An Efficient And Simple Graph Model For Scientific Article Cold Start Recommendation
CONCEPTUAL MODELING, ER 2016(2016)
摘要
Since there is little history information for the newly published scientific articles, it is difficult to recommend related new articles for users. Although tags of articles can provide important information for new articles, they are ignored by existing solutions. Moreover, the efficiency of these solutions is unsatisfactory, especially on the big data situation. In this paper, we propose an efficient and simple bi-relational graph for new scientific article recommendation called user-article based graph model with tags (UAGMT), which can integrate various valuable information (e.g., readership, tag, content and citation) into the graph for new article recommendation. Since the structure of the bi-relational graph model is simple and the model incorporates only a few similarity relationships, it can ensure high efficiency. Besides, the tags' information of articles which summarizes the main content is integrated to enhance the reliability of the similarity of articles. It is especially helpful for improving the cold start recommendation performance. A series of experiments on CiteULike dataset show that the recommendation efficiency is greatly improved by using our UAGMT with the guaranteed performance on the cold-start situation.
更多查看译文
关键词
New article recommendation,Cold start problem,Bi-relational graph,Tags' information
AI 理解论文
溯源树
样例
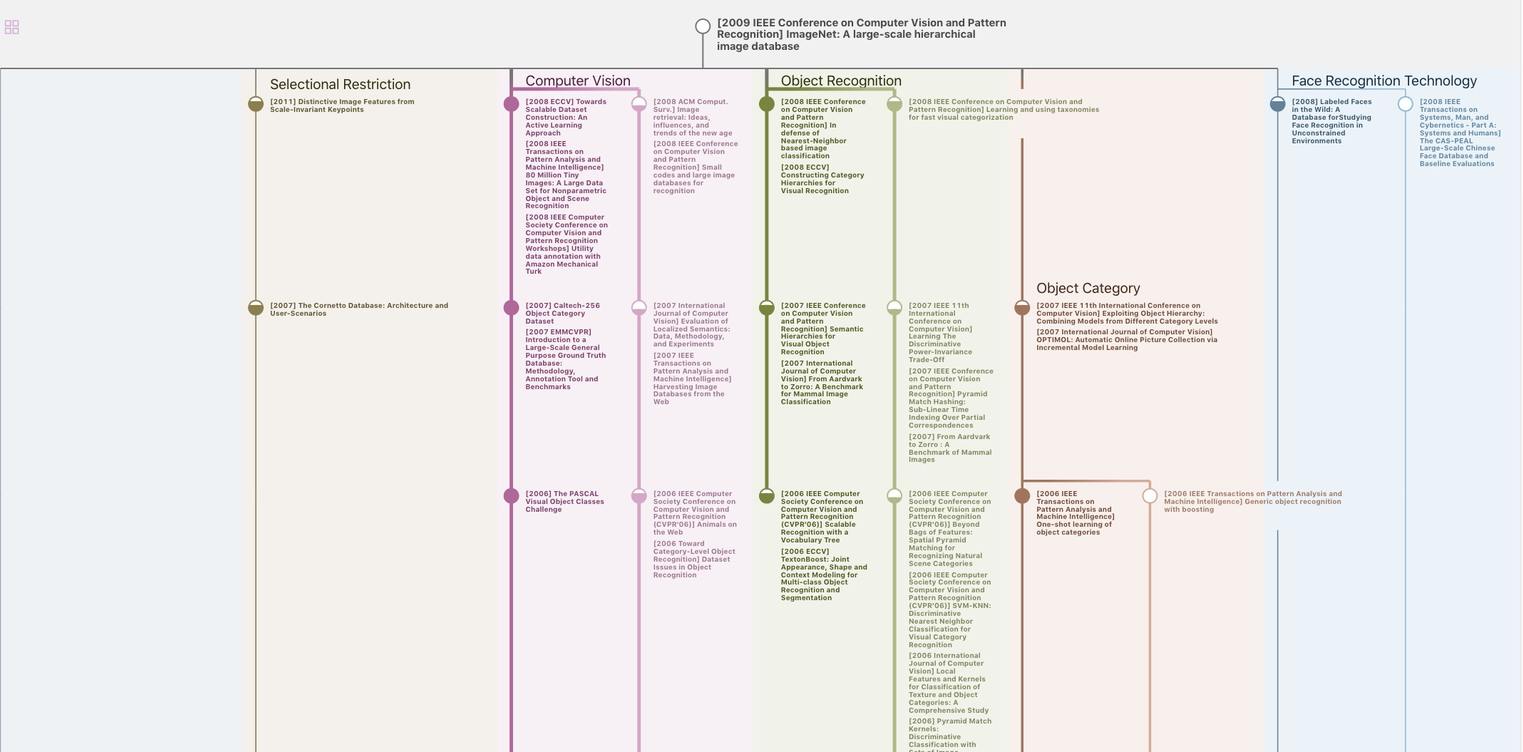
生成溯源树,研究论文发展脉络
Chat Paper
正在生成论文摘要