Which Method To Use For Optimal Structure And Function Representation Of Large Spiking Neural Networks: A Case Study On The Neucube Architecture
2016 International Joint Conference on Neural Networks (IJCNN)(2016)
摘要
This study analyses different representations of large spiking neural network (SNN) structures for conventional computers and uses the NeuCube SNN architecture as a case study. The representation includes neuronal connectivity and network's and neurons' states during the learning process. Three different structure types, namely adjacency matrix, adjacency list, and edge-weight table, were compared in terms of their storage needs and execution time performance of a learning algorithm, for varying numbers of neurons in the network. Comparative analysis shows that the adjacency list, combined with a backwards indexing mechanism, scales up most efficiently both in terms of performance and of storage requirements. The optimal algorithm was further used to simulate a large scale NeuCube system with 241,606 spiking neurons in a 3D space for prediction and analysis of benchmark spatio-temporal data.
更多查看译文
关键词
optimal structure,function representation,large spiking neural networks,NeuCube architecture,SNN structures,NeuCube SNN architecture,neuronal connectivity,adjacency matrix,adjacency list,edge-weight table,learning algorithm,backwards indexing mechanism,large scale NeuCube system,spiking neurons,benchmark spatio-temporal data
AI 理解论文
溯源树
样例
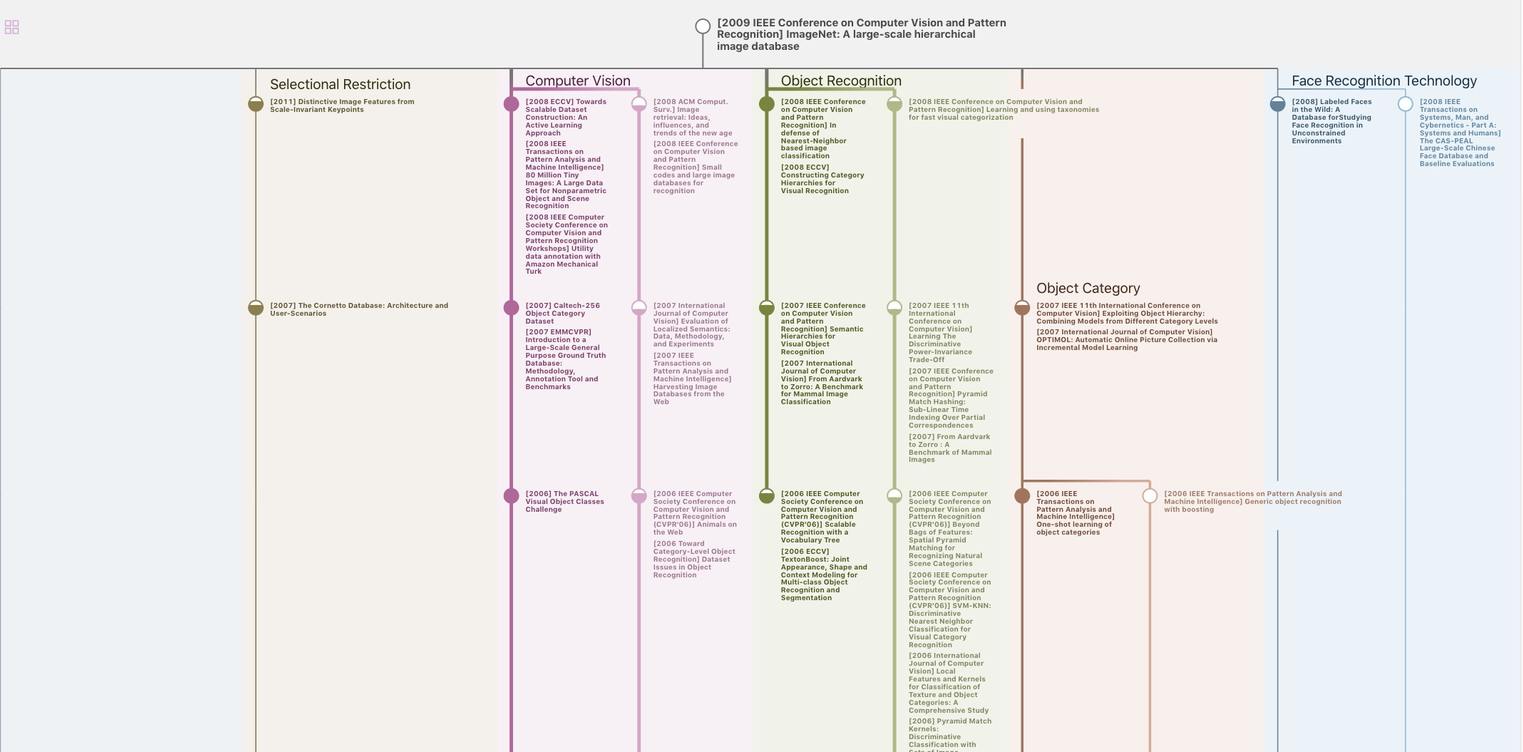
生成溯源树,研究论文发展脉络
Chat Paper
正在生成论文摘要