k-EVCLUS: Clustering Large Dissimilarity Data in the Belief Function Framework.
Lecture Notes in Artificial Intelligence(2016)
摘要
In evidential clustering, the membership of objects to clusters is considered to be uncertain and is represented by mass functions, forming a credal partition. The EVCLUS algorithm constructs a credal partition in such a way that larger dissimilarities between objects correspond to higher degrees of conflict between the associated mass functions. In this paper, we propose to replace the gradient-based optimization procedure in the original EVCLUS algorithm by a much faster iterative row-wise quadratic programming method. We also show that EVCLUS can be provided with only a random sample of the dissimilarities, reducing the time and space complexity from quadratic to linear. These improvements make EVCLUS suitable to cluster large dissimilarity datasets.
更多查看译文
关键词
Evidential clustering,Dempster-Shafer theory,Evidencen theory,Unsupervised learning
AI 理解论文
溯源树
样例
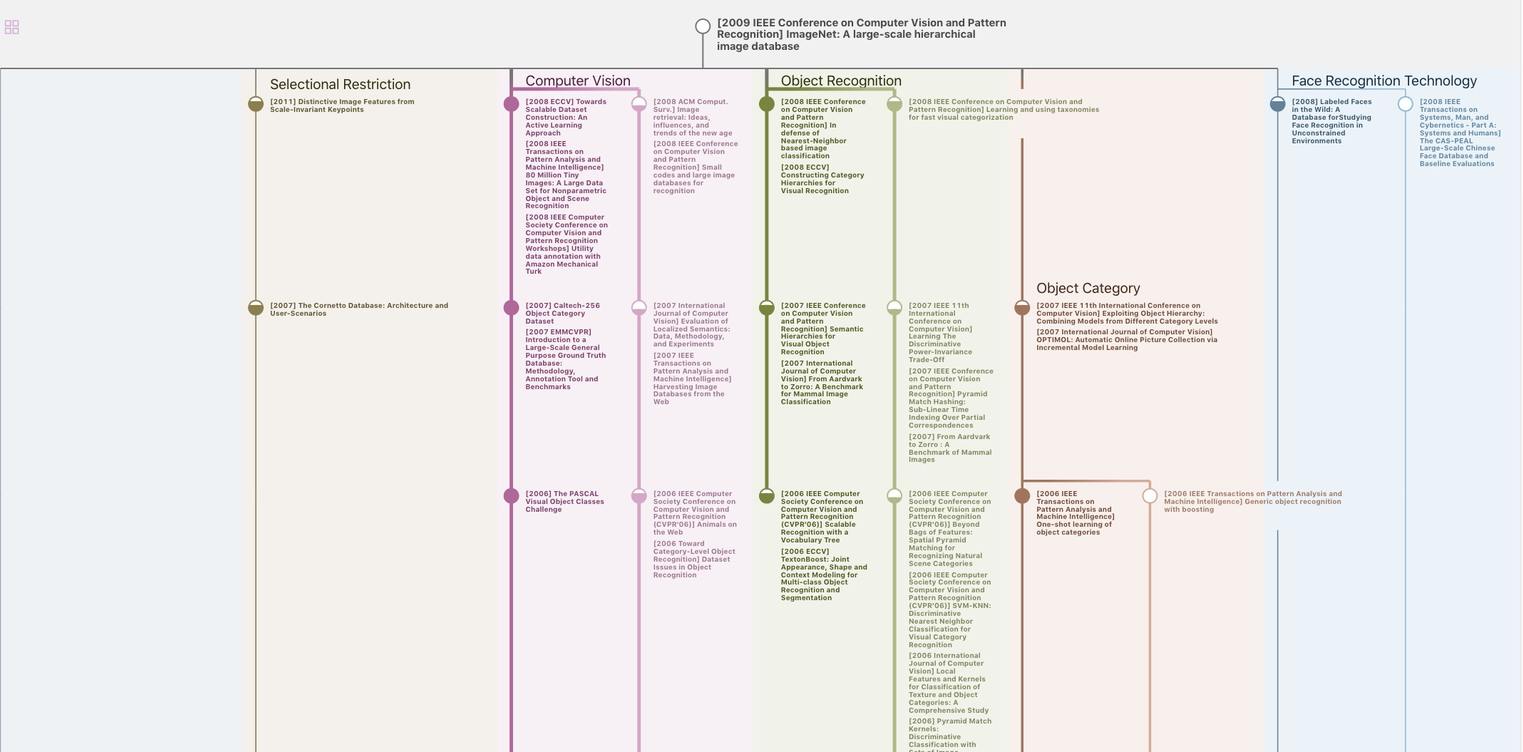
生成溯源树,研究论文发展脉络
Chat Paper
正在生成论文摘要