Parameters estimate of Riemannian Gaussian distribution in the manifold of covariance matrices
2016 IEEE Sensor Array and Multichannel Signal Processing Workshop (SAM)(2016)
摘要
The study of P
m
, the manifold of m × m symmetric positive definite matrices, has recently become widely popular in many engineering applications, like radar signal processing, mechanics, computer vision, image processing, and medical imaging. A large body of literature is devoted to the barycentre of a set of points in P
m
and the concept of barycentre has become essential to many applications and procedures, for instance classification of SPD matrices. However this concept is often used alone in order to define and characterize a set of points. Less attention is paid to the characterization of the shape of samples in the manifold, or to the definition of a probabilistic model, to represent the statistical variability of data in P
m
. Here we consider Gaussian distributions and mixtures of Gaussian distributions on P
m
. In particular we deal with parameter estimation of such distributions. This problem, while it is simple in the manifold P
2
, becomes harder for higher dimensions, since there are some quantities involved whose analytic expression is difficult to derive. In this paper we introduce a smooth estimate of these quantities using convex cubic splines, and we show that in this case the parameters estimate is coherent with theoretical results. We also present some simulations and a real EEG data analysis.
更多查看译文
关键词
parameters estimation,Riemannian Gaussian distribution,covariance matrices,radar signal processing,computer vision,image processing,medical imaging,barycentre,SPD matrices classification,probabilistic model,statistical variability,convex cubic splines,EEG data analysis,symmetric positive definite matrices
AI 理解论文
溯源树
样例
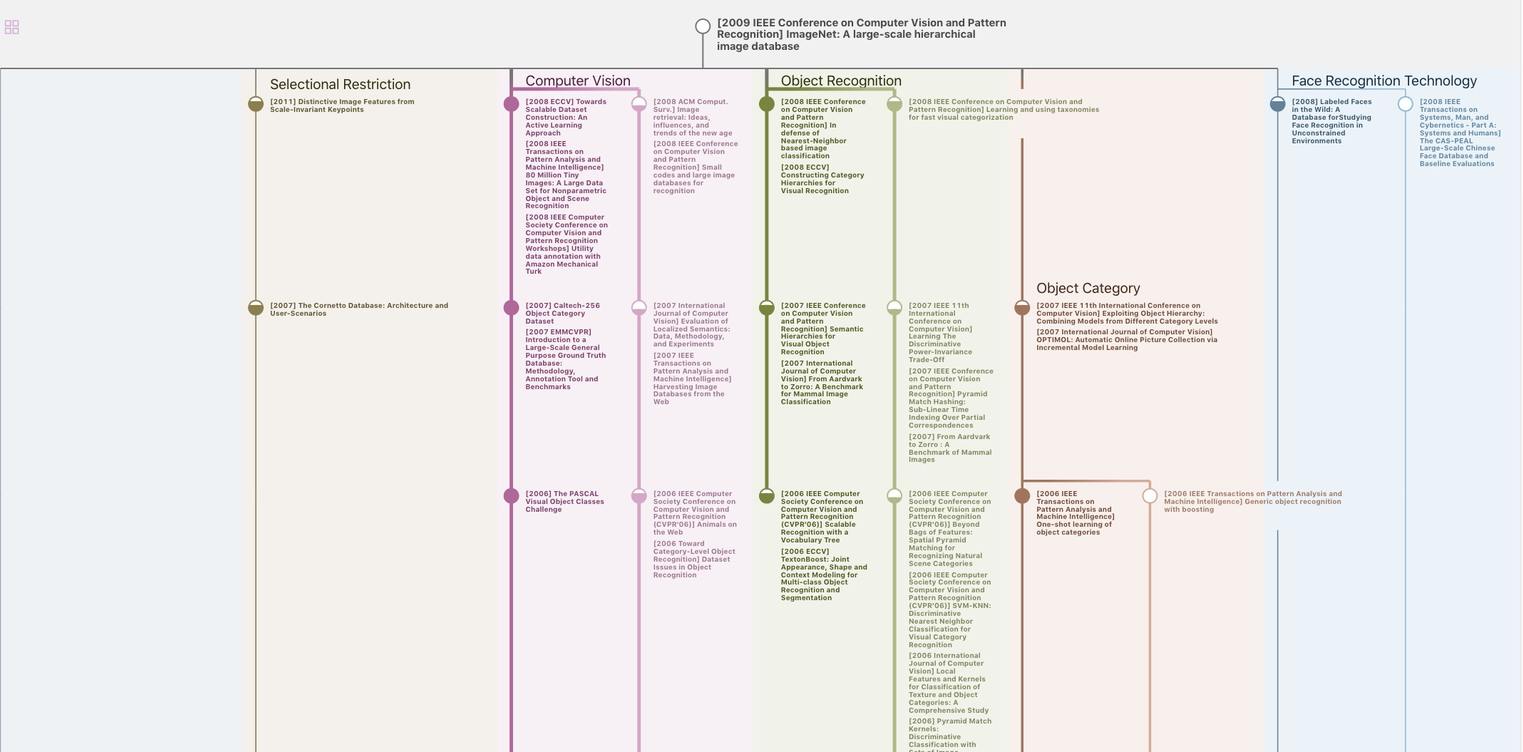
生成溯源树,研究论文发展脉络
Chat Paper
正在生成论文摘要