Towards Using Reservoir Computing Networks For Noise-Robust Image Recognition
2016 International Joint Conference on Neural Networks (IJCNN)(2016)
摘要
Reservoir Computing Network (RCN) is a special type of the single layer recurrent neural networks, in which the input and the recurrent connections are randomly generated and only the output weights are trained. Besides the ability to process temporal information, the key points of RCN are easy training and robustness against noise. Recently, we introduced a simple strategy to tune the parameters of RCN resulted in an effective and noise-robust RCN-based model for speech recognition. The aim of this work is to extend that study to the field of image processing. In particular, we investigate the potential of RCNs in achieving a competitive performance on the well-known MNIST dataset by following the aforementioned parameter optimizing strategy. Moreover, we achieve good noise robust recognition by utilizing such a network to denoise images and supplying them to a recognizer that is solely trained on clean images. The conducted experiments demonstrate that the proposed RCN-based handwritten digit recognizer achieves an error rate of 0.81 percent on the clean test data of the MNIST benchmark and that the proposed RCN-based denoiser can effectively reduce the error rate on the various types of noise.
更多查看译文
关键词
Reservoir computing networks,recurrent neural networks,text recognition,image classification,image denoising
AI 理解论文
溯源树
样例
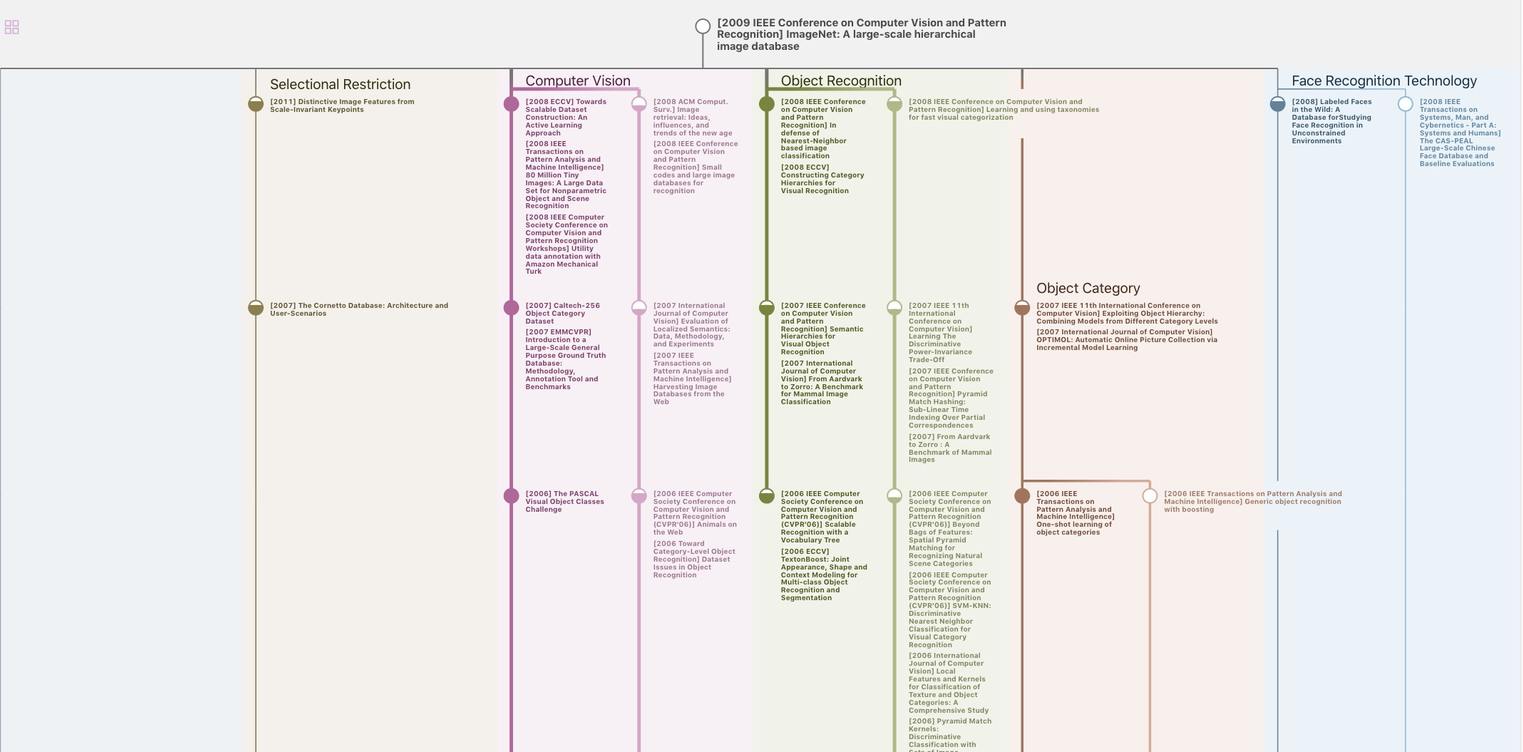
生成溯源树,研究论文发展脉络
Chat Paper
正在生成论文摘要