Integration and Quality Assessment of Heterogeneous Chord Sequences Using Data Fusion.
ISMIR(2016)
摘要
Two heads are better than one, and the many are smarter than the few. Integrating knowledge from multiple sources has shown to increase retrieval and classification accuracy in many domains. The recent explosion of crowd-sourced information, such as on websites hosting chords and tabs for popular songs, calls for sophisticated algorithms for data-driven quality assessment and data integration to create better, and more reliable data. In this paper, we propose to integrate the heterogeneous output of multiple automatic chord extraction algorithms using data fusion. First we show that data fusion creates significantly better chord label sequences from multiple sources, outperforming its source material, majority voting and random source integration. Second, we show that data fusion is capable of assessing the quality of sources with high precision from source agreement, without any ground-truth knowledge. Our study contributes to a growing body of work showing the benefits of integrating knowledge from multiple sources in an advanced way.
更多查看译文
关键词
heterogeneous chord sequences,quality assessment
AI 理解论文
溯源树
样例
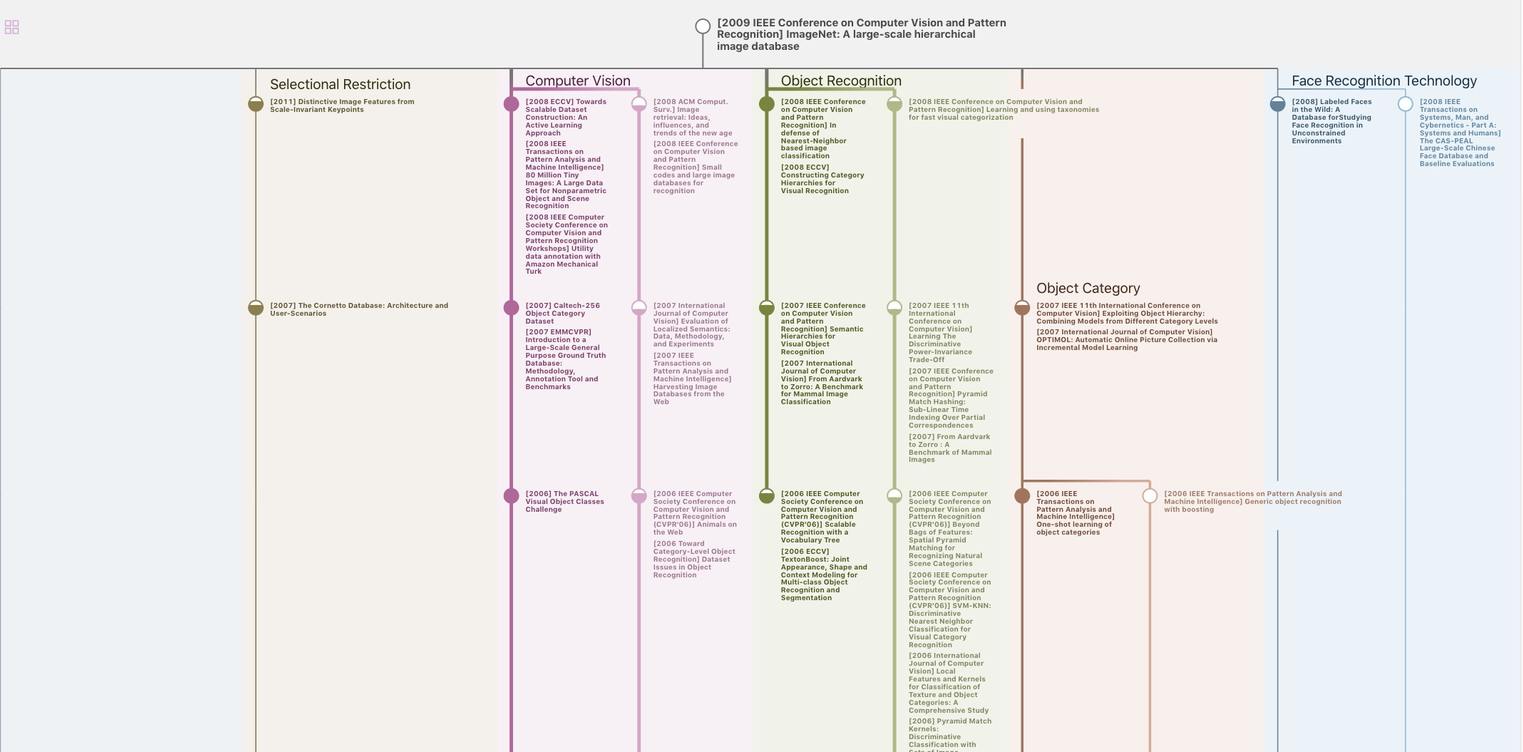
生成溯源树,研究论文发展脉络
Chat Paper
正在生成论文摘要