Fast Supervised Lda For Discovering Micro-Events In Large-Scale Video Datasets
MM '16: ACM Multimedia Conference Amsterdam The Netherlands October, 2016(2016)
摘要
This paper introduces fsLDA, a fast variational inference method for supervised LDA, which overcomes the computational limitations of the original supervised LDA and enables its application in large-scale video datasets. In addition to its scalability, our method also overcomes the drawbacks of standard, unsupervised LDA for video, including its focus on dominant but often irrelevant video information (e.g. background, camera motion). As a result, experiments in the UCF11 and UCF101 datasets show that our method consistently outperforms unsupervised LDA in every metric. Furthermore, analysis shows that class-relevant topics of fsLDA lead to sparse video representations and encapsulate high-level information corresponding to parts of video events, which we denote "micro-events".
更多查看译文
关键词
video event detection,video micro-events,supervised topic modeling,variational inference
AI 理解论文
溯源树
样例
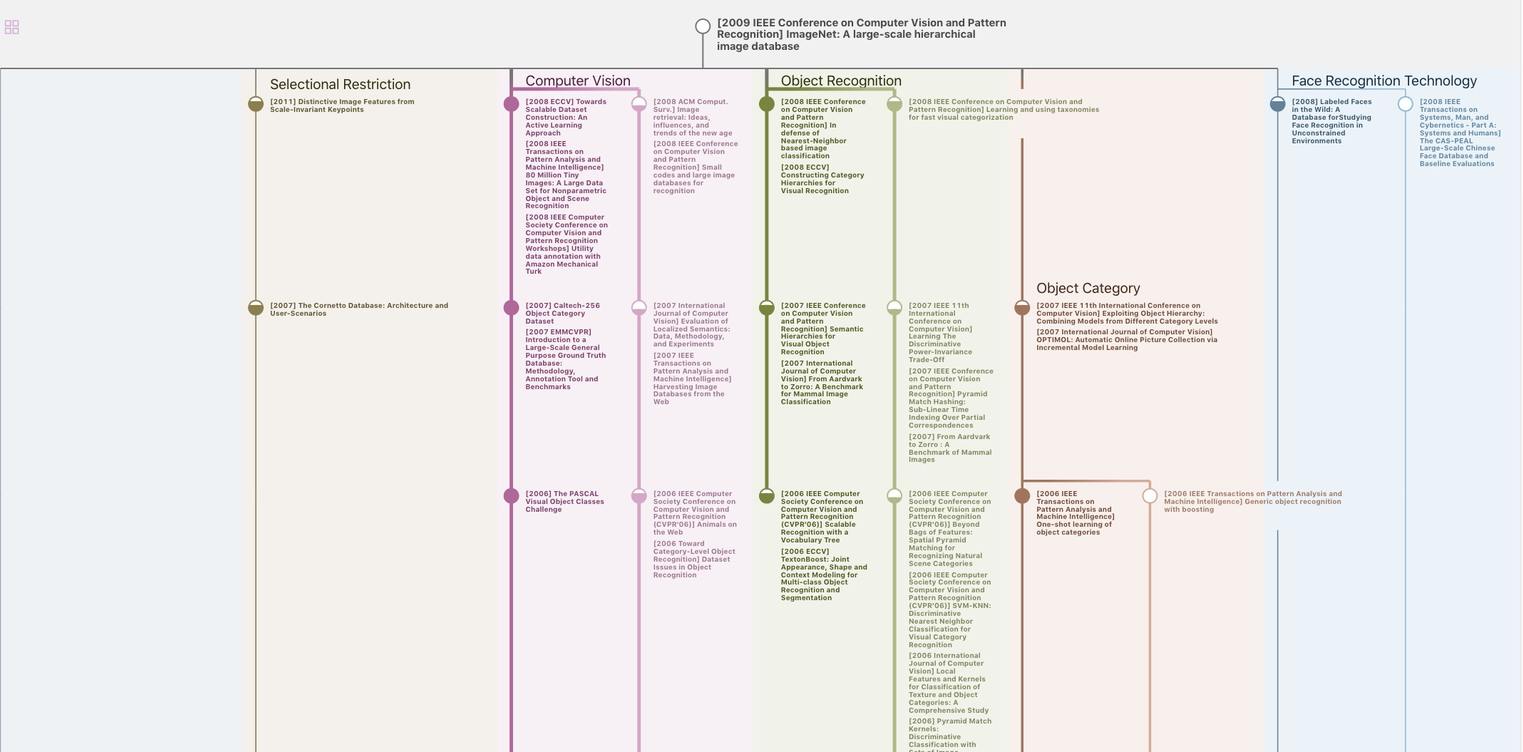
生成溯源树,研究论文发展脉络
Chat Paper
正在生成论文摘要