Representative-Based Active Learning With Max-Min Distance
ROUGH SETS, (IJCRS 2016)(2016)
摘要
Active learning has been a hot topic because labeled data are useful, however expensive. Many existing approaches are based on decision trees, Naive Bayes algorithms, etc. In this paper, we propose a representative-based active learning algorithm with max-min distance. Our algorithm has two techniques interacting with each other. One is the representative-based classification inspired by covering-based neighborhood rough sets. The other is critical instance selection with max-min distance. Experimental results on six UCI datasets indicate that, with the same number of labeled instances, our algorithm is comparable with or better than the ID3, C4.5 and Naive Bayes algorithms.
更多查看译文
关键词
Active learning,Classifier,Distance,Representative,Similarity
AI 理解论文
溯源树
样例
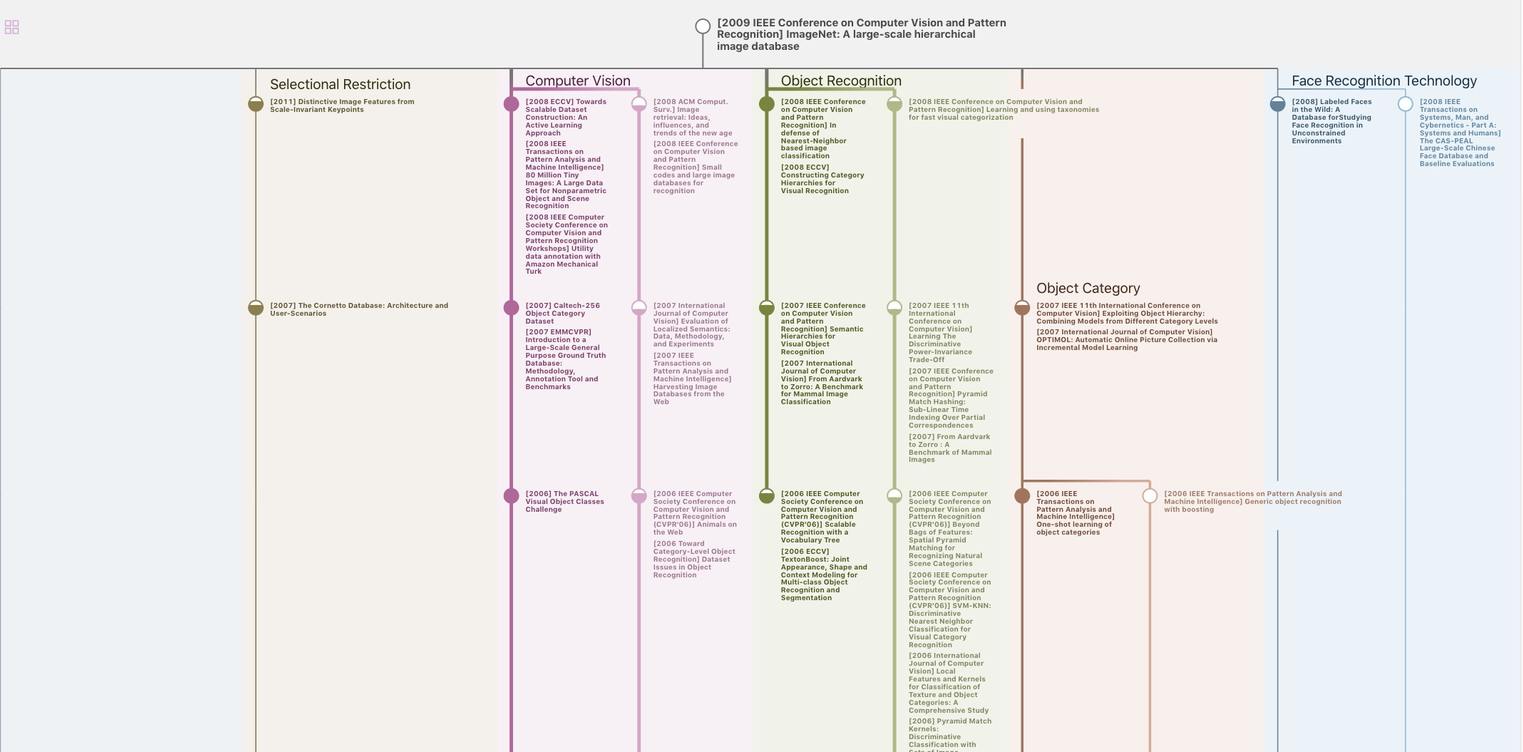
生成溯源树,研究论文发展脉络
Chat Paper
正在生成论文摘要