Early Random Shapelet Forest
DISCOVERY SCIENCE, (DS 2016)(2016)
摘要
Early classification of time series has emerged as an increasingly important and challenging problem within signal processing, especially in domains where timely decisions are critical, such as medical diagnosis in health-care. Shapelets, i.e., discriminative sub-sequences, have been proposed for time series classification as a means to capture local and phase independent information. Recently, forests of randomized shapelet trees have been shown to produce state-of-the-art predictive performance at a low computational cost. In this work, they are extended to allow for early classification of time series. An extensive empirical investigation is presented, showing that the proposed algorithm is superior to alternative state-of-the-art approaches, in case predictive performance is considered to be more important than earliness. The algorithm allows for tuning the trade-off between accuracy and earliness, thereby supporting the generation of early classifiers that can be dynamically adapted to specific needs at low computational cost.
更多查看译文
关键词
Time Series, Predictive Performance, Prediction Function, Dynamic Time Warping, Prediction Time
AI 理解论文
溯源树
样例
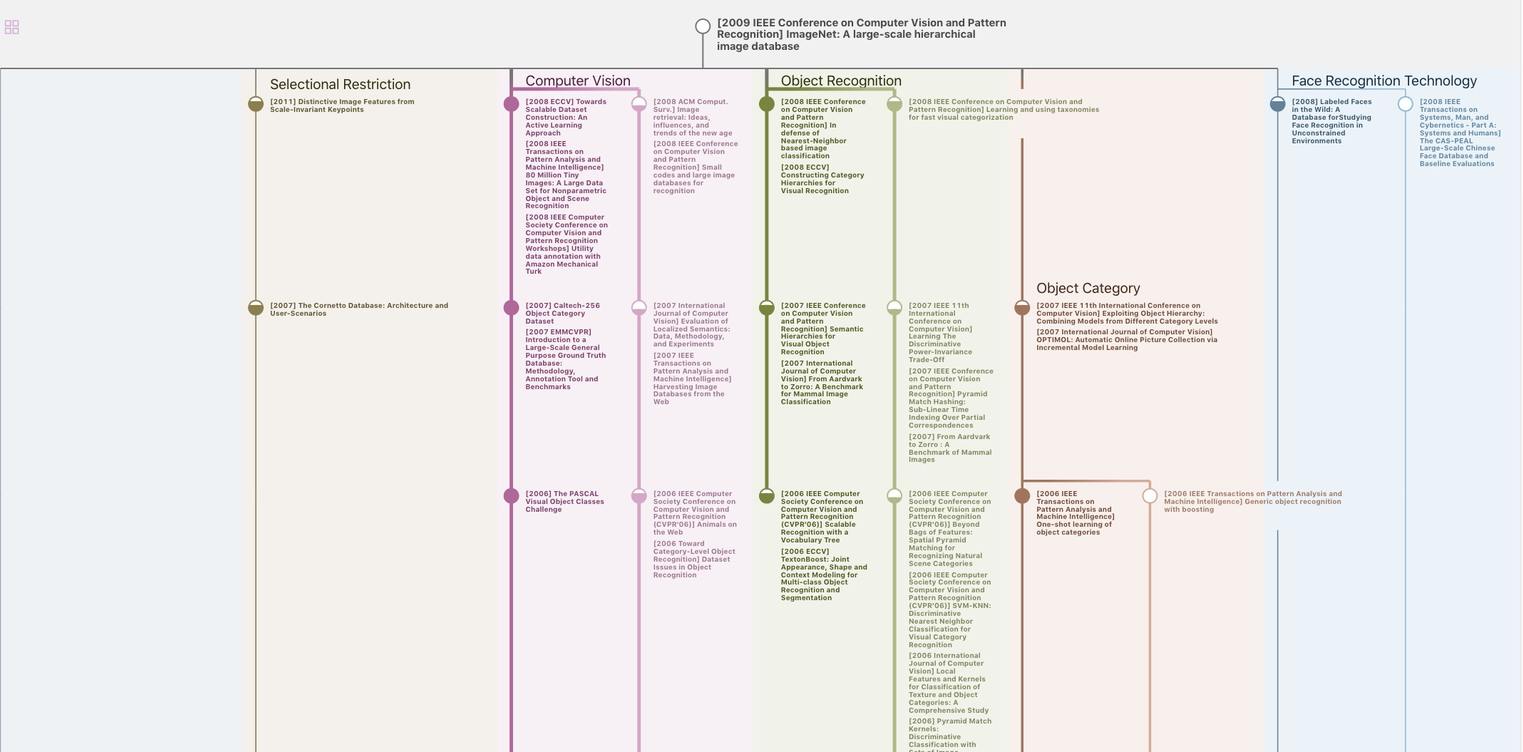
生成溯源树,研究论文发展脉络
Chat Paper
正在生成论文摘要