Differential Evolution With An Ensemble Of Low-Quality Surrogates For Expensive Optimization Problems
2016 IEEE Congress on Evolutionary Computation (CEC)(2016)
摘要
Differential Evolution (DE), a population-based stochastic search technique is adept at solving real-world optimization problems. Unlike most population based algorithms, the use of DE is usually inexpedient in solving expensive optimization problems as the computational costs of these simulations are excessively high. This problem can be resolved by commingling surrogate model in DE that approximates the output behavior of complex systems based on a limited set of expensive simulations. Surrogate models are compact and cheap to evaluate and have proven very useful for solving expensive optimization tasks. Though, the use of a surrogate model can address the expensive problems, the optimization based on a single surrogate can lead to premature convergence. DE fused with an ensemble of surrogates, each having different roles and features reports more precise results. In this paper, we present a novel method in which DE is integrated with an ensemble of low-quality surrogate models. The proposed algorithm is referred to as DE-ELS (Differential evolution with an ensemble of low-quality surrogate models) and employs polynomial regression, Kriging, and Nearest Neighbors technique for constructing the surrogates. The performance of DE-ELS is evaluated on a set of 8 bound-constrained problems and is compared with state-of-the-art algorithms belonging to IEEE-CEC 2014 competition test suite
更多查看译文
关键词
Differential Evolution,Surrogate Models,polynomial regression,kriging,Nearest Neighbors technique
AI 理解论文
溯源树
样例
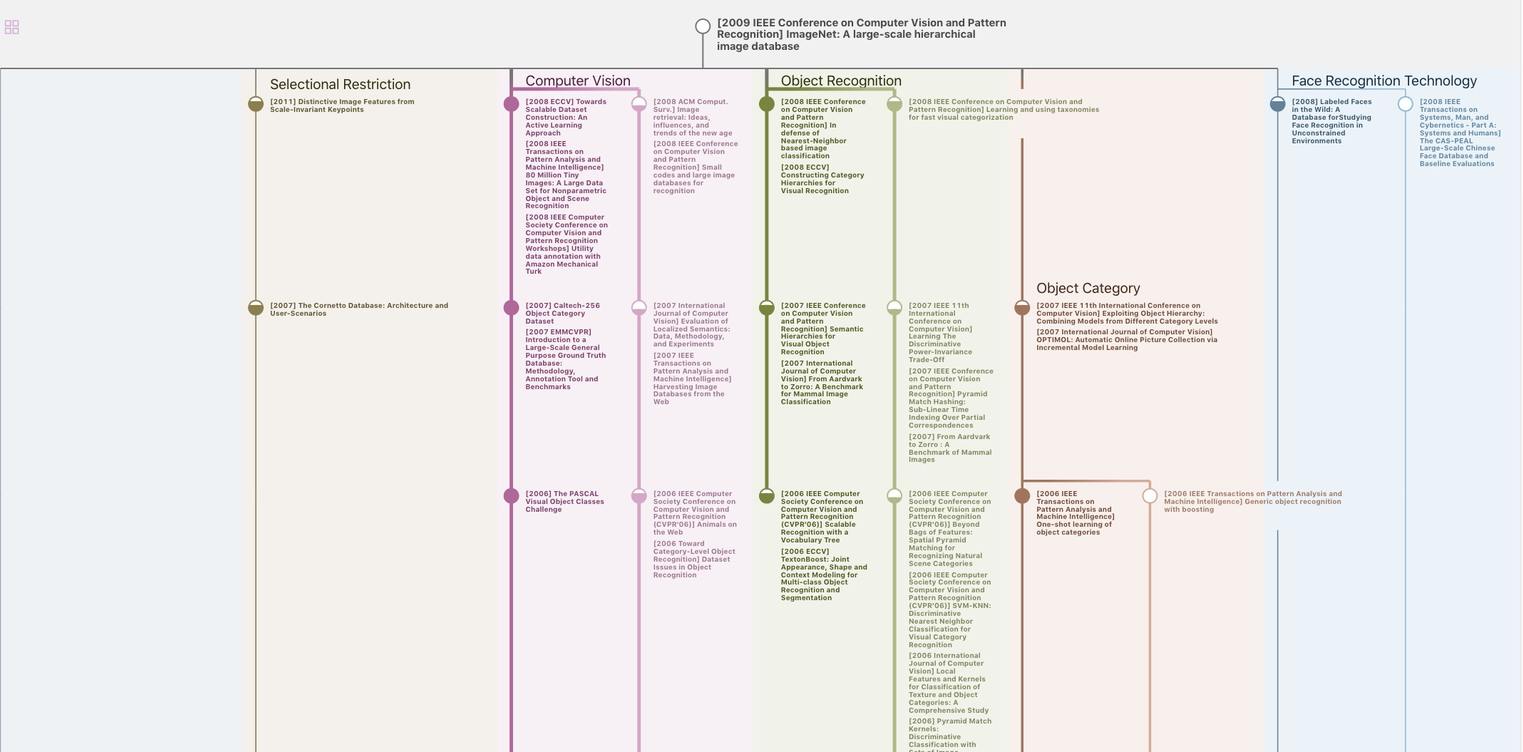
生成溯源树,研究论文发展脉络
Chat Paper
正在生成论文摘要