A Systematic Algorithm To Escape From Local Minima In Training Feed-Forward Neural Networks
2016 INTERNATIONAL JOINT CONFERENCE ON NEURAL NETWORKS (IJCNN)(2016)
摘要
A learning process is easily trapped into a local minimum when training multi-layer feed-forward neural networks. An algorithm called Wrong Output Modification (WOM) was proposed to help a learning process escape from local minima, but WOM still cannot totally solve the local minimum problem. Moreover, there is no performance analysis to show that the learning has a higher probability of converging to a global solution by using this algorithm. Additionally, the generalization performance of this algorithm was not investigated when the early stopping method of training is applied. Based on these limitations of WOM, we propose a new algorithm to ensure the learning can escape from local minima, and its performance is analyzed. We also test the generalization performance of this new algorithm when the early stopping method of training is applied.
更多查看译文
关键词
local minima,learning process,multilayer feedforward neural network training,wrong output modification,WOM,performance analysis,generalization performance,early stopping method
AI 理解论文
溯源树
样例
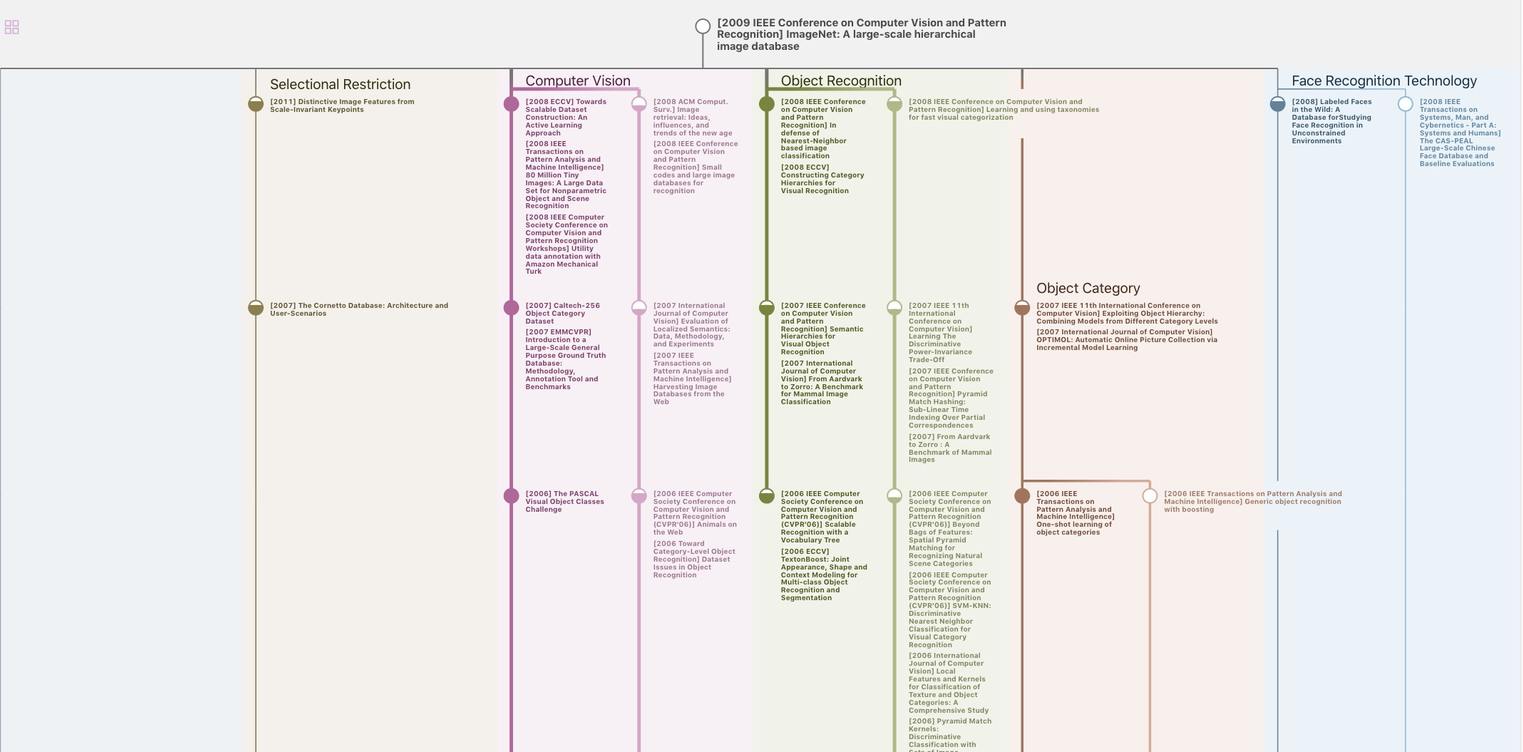
生成溯源树,研究论文发展脉络
Chat Paper
正在生成论文摘要