A Kernel-Based Sarsa( \lambda ) Algorithm with Clustering-Based Sample Sparsification.
Lecture Notes in Computer Science(2016)
摘要
In the past several decades, as a significant class of solutions to the large scale or continuous space control problems, kernel-based reinforcement learning (KBRL) methods have been a research hotspot. While the existing sample sparsification methods of KBRL exist the problems of low time efficiency and poor effect. For this problem, we propose a new sample sparsification method, clustering-based novelty criterion (CNC), which combines a clustering algorithm with a distance-based novelty criterion. Besides, we propose a clustering-based selective kernel Sarsa(lambda) (CSKS(lambda)) on the basis of CNC, which applies Sarsa(lambda) to learning parameters of the selective kernel-based value function based on local validity. Finally, we illustrate that our CSKS(lambda) surpasses other state-of-the-art algorithms by Acrobot experiment.
更多查看译文
关键词
Reinforcement learning,Kernel method,Sample sparsification,Clustering,Sarsa(lambda)
AI 理解论文
溯源树
样例
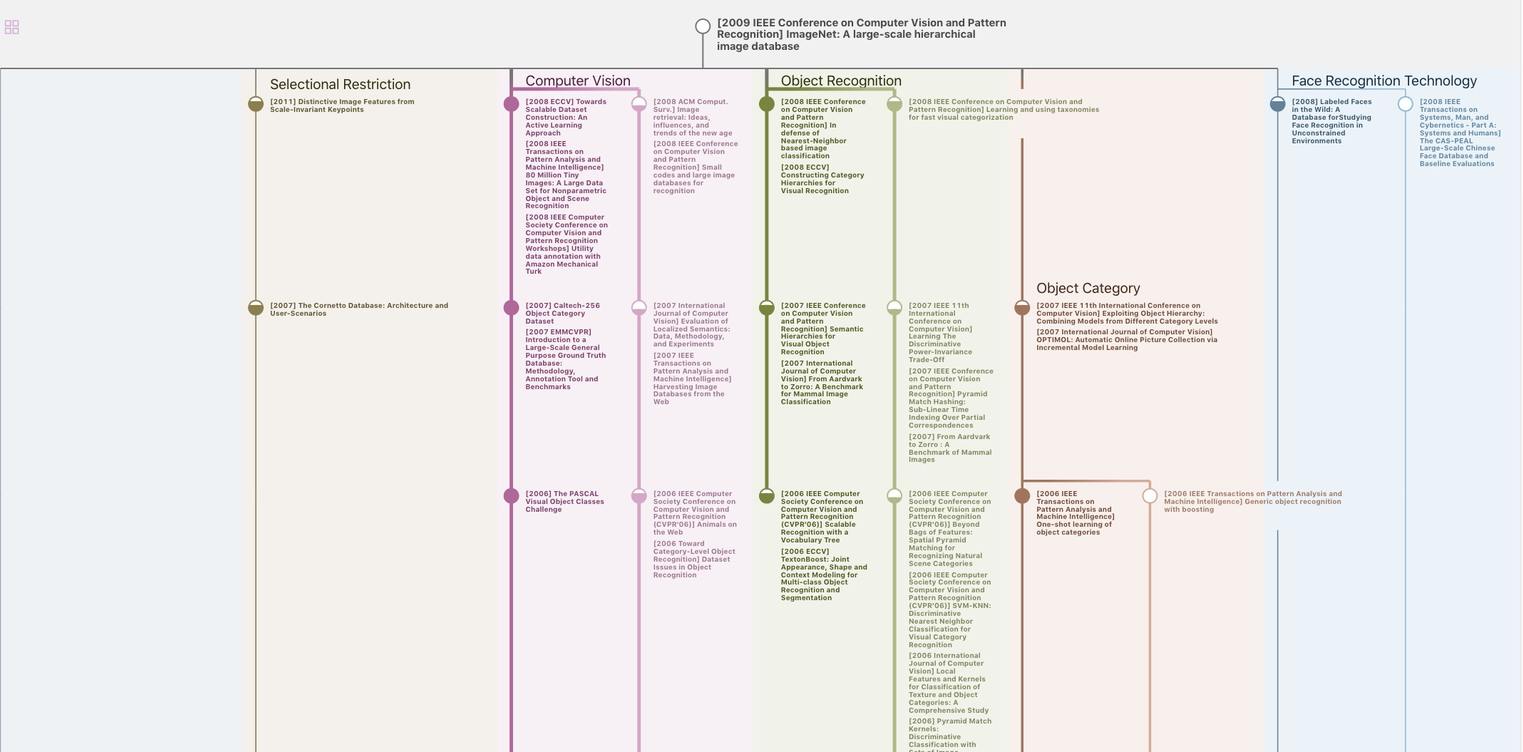
生成溯源树,研究论文发展脉络
Chat Paper
正在生成论文摘要