A Bidirectional Hierarchical Skip-Gram Model For Text Topic Embedding
2016 International Joint Conference on Neural Networks (IJCNN)(2016)
摘要
Taking advantage of the large scale corpus on the web to effectively and efficiently mine the topics within texts is an essential problem in the era of big data. We focus on the problem of learning text topic embedding in an unsupervised manner, which enjoys the properties of efficiency and scalability. Text topic embedding represents words and documents in a semantic topic space, in which the words and documents with similar topic will be embedded close to each other. When compared with conventional topic models, which implicitly capture the document-level word co-occurrence patterns, text topic embedding alleviates the data sparsity problem and captures the semantic relevance between different words and documents. To model text topic embedding, we propose a Bidirectional Hierarchical Skip-Gram model (BHSG) based on skip-gram model. BHSG includes two components: semantic generation module to learn semantic relevance between texts and topic enhance module to produce the text topic embedding based on text embedding learned in the former module. We evaluated our method on two kinds of topic-related tasks: text classification and information retrieval. The experimental results on four public datasets and one dataset we provide all demonstrate that our proposed method can achieve a better performance.
更多查看译文
关键词
bidirectional hierarchical skip-gram model,text topic embedding,large scale corpus,World Wide Web,topic mining,big data,learning,semantic topic space,data sparsity,BHSG,text classification,information retrieval
AI 理解论文
溯源树
样例
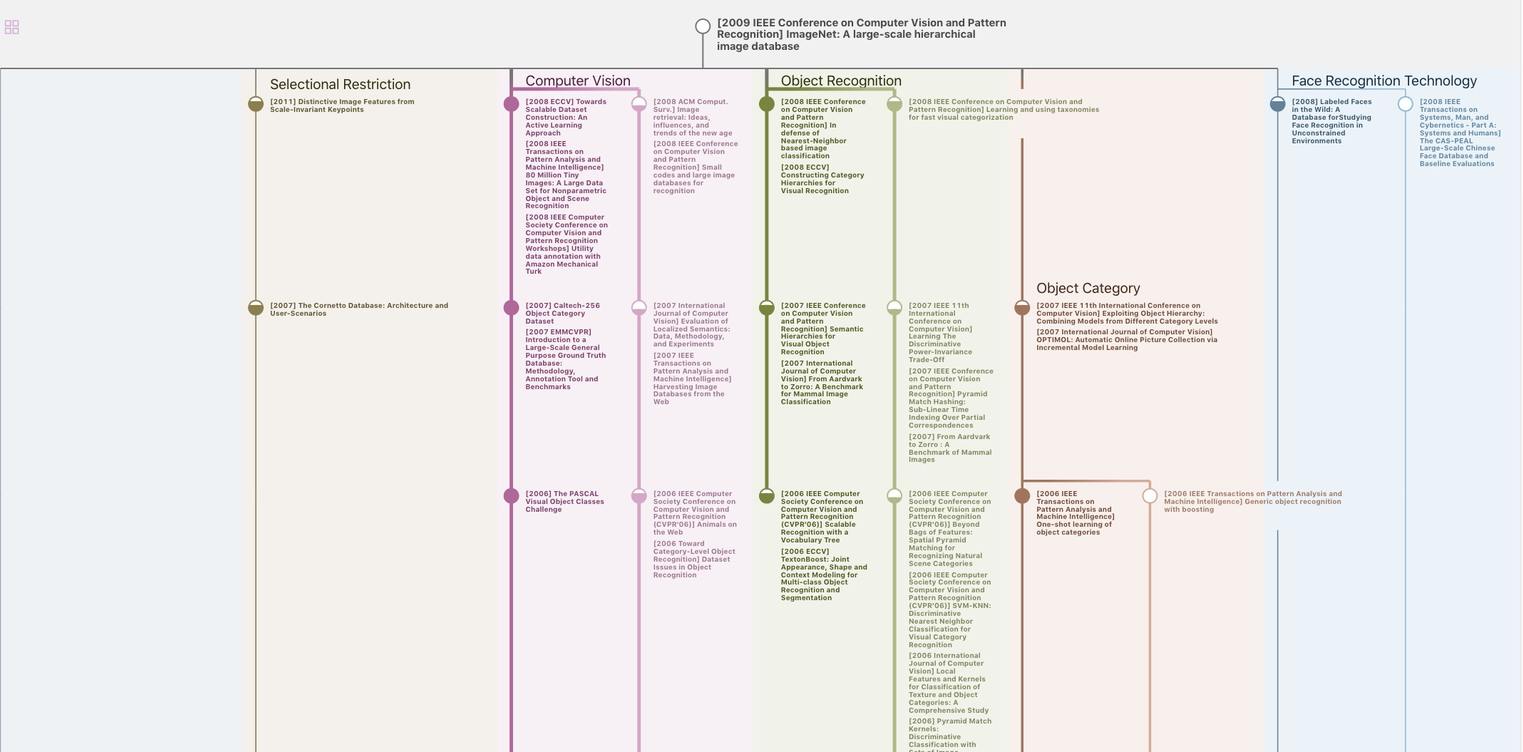
生成溯源树,研究论文发展脉络
Chat Paper
正在生成论文摘要