An improved extremal optimization based on the distribution knowledge of candidate solutions
Natural Computing(2016)
摘要
Extremal optimization (EO) is a phenomenon-mimicking algorithm inspired by the Bak-Sneppen model of self-organized criticality from the field of statistical physics. The canonical EO works on a single solution and only employs mutation operator, which is inclined to prematurely converge to local optima. In this paper, a population-based extremal optimization algorithm is developed to provide a parallel way for exploring the search space. In addition, a new mutation strategy named cloud mutation is proposed by analyzing the distribution knowledge of each component set in the solution set. The population-based extremal optimization with cloud mutation is characteristic of mining and recreating the uncertainty properties of candidate solutions in the search process. Finally, the proposed algorithm is applied to numerical optimization problems in comparison with other reported meta-heuristic algorithms. The statistical results show that the proposed algorithm can achieve a satisfactory optimization performance with regards to solution quality, successful rate, convergence speed, and computing robustness.
更多查看译文
关键词
Extremal optimization,Distribution knowledge,Cloud mutation,Numerical optimization
AI 理解论文
溯源树
样例
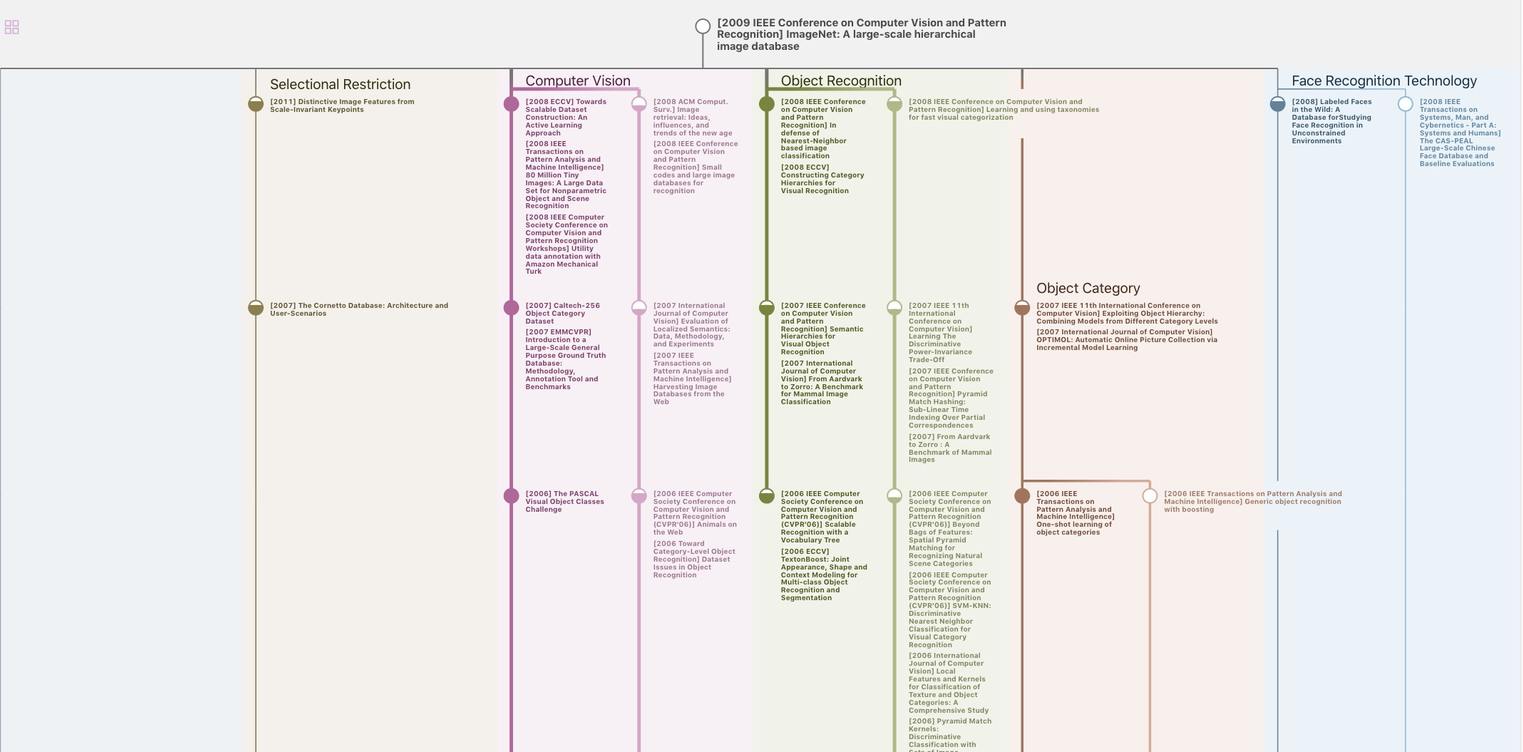
生成溯源树,研究论文发展脉络
Chat Paper
正在生成论文摘要