Maximum Class Boundary Criterion For Supervised Dimensionality Reduction
2016 International Joint Conference on Neural Networks (IJCNN)(2016)
摘要
Participation of class-wise noisy patterns may mislead the selection process of relevant patterns for subspace projection. And modelling between-class scatter for each class using the patterns that are nearer to the corresponding class decision boundary may improve the quality of feature generation. In this manuscript, a novel dimensionality reduction method, named Maximum Class Boundary Criterion (MCBC) is proposed. MCBC increases class separability by realizing the significant class-boundary and class-non-boundary patterns after the elimination of noisy patterns. The objective of MCBC is modeled such that the class-boundary patterns are pushed away from the corresponding class means and class-non-boundary patterns are forced towards their class means. As a result, the classification performance of the extracted MCBC features is improved. Experimental study is performed on UCI machine learning and face recognition data to highlight the performance of MCBC. The results conclude that MCBC can generate better discriminative features compared to the state-of-the-art dimensionality reduction methods.
更多查看译文
关键词
maximum class boundary criterion,supervised dimensionality reduction,class-wise noisy patterns participation,relevant patterns selection process,subspace projection,between-class scatter modelling,class decision boundary,feature generation,class separability,significant class-boundary realization,class-non-boundary patterns,noisy pattern elimination,classification performance,MCBC feature extraction,UCI machine learning,face recognition data
AI 理解论文
溯源树
样例
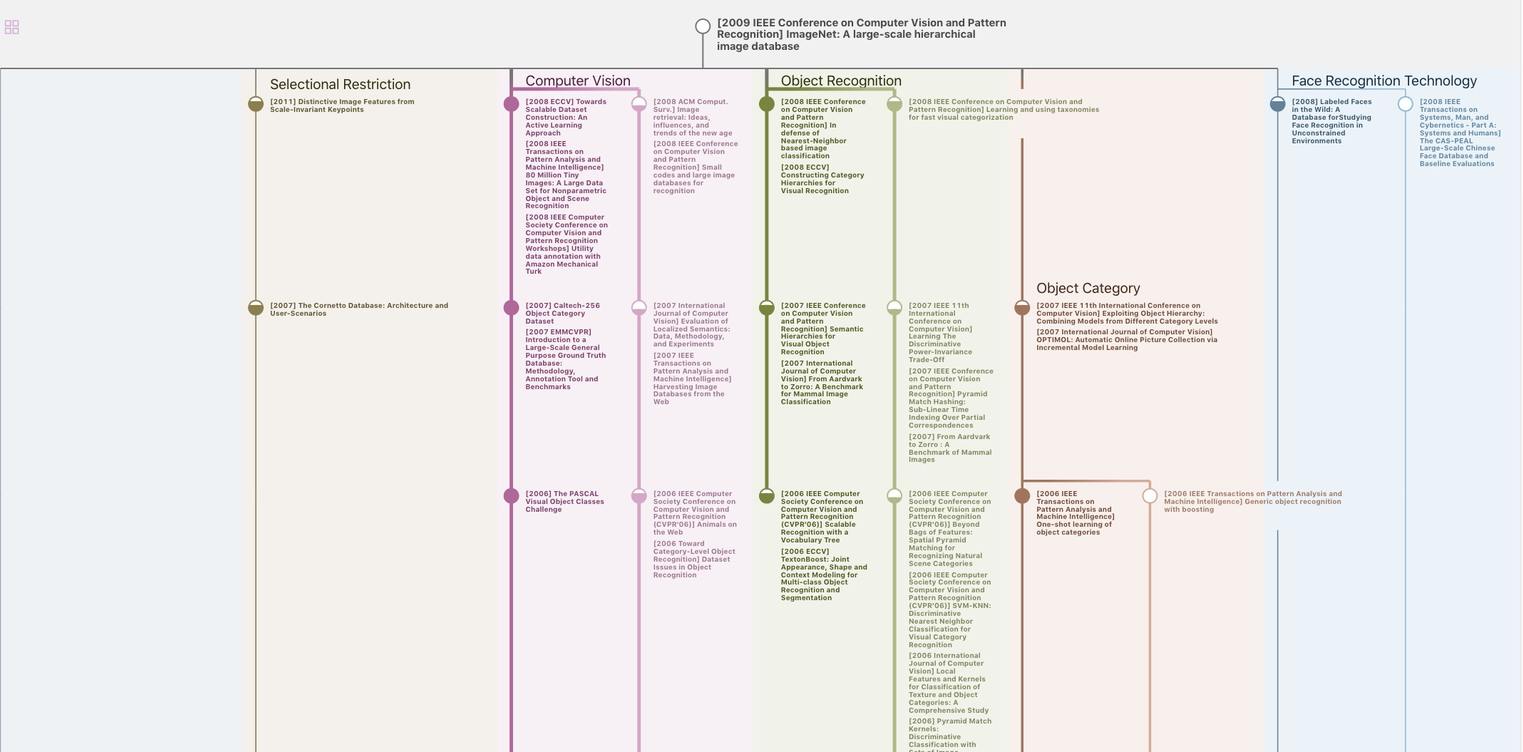
生成溯源树,研究论文发展脉络
Chat Paper
正在生成论文摘要