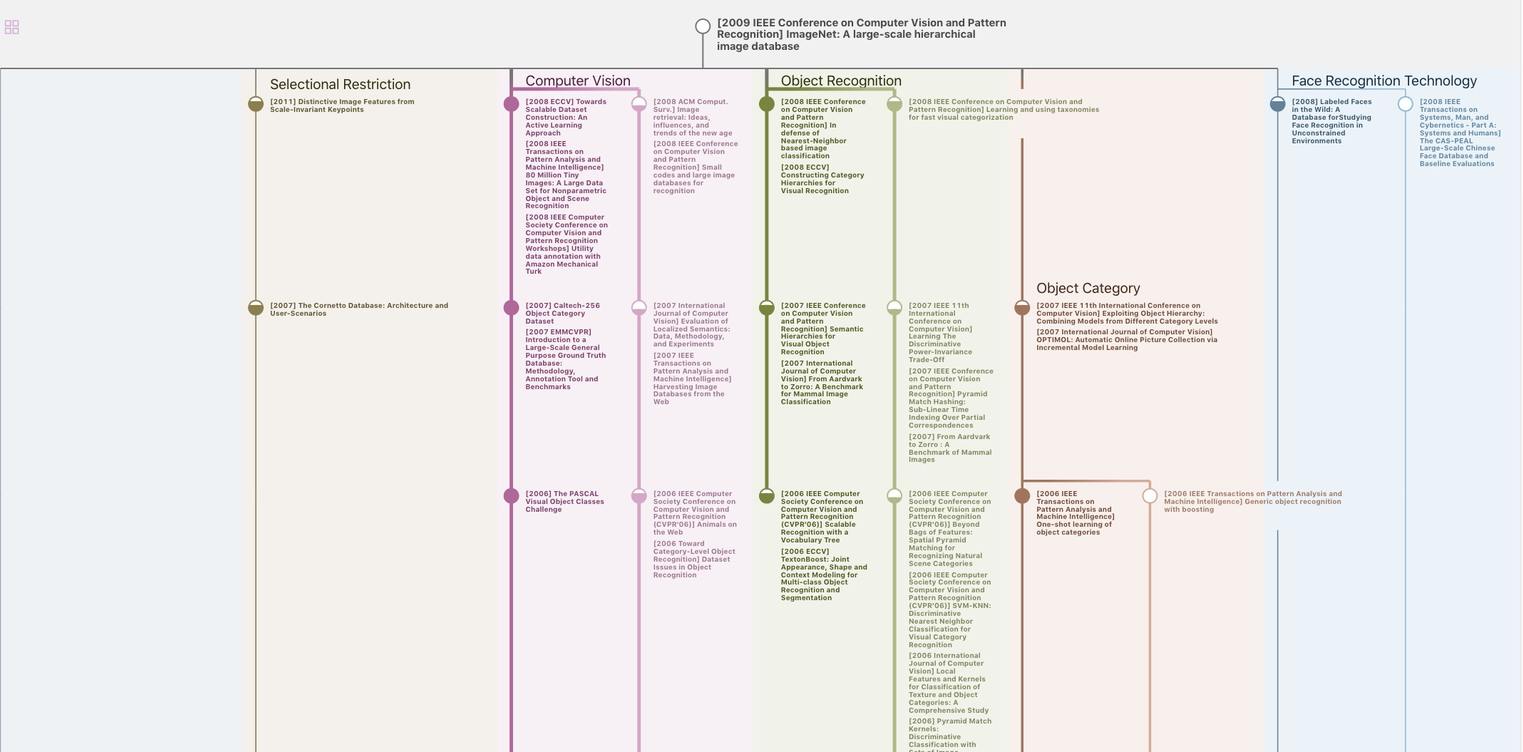
生成溯源树,研究论文发展脉络
Chat Paper
正在生成论文摘要