Spleap: Soft Pooling Of Learned Parts For Image Classification
COMPUTER VISION - ECCV 2016, PT VIII(2016)
摘要
The aggregation of image statistics - the so-called pooling step of image classification algorithms - as well as the construction of part-based models, are two distinct and well-studied topics in the literature. The former aims at leveraging a whole set of local descriptors that an image can contain (through spatial pyramids or Fisher vectors for instance) while the latter argues that only a few of the regions an image contains are actually useful for its classification. This paper bridges the two worlds by proposing a new pooling framework based on the discovery of useful parts involved in the pooling of local representations. The key contribution lies in a model integrating a boosted non-linear part classifier as well as a parametric soft-max pooling component, both trained jointly with the image classifier. The experimental validation shows that the proposed model not only consistently surpasses standard pooling approaches but also improves over state-of-the-art part-based models, on several different and challenging classification tasks.
更多查看译文
关键词
Training Image,Image Classification,Association Rule Mining,Weak Classifier,Weak Learner
AI 理解论文
溯源树
样例
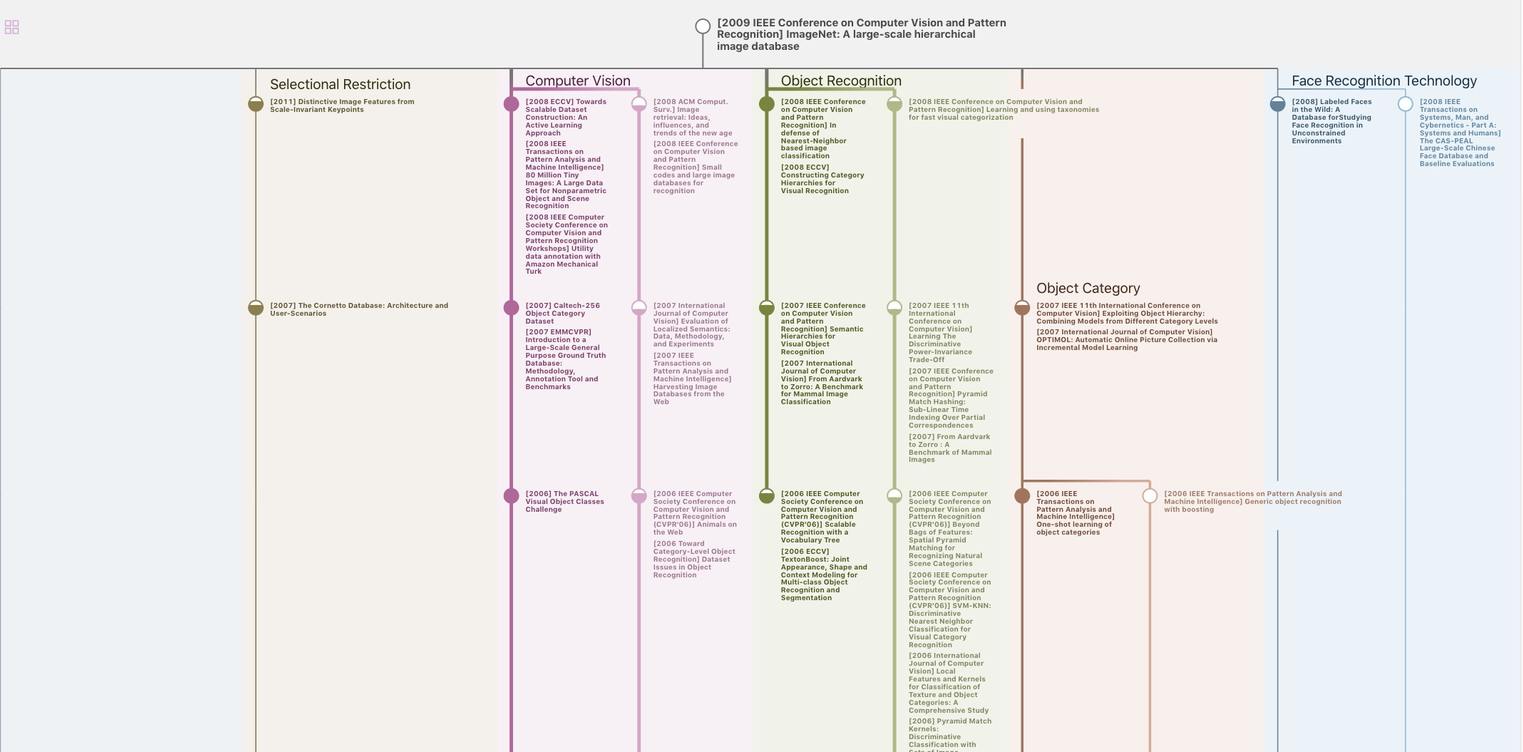
生成溯源树,研究论文发展脉络
Chat Paper
正在生成论文摘要