Granularity and the cost of error recovery in resilient AMR scientific applications.
SC(2016)
摘要
Supercomputing platforms are expected to have larger failure rates in the future because of scaling and power concerns. The memory and performance impact may vary with error types and failure modes. Therefore, localized recovery schemes will be important for scientific computations, including failure modes where application intervention is suitable for recovery. We present a resiliency methodology for applications using structured adaptive mesh refinement, where failure modes map to granularities within the application for detection and correction. This approach also enables parameterization of cost for differentiated recovery. The cost model is built with tuning parameters that can be used to customize the strategy for different failure rates in different computing environments. We also show that this approach can make recovery cost proportional to the failure rate.
更多查看译文
关键词
Scientific computing,High performance computing
AI 理解论文
溯源树
样例
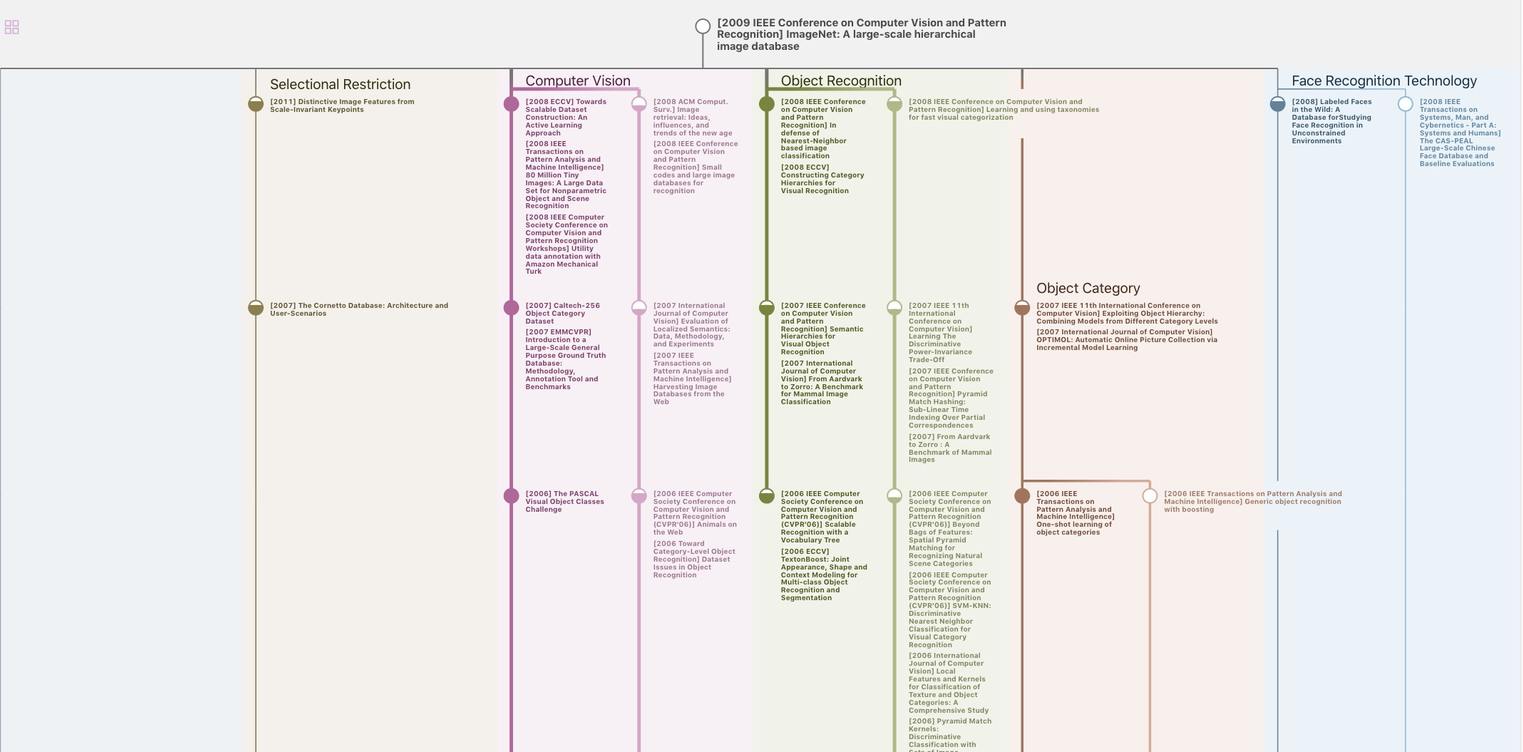
生成溯源树,研究论文发展脉络
Chat Paper
正在生成论文摘要