Selecting Features with Class Based and Importance Weighted Document Frequency in Text Classification.
DOCENG(2016)
摘要
ABSTRACTDocument Frequency (DF), which counts how many documents a feature appears in, is reported by Yang and Pedersen [1] to be quite effective for feature selection in text classification. Features with the same DF value are likely to have different appearance distribution over categories, and demonstrate quite different discriminative powers for classification. However, the original DF metric is class independent and does not consider features' distribution over classes. On the other hand, different features play different roles in delivering the content of a document. The chosen features are expected to be the important ones, which carry the main information of a document collection. However, the traditional DF metric considers features equally important. To overcome simultaneously the above two problems of the original document frequency metric, we propose a class based and importance weighted document frequency measure. Preliminary experiments on two text classification datasets do validate the effectiveness of the proposed metric.
更多查看译文
AI 理解论文
溯源树
样例
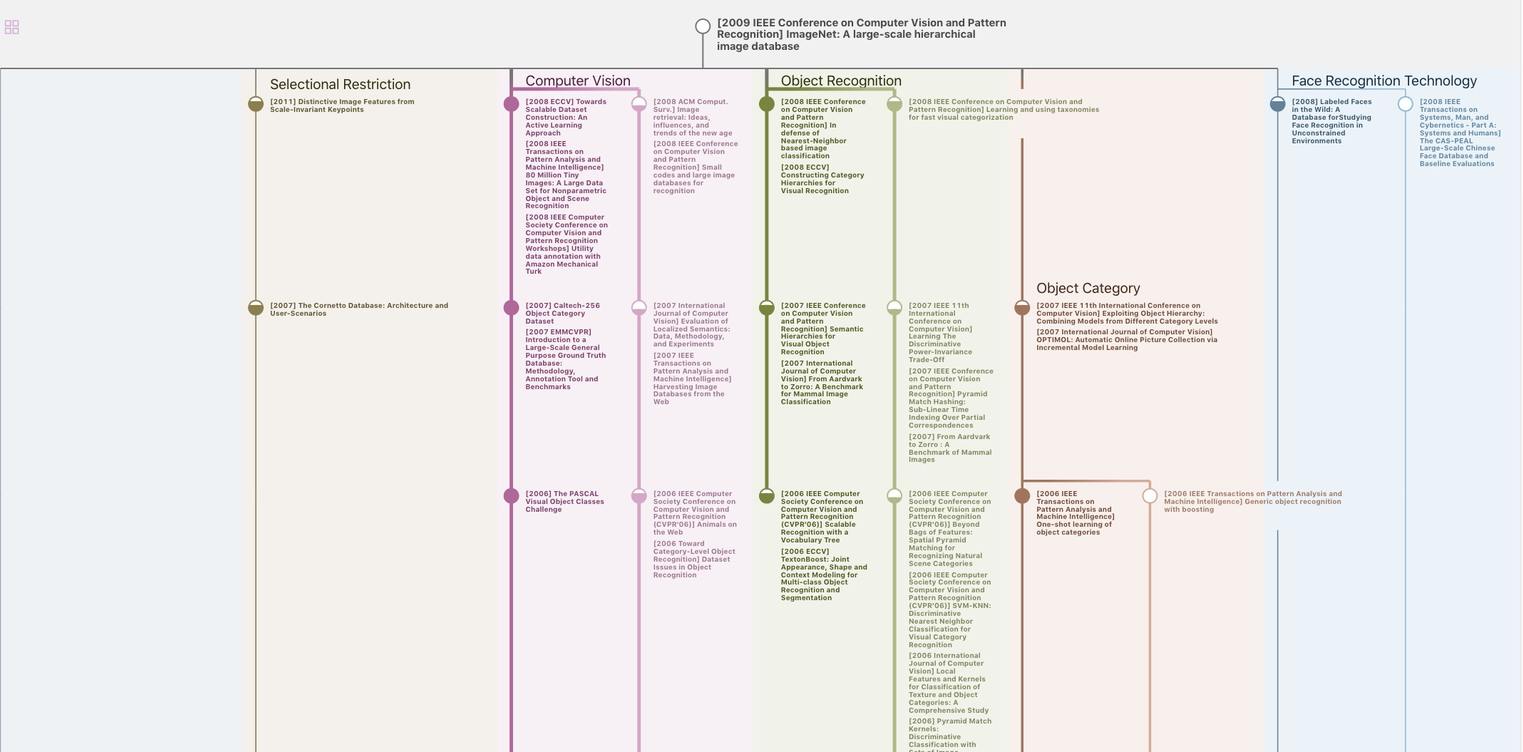
生成溯源树,研究论文发展脉络
Chat Paper
正在生成论文摘要