Scalable Large Margin Online Metric Learning
2016 International Joint Conference on Neural Networks (IJCNN)(2016)
摘要
We present a novel online metric learning model, called scalable large margin online metric learning (SLMOML). SLMOML belongs to the passive-aggressive family of learning models. In the formulation of SLMOML, we use the LogDet divergence to measure the closeness between two continuously learned matrices, which naturally ensures the positive semi-definiteness of the learned matrix at each iteration, provided the initial matrix is positive semi-definite. In addition, a hinge loss is used to maintain a large margin of distance between relatively dissimilar data. Using the Karush-Kuhn-Tucker (KKT) condition, the updating rule of SLMOML can be equivalently viewed as Bregman projections. Based on this fact, we have proved the global convergence of SLMOML. Extensive experiments on real world applications demonstrate the superiority of SLMOML over state-of-the-art metric learning and similarity learning approaches.
更多查看译文
关键词
scalable large margin online metric learning,SLMOML,learning model passive-aggressive family,LogDet divergence,closeness measurement,continuously learned matrices,learned matrix positive semidefiniteness,Karush-Kuhn-Tucker condition,KKT condition,Bregman projections,global convergence,similarity learning approaches
AI 理解论文
溯源树
样例
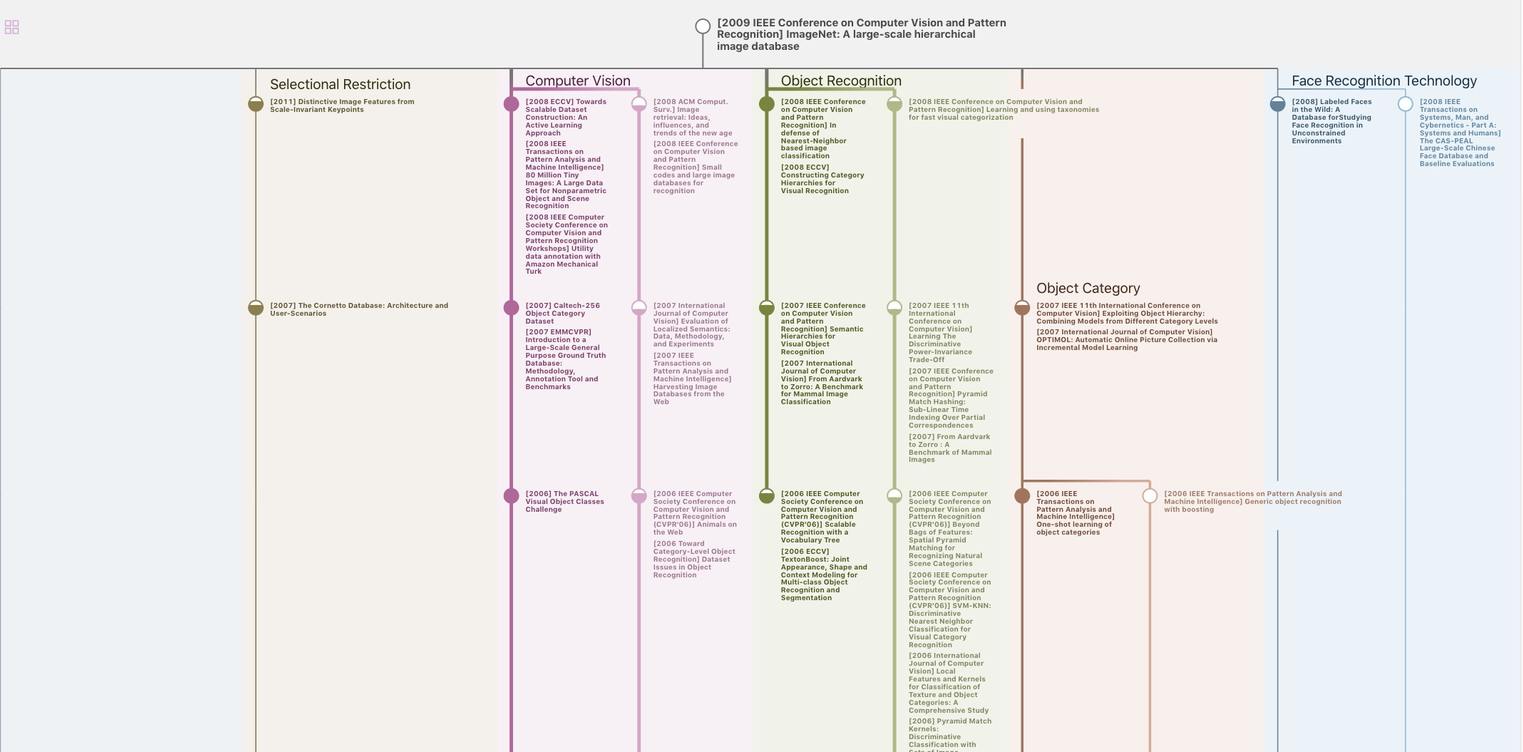
生成溯源树,研究论文发展脉络
Chat Paper
正在生成论文摘要