Testing multiple hypotheses through IMP weighted FDR based on a genetic functional network with application to a new zebrafish transcriptome study
BioData Mining(2015)
摘要
In genome-wide studies, hundreds of thousands of hypothesis tests are performed simultaneously. Bonferroni correction and False Discovery Rate (FDR) can effectively control type I error but often yield a high false negative rate. We aim to develop a more powerful method to detect differentially expressed genes. We present a Weighted False Discovery Rate (WFDR) method that incorporate biological knowledge from genetic networks. We first identify weights using Integrative Multi-species Prediction (IMP) and then apply the weights in WFDR to identify differentially expressed genes through an IMP-WFDR algorithm. We performed a gene expression experiment to identify zebrafish genes that change expression in the presence of arsenic during a systemic Pseudomonas aeruginosa infection. Zebrafish were exposed to arsenic at 10 parts per billion and/or infected with P. aeruginosa . Appropriate controls were included. We then applied IMP-WFDR during the analysis of differentially expressed genes. We compared the mRNA expression for each group and found over 200 differentially expressed genes and several enriched pathways including defense response pathways, arsenic response pathways, and the Notch signaling pathway.
更多查看译文
关键词
False discovery rate,Family-wise error rate,Genomic studies,Data integration
AI 理解论文
溯源树
样例
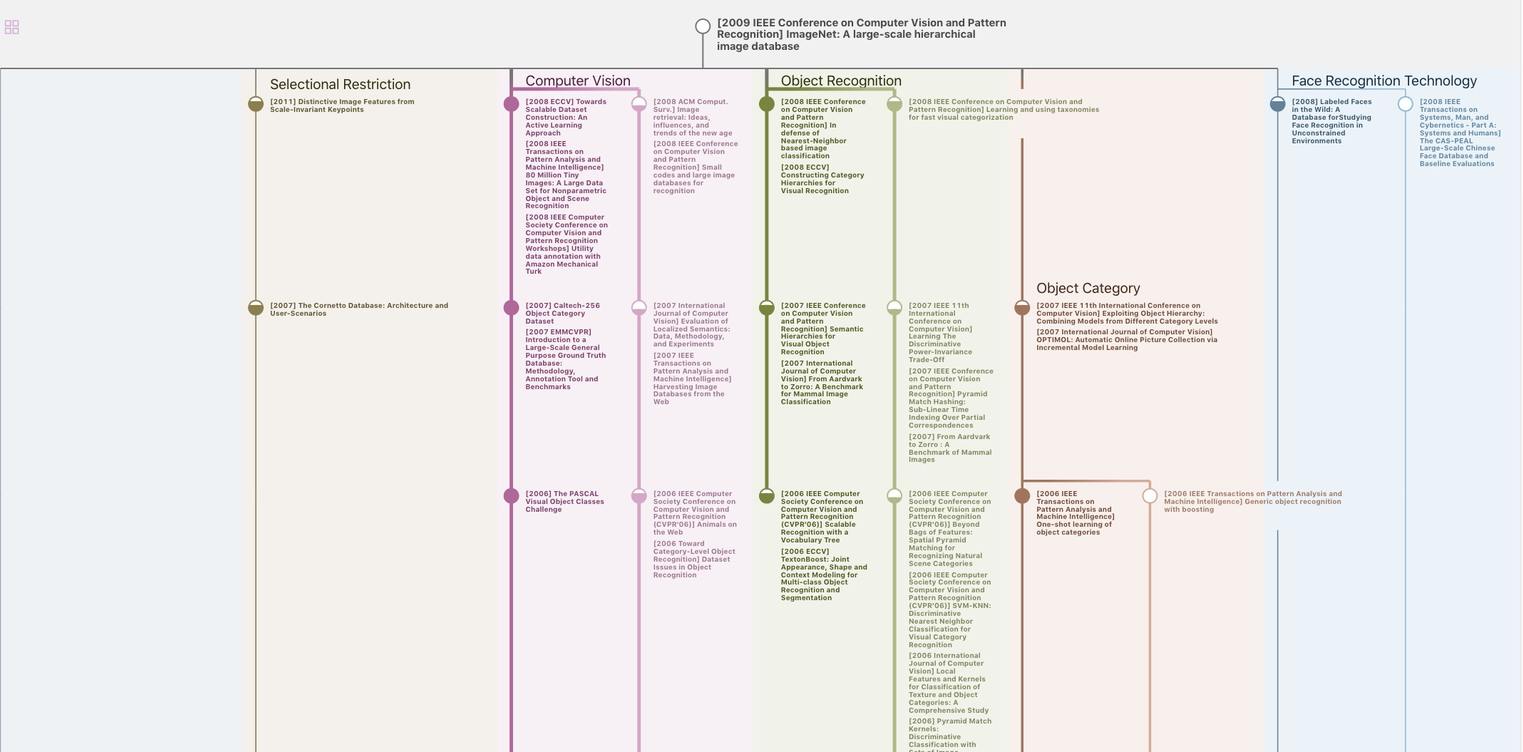
生成溯源树,研究论文发展脉络
Chat Paper
正在生成论文摘要