Locality-Sensitive Linear Bandit Model for Online Social Recommendation.
Lecture Notes in Computer Science(2016)
摘要
Recommender systems provide personalized suggestions by learning users' preference based on their historical feedback. To alleviate the heavy relying on historical data, several online recommendation methods are recently proposed and have shown the effectiveness in solving data sparsity and cold start problems in recommender systems. However, existing online recommendation methods neglect the use of social connections among users, which has been proven as an effective way to improve recommendation accuracy in offline settings. In this paper, we investigate how to leverage social connections to improve online recommendation performance. In particular, we formulate the online social recommendation task as a contextual bandit problem and propose a Locality-sensitive Linear Bandit (LS.Lin) method to solve it. The proposed model incorporates users' local social relations into a linear contextual bandit model and is capable to deal with the dynamic changes of user preference and the network structure. We provide a theoretical analysis to the proposed LS. Lin method and then demonstrate its improved performance for online social recommendation in empirical studies compared with baseline methods.
更多查看译文
关键词
Social recommendation,Linear bandits,Online learning
AI 理解论文
溯源树
样例
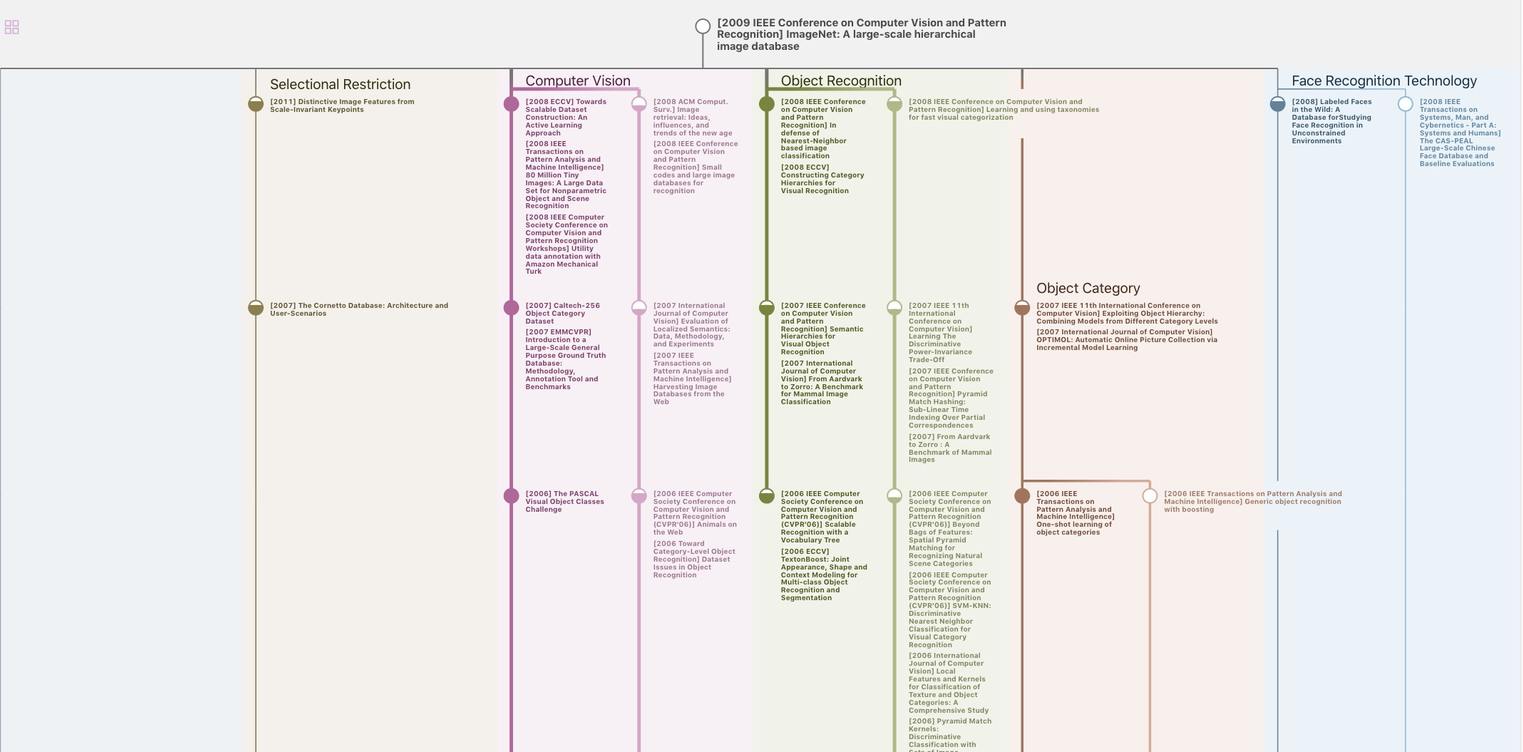
生成溯源树,研究论文发展脉络
Chat Paper
正在生成论文摘要