Class-Specific Object Pose Estimation And Reconstruction Using 3d Part Geometry
COMPUTER VISION - ECCV 2016 WORKSHOPS, PT III(2016)
摘要
We propose a novel approach for detecting and reconstructing class-specific objects from 2D images. Reconstruction and detection, despite major advances, are still wanting in performance. Hence, approaches that try to solve them jointly, so that one can be used to resolve the ambiguities of the other, especially while employing data-driven class-specific learning, are increasingly popular. In this paper, we learn a deformable, fine-grained, part-based model from real world, class-specific, image sequences, so that given a new image, we can simultaneously estimate the 3D shape, viewpoint and the subsequent 2D detection results. This is a step beyond existing approaches, which are usually limited to 3D CAD shapes, regression based pose estimation, template based deformation modelling etc. We employ Structure from Motion (SfM) and part based models in our learning process, and estimate a 3D deformable object instance and a projection matrix that explains the image information. We demonstrate our approach with high quality qualitative and quantitative results on our real world RealCar dataset, as well as the EPFL car dataset.
更多查看译文
关键词
Radial Basis Function Neural Network,Convolutional Neural Network,Appearance Model,Angular Error,Object Instance
AI 理解论文
溯源树
样例
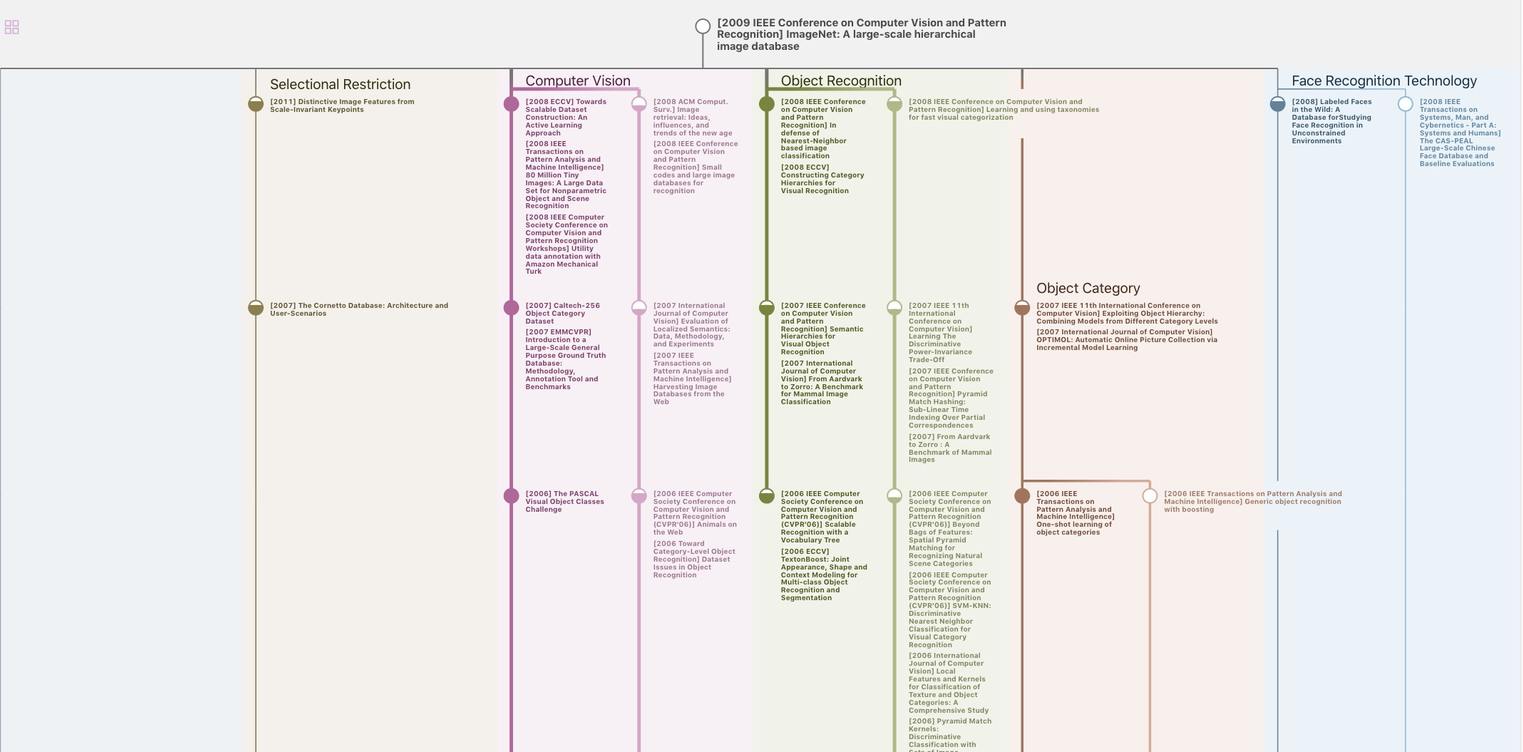
生成溯源树,研究论文发展脉络
Chat Paper
正在生成论文摘要