Exploiting Synonymy And Hypernymy To Learn Efficient Meaning Representations
DIGITAL LIBRARIES: KNOWLEDGE, INFORMATION, AND DATA IN AN OPEN ACCESS SOCIETY(2016)
摘要
Word representation learning methods such as word2vec usually associate one vector per word; however, in order to face polysemy problems, it's important to produce distributed representations for each meaning, not for each surface form of a word. In this paper, we propose an extension for the existing AutoExtend model, an auto-encoder architecture that utilises synonymy relations to learn sense representations. We introduce a new layer in the architecture to exploit hypernymy relations predominantly present in existing ontologies. We evaluate the quality of the obtained vectors on word-sense disambiguation tasks and show that the use of the hypernymy relation leads to improvements of 1.2% accuracy on Senseval-3 and 0.8% on Semeval-2007 English lexical sample tasks, compared to the original model.
更多查看译文
关键词
Sense embedding,Semantic relation,Auto-encoder,Hypernymy,Word-sense disambiguation
AI 理解论文
溯源树
样例
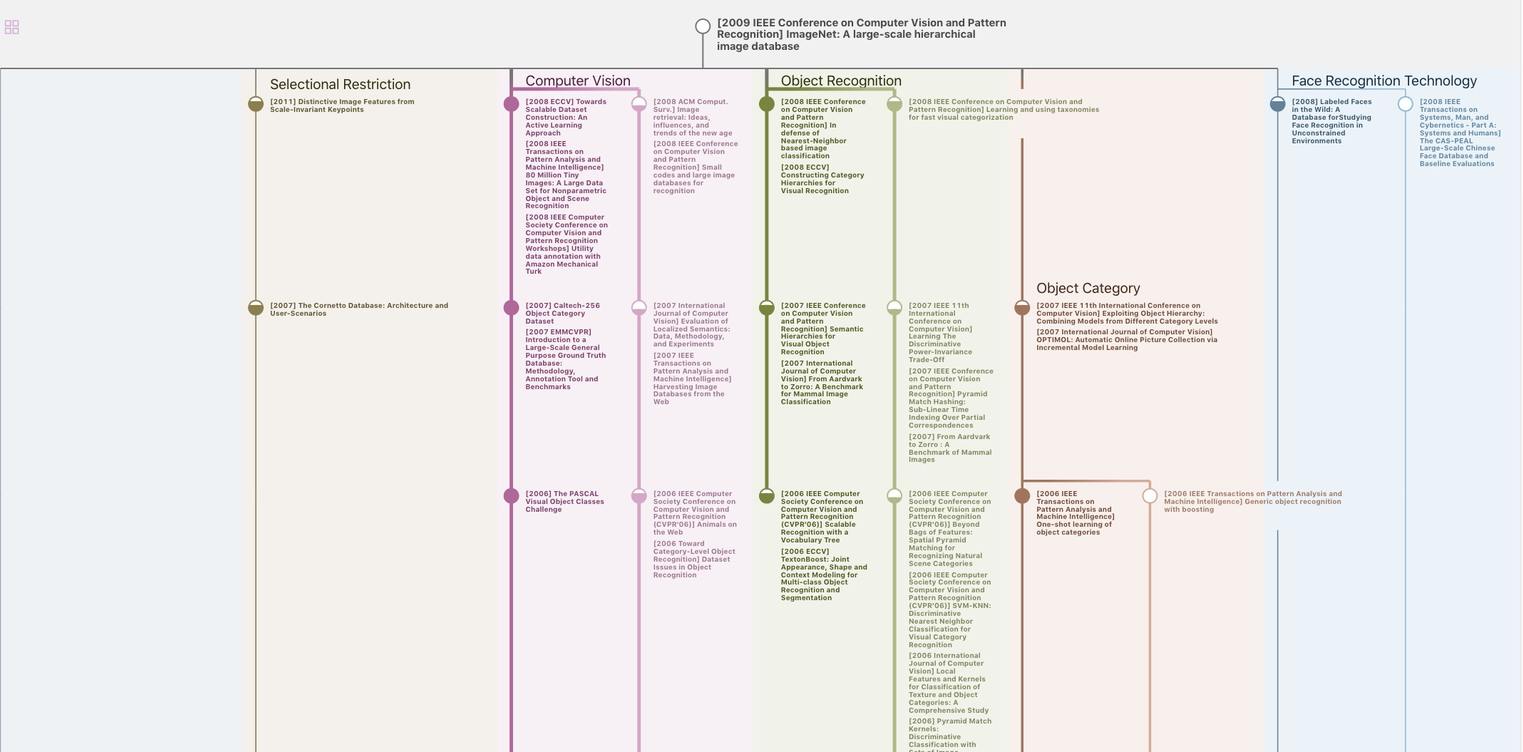
生成溯源树,研究论文发展脉络
Chat Paper
正在生成论文摘要