L(0)-Sparse Subspace Clustering
European Conference on Computer Vision(2016)
摘要
Subspace clustering methods with sparsity prior, such as Sparse Subspace Clustering (SSC) [1], are effective in partitioning the data that lie in a union of subspaces. Most of those methods require certain assumptions, e.g. independence or disjointness, on the subspaces. These assumptions are not guaranteed to hold in practice and they limit the application of existing sparse subspace clustering methods. In this paper, we propose l(0)-induced sparse subspace clustering (l(0)-SSC). In contrast to the required assumptions, such as independence or disjointness, on subspaces for most existing sparse subspace clustering methods, we prove that subspace-sparse representation, a key element in subspace clustering, can be obtained by l(0)-SSC for arbitrary distinct underlying subspaces almost surely under the mild i.i.d. assumption on the data generation. We also present the "no free lunch" theorem that obtaining the subspace representation under our general assumptions can not be much computationally cheaper than solving the corresponding l(0) problem of l(0)-SSC. We develop a novel approximate algorithm named Approximate l(0)-SSC (Al-0-SSC) that employs proximal gradient descent to obtain a sub-optimal solution to the optimization problem of l(0)-SSC with theoretical guarantee, and the sub-optimal solution is used to build a sparse similarity matrix for clustering. Extensive experimental results on various data sets demonstrate the superiority of Al-0-SSC compared to other competing clustering methods.
更多查看译文
关键词
Sparse subspace clustering, Proximal gradient descent
AI 理解论文
溯源树
样例
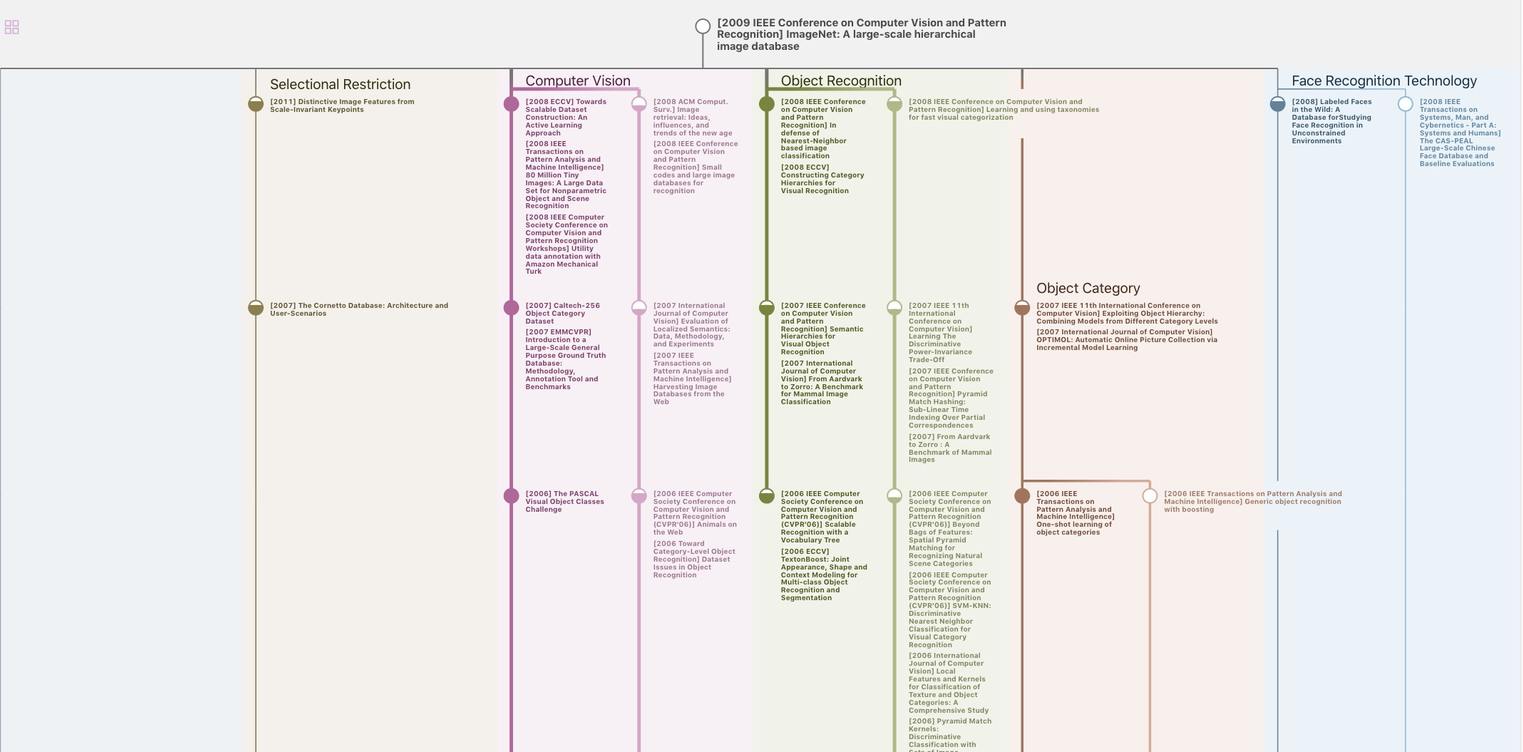
生成溯源树,研究论文发展脉络
Chat Paper
正在生成论文摘要