Adaptive Multi-class Correlation Filters.
PCM(2016)
摘要
Correlation filters have attracted growing attention due to their high efficiency, which have been well studied for binary classification. However, by setting the desired output to be a fixed Gaussian function, the conventional multi-class classification based on correlation filters becomes problematic due to the under-fitting in many real-world applications. In this paper, we propose an adaptive multi-class correlation filters (AMCF) method based on an alternating direction method of multipliers (ADMM) framework. Within this framework, we introduce an adaptive output to alleviate the under-fitting problem in the ADMM iterations. By doing so, a closed-form sub-solution is obtained and further used to constrain the optimization objective, simplifying the entire inference mechanism. The proposed approach is successfully combined with the Histograms of Oriented Gradients (HOG) features, multi-channel features and convolution features, and achieves superior performances over state-of-the-arts in two multi-class classification tasks including handwritten digits recognition and RGBD-based action recognition.
更多查看译文
关键词
Multi-class correlation filters, ADMM, Adaptive output
AI 理解论文
溯源树
样例
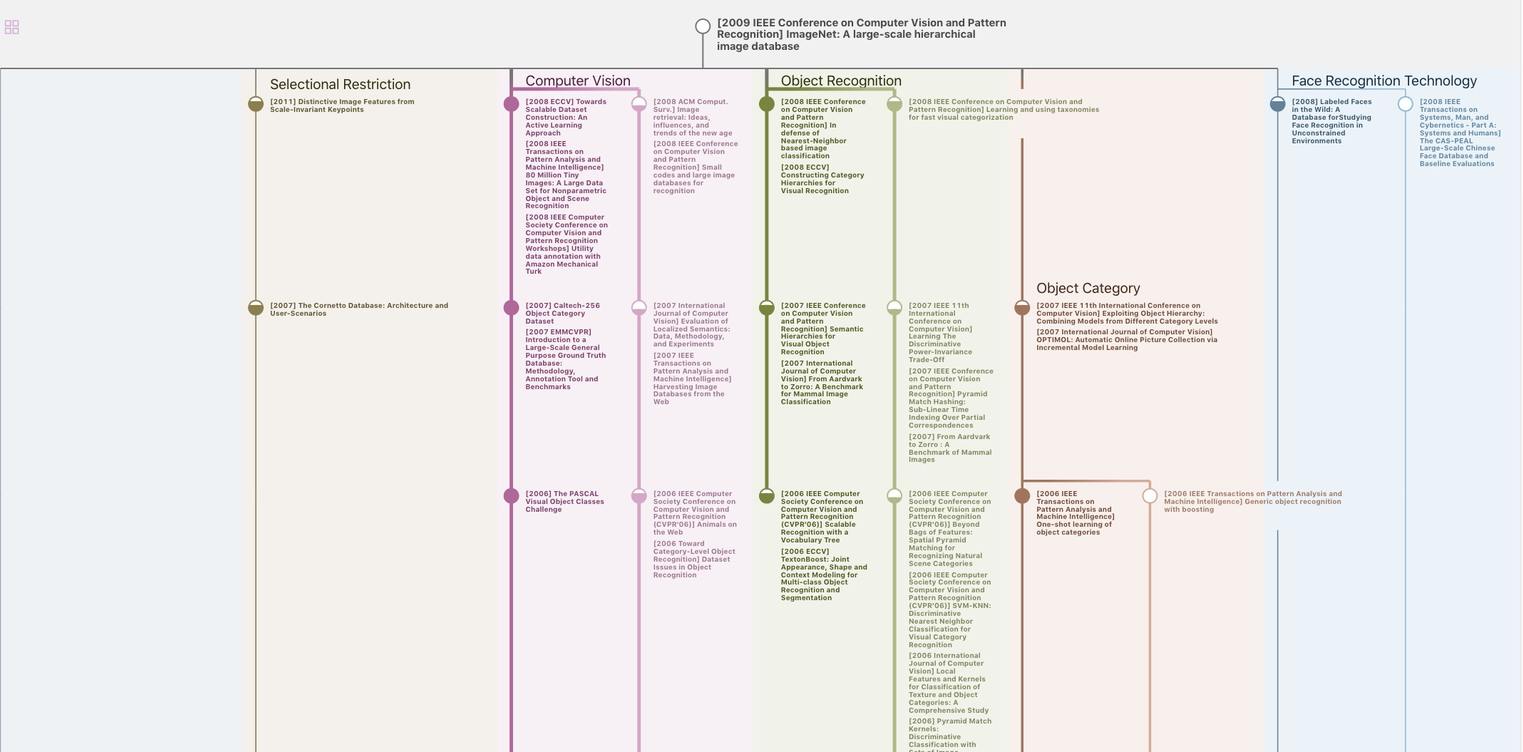
生成溯源树,研究论文发展脉络
Chat Paper
正在生成论文摘要