Manifold Regularization Based Approximate Value Iteration For Learning Control
2016 International Joint Conference on Neural Networks (IJCNN)(2016)
摘要
In this paper, we develop a model-free and data efficient batch reinforcement learning algorithm for learning control of continuous state-space and discounted-reward Markov decision processes. This algorithm is an approximate value iteration which uses the manifold regularization method to learn feature representations for Q-value function approximation. The learned features can preserve the intrinsic geometry of the state space by learning on collected samples, and thus can improve the quality of the final value function estimate and the learned policy. The effectiveness and efficiency of the proposed scheme is evaluated on a benchmark control task, i.e., the inverted pendulum balancing problem.
更多查看译文
关键词
manifold regularization based approximate value iteration,control learning,model-free data efficient batch reinforcement learning algorithm,continuous state-space processes,discounted-reward Markov decision processes,feature representations learning,Q-value function approximation,intrinsic geometry preservation,collected samples learning,value function estimate quality improvement,learned policy quality improvement,inverted pendulum balancing problem
AI 理解论文
溯源树
样例
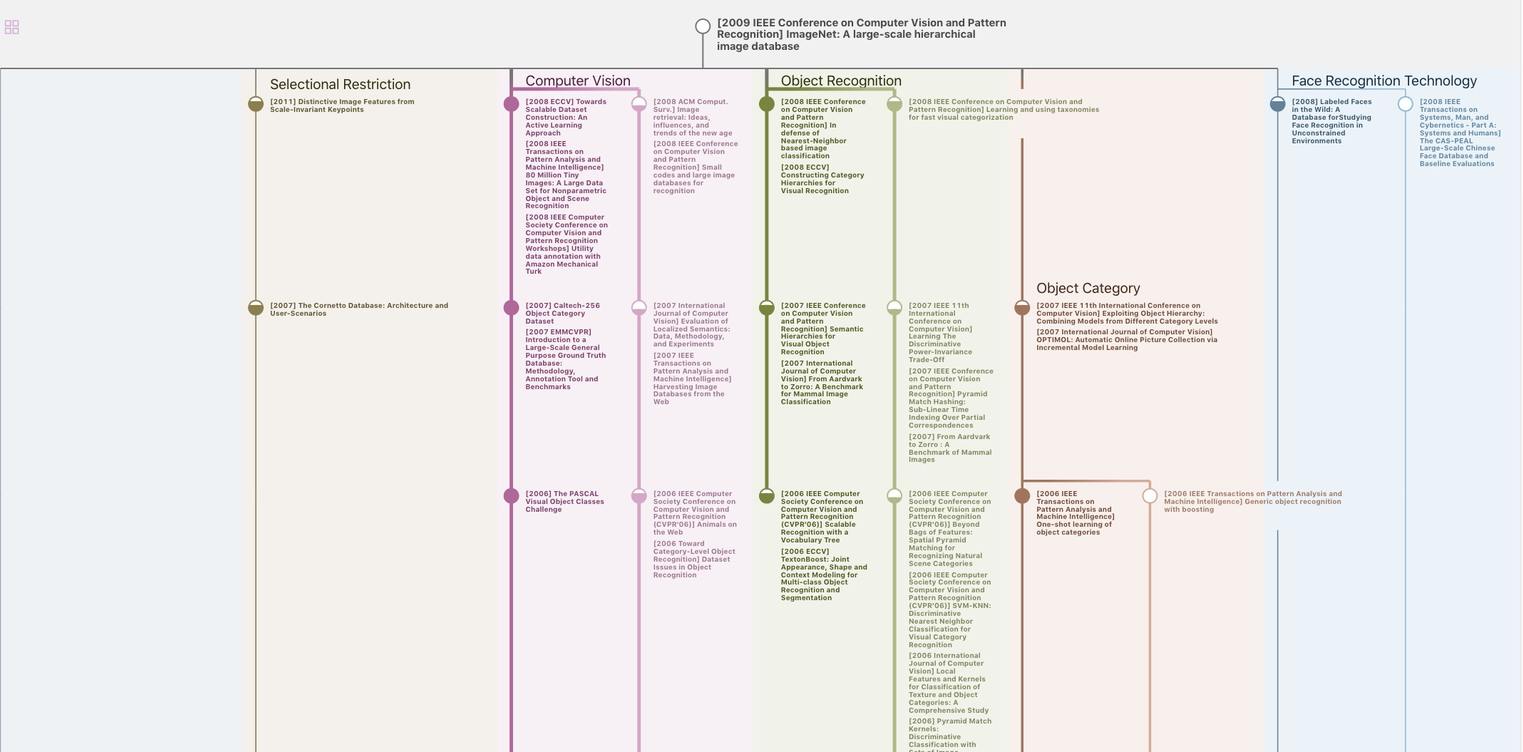
生成溯源树,研究论文发展脉络
Chat Paper
正在生成论文摘要