Sketch Metric Learning
2016 International Joint Conference on Neural Networks (IJCNN)(2016)
摘要
The main theme of this paper is to develop a systematic framework to learn a Mahalanobis distance metric based on matrix sketching. Within this framework, we present a novel sketch metric learning algorithm which sequentially sketches the received samples from training dataset and formulates a new kind of constraint for metric learning. This is in contrast to the traditional constraints that are only consisted of data from training dataset. In this paper, one training instance in the constraint is replaced by a pseudo center, which is generated during the sketching stage. Due to this change, our learning algorithm can focus on pushing every received sample to its corresponding similar pseudo center closer and pulling it far away from the dissimilar one. In addition, it can further achieve better performance of some kinds of time-varying process (e.g. on object tracking) than the compared related competitors. We demonstrate how to implement other methods in our algorithm framework and experiment to show that our method outperforms the competitors and relevant baselines on multiple datasets.
更多查看译文
关键词
Mahalanobis distance metric,matrix sketching,sketch metric learning algorithm,pseudo center,time-varying process
AI 理解论文
溯源树
样例
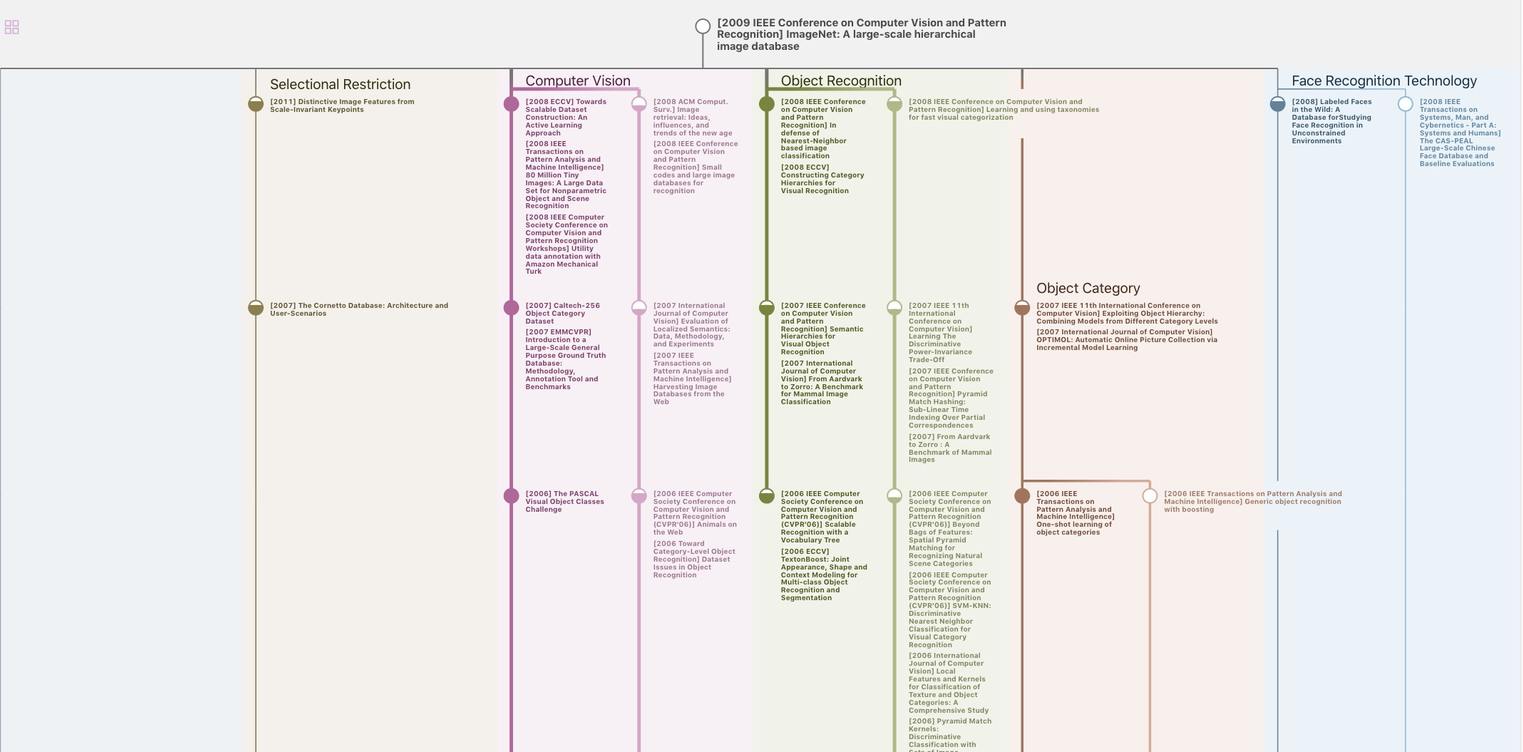
生成溯源树,研究论文发展脉络
Chat Paper
正在生成论文摘要