Handling non-linearity in radar data assimilation using the non-linear least squares enhanced POD-4DVar
Science China Earth Sciences(2017)
摘要
The Proper Orthogonal Decomposition (POD)-based ensemble four-dimensional variational (4DVar) assimilation method (POD4DEnVar) was proposed to combine the strengths of EnKF (i.e., the ensemble Kalman filter) and 4DVar assimilation methods. Recently, a POD4DEnVar-based radar data assimilation scheme (PRAS) was built and its effectiveness was demonstrated. POD4DEnVar is based on the assumption of a linear relationship between the model perturbations (MPs) and the observation perturbations (OPs); however, this assumption is likely to be destroyed by the highly non-linear forecast model or observation operator. To address this issue, using the Gauss-Newton iterative method, the nonlinear least squares enhanced POD4DEnVar algorithm (referred to as NLS-4DVar) was proposed. Naturally, the PRAS was upgraded to form the NLS-4DVar-based radar data assimilation scheme (NRAS). To evaluate the performance of NRAS against PRAS, observing system simulation experiments (OSSEs) were conducted to assimilate reflectivity and radial velocity individually, with one, two, and three iterations. The results demonstrated that the NRAS outperformed PRAS in improving the initial condition and the forecasting of model variables and rainfall. The NRAS, with a smaller number of iterations, can yield a convergent result. In contrast to the situation when assimilating radial velocity, the advantages of NRAS over PRAS were more obvious when assimilating reflectivity.
更多查看译文
关键词
Data assimilation,Non-linearity,Gauss-Newton,NRAS,PRAS,Radar reflectivity,Radial velocity
AI 理解论文
溯源树
样例
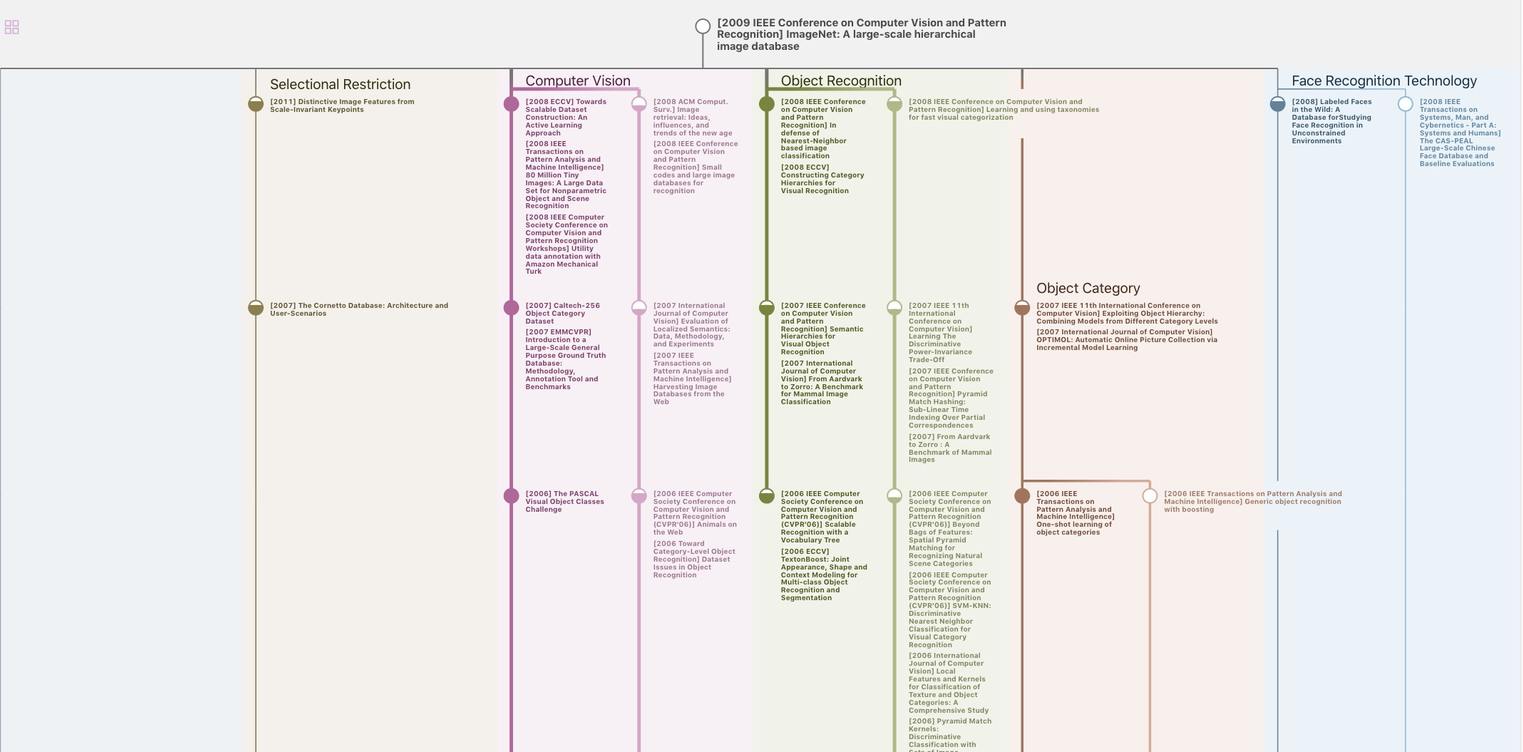
生成溯源树,研究论文发展脉络
Chat Paper
正在生成论文摘要