Generic 3d Representation Via Pose Estimation And Matching
COMPUTER VISION - ECCV 2016, PT III(2017)
摘要
Though a large body of computer vision research has investigated developing generic semantic representations, efforts towards developing a similar representation for 3D has been limited. In this paper, we learn a generic 3D representation through solving a set of foundational proxy 3D tasks: object-centric camera pose estimation and wide baseline feature matching. Our method is based upon the premise that by providing supervision over a set of carefully selected foundational tasks, generalization to novel tasks and abstraction capabilities can be achieved. We empirically show that the internal representation of a multi-task ConvNet trained to solve the above core problems generalizes to novel 3D tasks (e.g., scene layout estimation, object pose estimation, surface normal estimation) without the need for fine-tuning and shows traits of abstraction abilities (e.g., cross modality pose estimation).In the context of the core supervised tasks, we demonstrate our representation achieves state-of-the-art wide baseline feature matching results without requiring apriori rectification (unlike SIFT and the majority of learnt features). We also show 6DOF camera pose estimation given a pair local image patches. The accuracy of both supervised tasks come comparable to humans. Finally, we contribute a large-scale dataset composed of object-centric street view scenes along with point correspondences and camera pose information, and conclude with a discussion on the learned representation and open research questions.
更多查看译文
关键词
Generic vision, Representation, Descriptor learning, Pose estimation, Wide-baseline matching, Street view
AI 理解论文
溯源树
样例
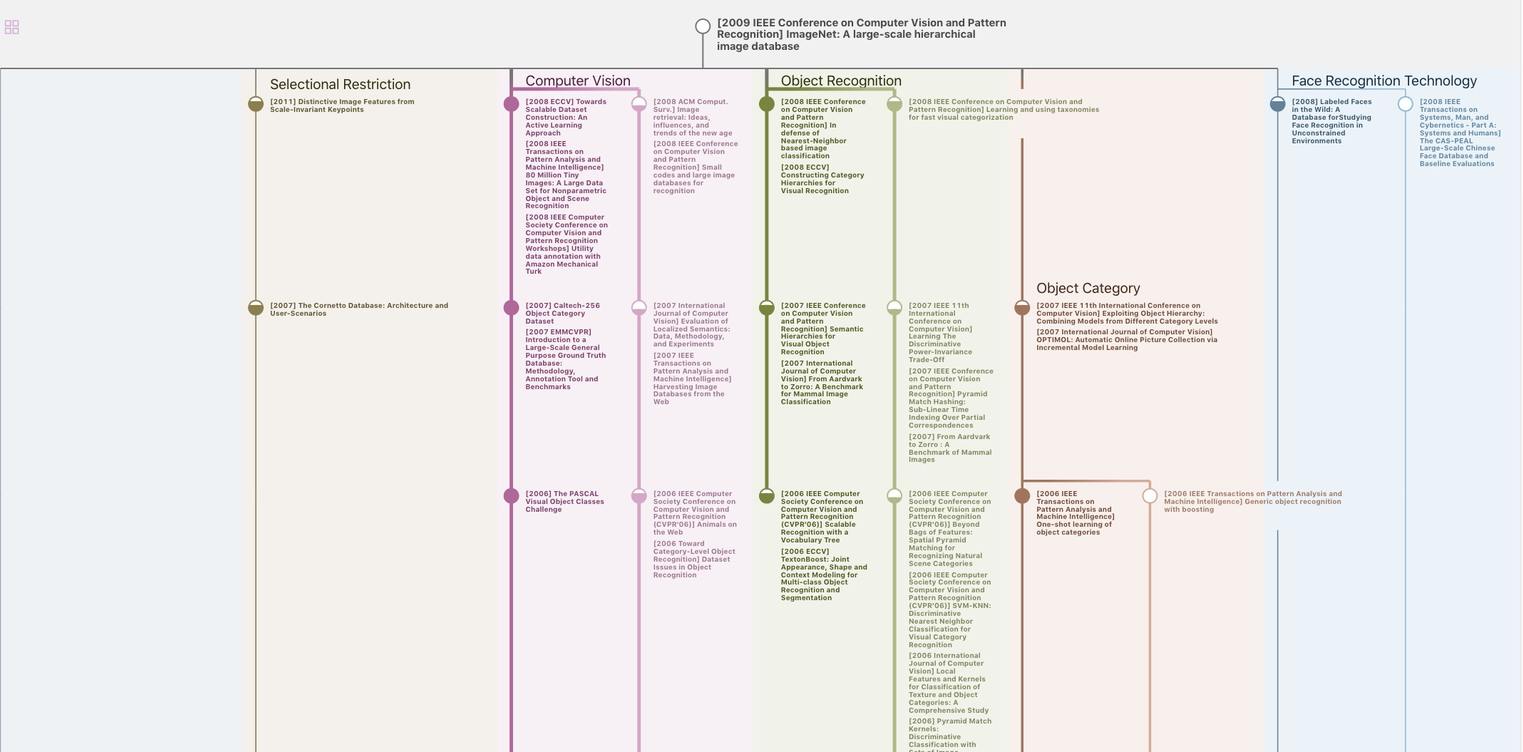
生成溯源树,研究论文发展脉络
Chat Paper
正在生成论文摘要