Learning Functional Argument Mappings for Hierarchical Tasks from Situation Specific Explanations.
Australasian Conference on Artificial Intelligence(2016)
摘要
Hierarchical tasks learnt from situation specific explanations are typically limited in how well they generalise to situations beyond the explanation provided. To address this we present an approach to learning functional argument mappings for enabling task generalisation regardless of explanation specificity. These functional argument mappings allow subtasks within a hierarchical task to utilise both arguments provided to the parent task, as well as domain knowledge, to generalise to novel situations. We validate this approach with a number of scenarios in which the agent learns generalised tasks from situation specific explanations, and show that these tasks provide equal performance when compared to tasks learnt from generalisable explanations.
更多查看译文
AI 理解论文
溯源树
样例
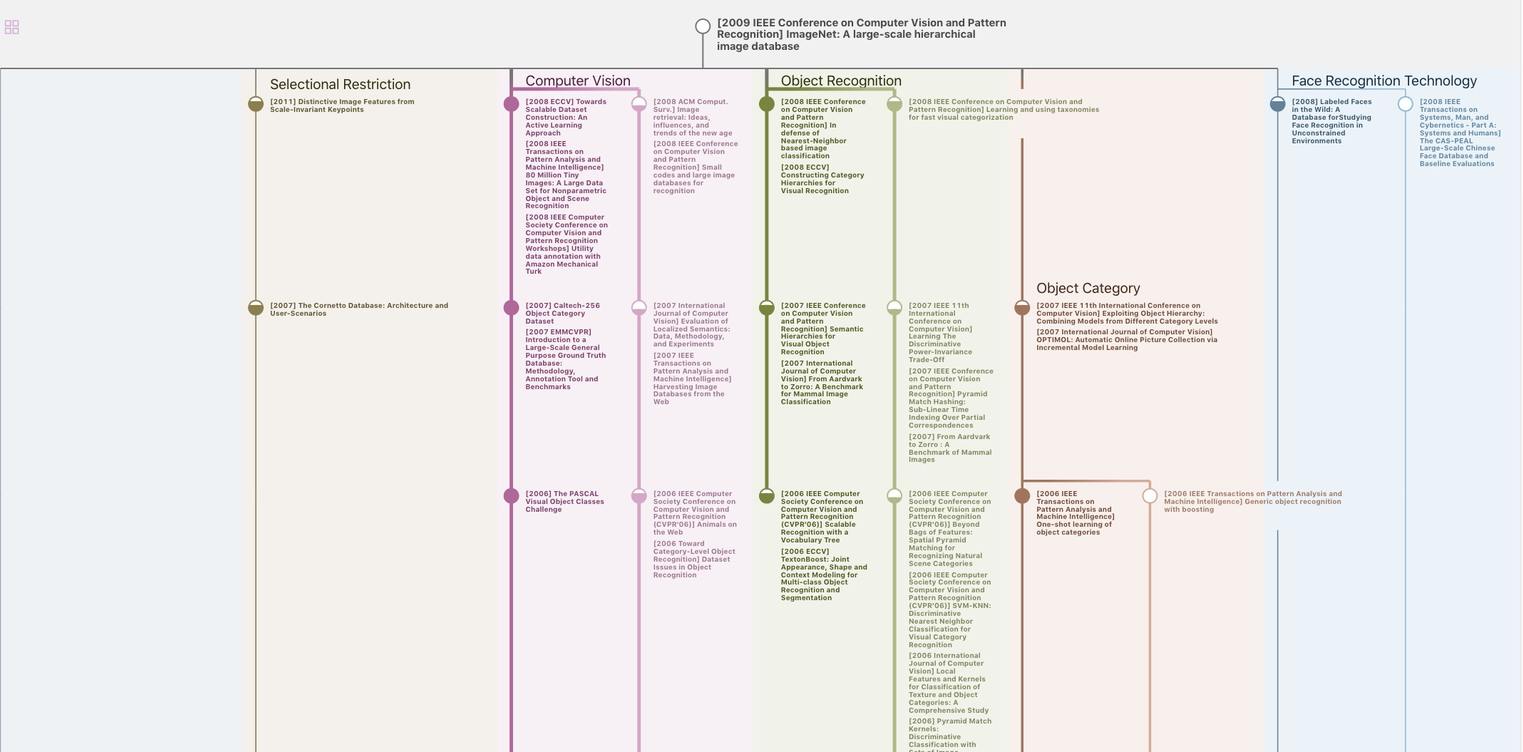
生成溯源树,研究论文发展脉络
Chat Paper
正在生成论文摘要