Self-Calibrating Protocols as diagnostic aids for personal medicine, neurological conditions and pain assessment
PETRA(2016)
摘要
Recent advances in the tracking and quantification of pain using consumer-grade wearable EEG headbands, such as Muse [8] and Neurosky [12], coupled to efficient machine learning [9], pave the way towards applying Self-Calibrating Protocols (SCP) [10] and Dynamic Background Reduction (DBR) [11] principles to basic research, while empowering new applications. In the cases of neurological conditions and chronic pain management, SCP is of particular interest during the early diagnostic process as well as an aid in personalizing intervention strategies. In this paper, we outline a framework based on SCP, to design machine learning systems that completely bypass the pitfalls of using normed neurophysiological states for diagnostics. This effort targets short-term practical development of personalized early diagnostics and treatment strategies and has longer-term implications for Brain-Computer Interface (BCI) and Human-Computer Interaction (HCI) methodologies.
更多查看译文
AI 理解论文
溯源树
样例
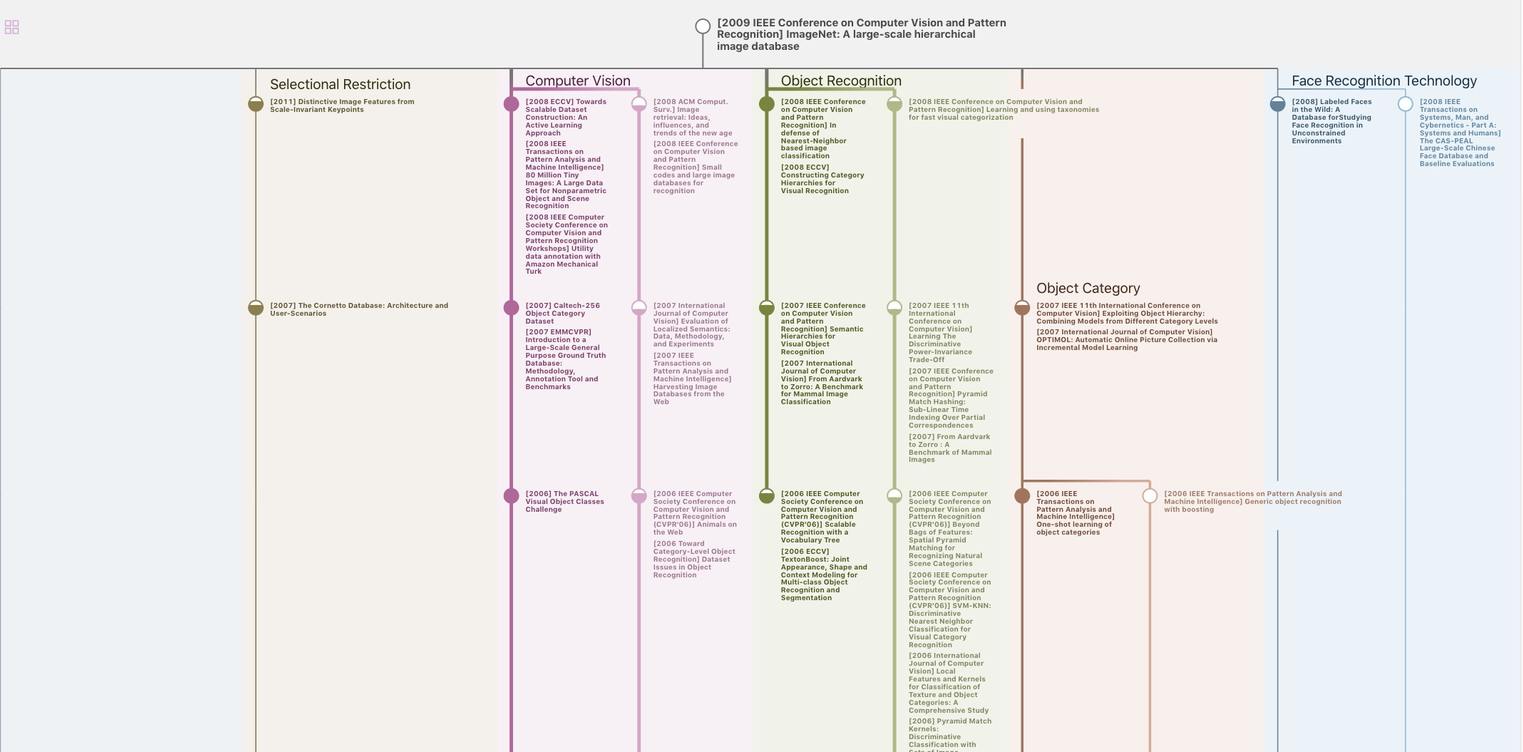
生成溯源树,研究论文发展脉络
Chat Paper
正在生成论文摘要