A Comparative Study of Social Network Classifiers for Predicting Churn in the Telecommunication Industry
2016 IEEE/ACM International Conference on Advances in Social Networks Analysis and Mining (ASONAM)(2020)
摘要
Relational learning in networked data has been shown to be effective in a number of studies. Relational learners, composed of relational classifiers and collective inference methods, enable the inference of nodes in a network given the existence and strength of links to other nodes. These methods have been adapted to predict customer churn in telecommunication companies showing that incorporating them may give more accurate predictions. In this research, the performance of a variety of relational learners is compared by applying them to a number of CDR datasets originating from the telecommunication industry, with the goal to rank them as a whole and investigate the effects of relational classifiers and collective inference methods separately. Our results show that collective inference methods do not improve the performance of relational classifiers and the best performing relational classifier is the network-only link-based classifier, which builds a logistic model using link-based measures for the nodes in the network.
更多查看译文
关键词
social network classifiers,customer churn prediction,telecommunication industry,relational learning,networked data,relational learners,collective inference methods,telecommunication companies,CDR datasets,network-only link-based classifier,logistic model,link-based measures
AI 理解论文
溯源树
样例
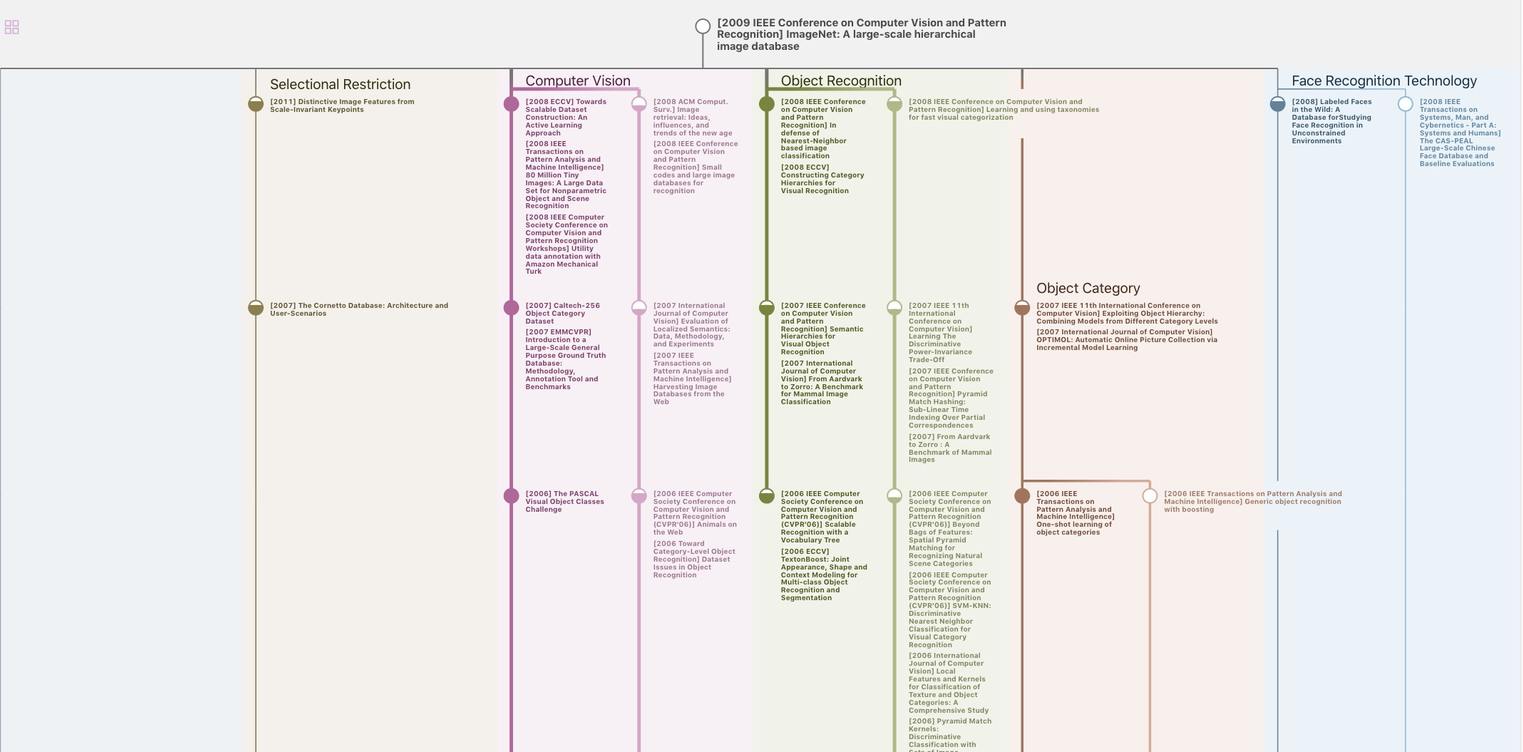
生成溯源树,研究论文发展脉络
Chat Paper
正在生成论文摘要