P-Spectral Clustering Based On Neighborhood Attribute Granulation
INTELLIGENT INFORMATION PROCESSING VIII(2016)
摘要
Clustering analysis is an important method for data mining and information statistics. Data clustering is to find the intrinsic links between objects and describe the internal structures of data sets. p-Spectral clustering is based on Cheeger cut criterion. It has good performance on many challenging data sets. But the original p-spectral clustering algorithm is not suitable for high-dimensional data. To solve this problem, this paper improves p-spectral clustering using neighborhood attribute granulation and proposes NAG-pSC algorithm. Neighborhood rough sets can directly process the continuous data. We introduce information entropy into the neighborhood rough sets to weaken the negative impact of noise data and redundant attributes on clustering. In this way, the data points within the same cluster are more compact, while the data points between different clusters are more separate. The effectiveness of the proposed NAG-pSC algorithm is tested on several benchmark data sets. Experiments show that the neighborhood attribute granulation will highlight the differences between data points while maintaining their characteristics in the clustering. With the help of neighborhood attribute granulation, NAG-pSC is able to recognize more complex data structures and has strong robustness to the noise or irrelevant features in high-dimensional data.
更多查看译文
关键词
p-Spectral clustering,Rough set,Attribute reduction,Neighborhood granulation
AI 理解论文
溯源树
样例
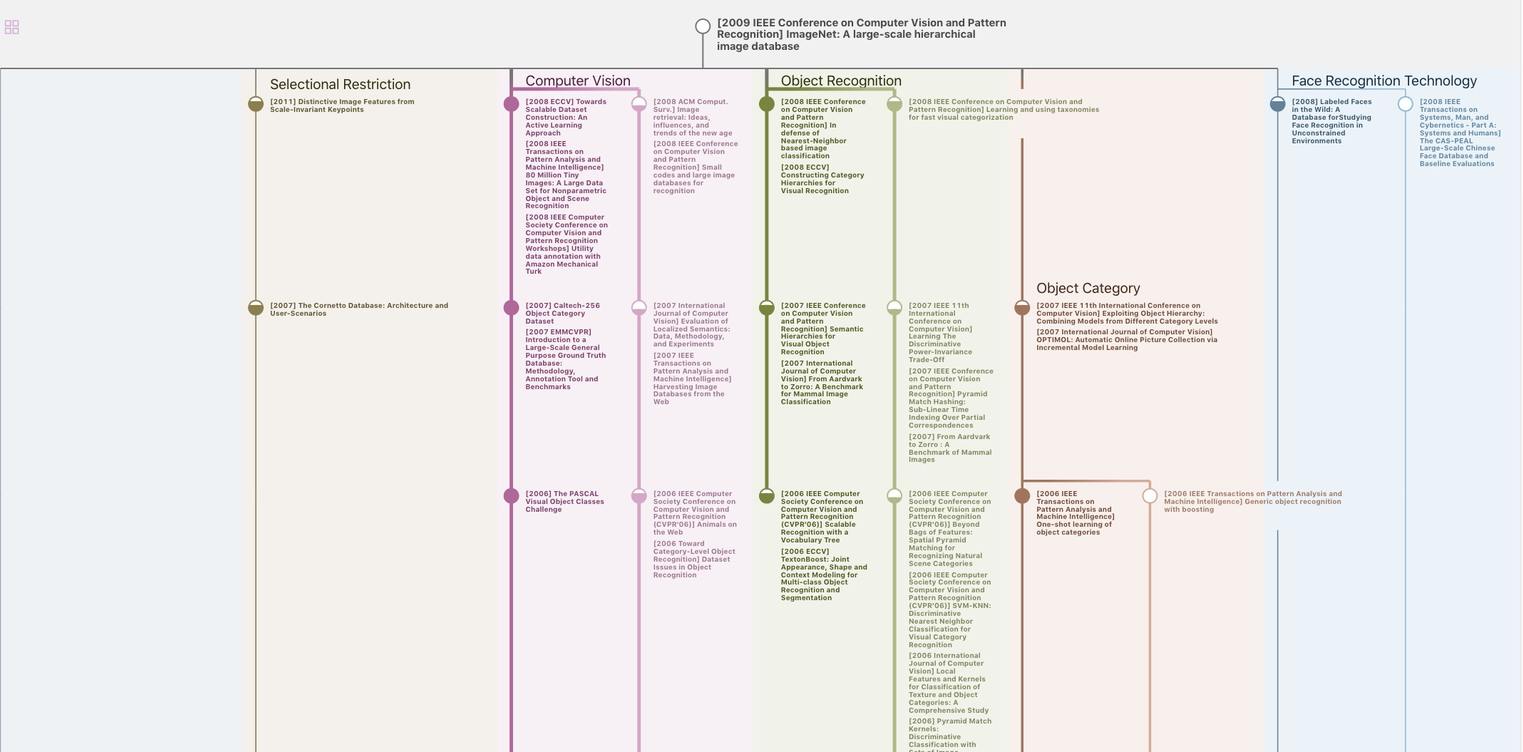
生成溯源树,研究论文发展脉络
Chat Paper
正在生成论文摘要