Single Classifier Selection for Ensemble Learning.
ADMA(2016)
摘要
Ensemble classification is one of representative learning techniques in the field of machine learning, which combines a set of single classifiers together aiming at achieving better classification performance. Not every arbitrary set of single classifiers can obtain a good ensemble classifier. The efficient and necessary condition to construct an accurate ensemble classifier is that the single classifiers should be accurate and diverse. In this paper, we first formally give the definitions of accurate and diverse classifiers and put forward metrics to quantify the accuracy and diversity of the single classifiers; afterwards, we propose a novel parameter-free method to pick up a set of accurate and diverse single classifiers for ensemble. The experimental results on real world data sets show the effectiveness of the proposed method which could improve the performance of the representative ensemble classifier Bagging.
更多查看译文
关键词
single classifier selection,ensemble,learning
AI 理解论文
溯源树
样例
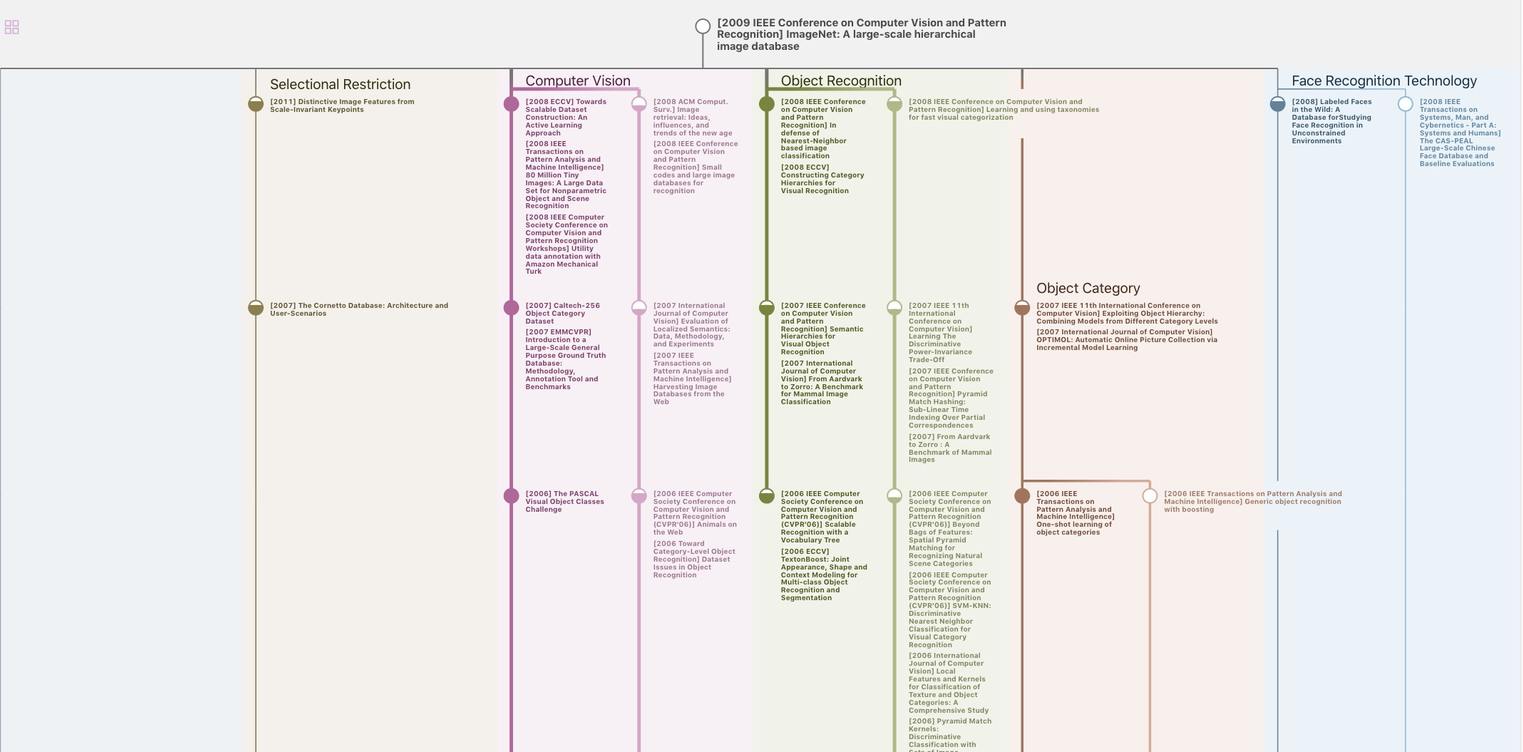
生成溯源树,研究论文发展脉络
Chat Paper
正在生成论文摘要