A Novel Mutual-Information-Guided Sparse Feature Selection Approach For Epilepsy Diagnosis Using Interictal Eeg Signals
BRAIN INFORMATICS AND HEALTH(2016)
摘要
Diagnosing people with possible epilepsy has major implications for their health, occupation, driving and social interactions. The current epilepsy diagnosis procedure is often subject to errors with considerable interobserver variations by manually observing long-term lengthy EEG recordings that require the presence of seizure (ictal) activities. It is costly and often difficult to obtain long-term EEG data with seizure activities that imped epilepsy diagnosis for many people, in particular in areas that lack of medical resources and well-trained neurologists. There is a desperate need for a new diagnostic tool that is capable of providing quick and accurate epilepsy-screening using short-term interictal EEG signals. However, it is challenging to analyze interictal EEG recordings when patients behaviors same as normal subjects. This research is dedicated to develop new automatic data-driven pattern recognition system for interictal EEG signals and design a quick screening process to help neurologists diagnose patients with epilepsy. In particular, we propose a novel information-theory-guided spare feature selection framework to select the most important EEG features to discriminate epileptic or non-epileptic EEG patterns accurately. The proposed approach were tested on an EEG dataset with 11 patients and 11 normal subjects, achieved an impressive diagnostic accuracy of 90% based on visually-evoked potentials in a human-computer task. This preliminary study indicates that it is promising to provide fast, reliable, and affordable epilepsy diagnostic solutions using short-term interictal EEG signals.
更多查看译文
关键词
Feature Selection, Mutual Information, Discrete Fourier Transform, Feature Subset, Contrast Level
AI 理解论文
溯源树
样例
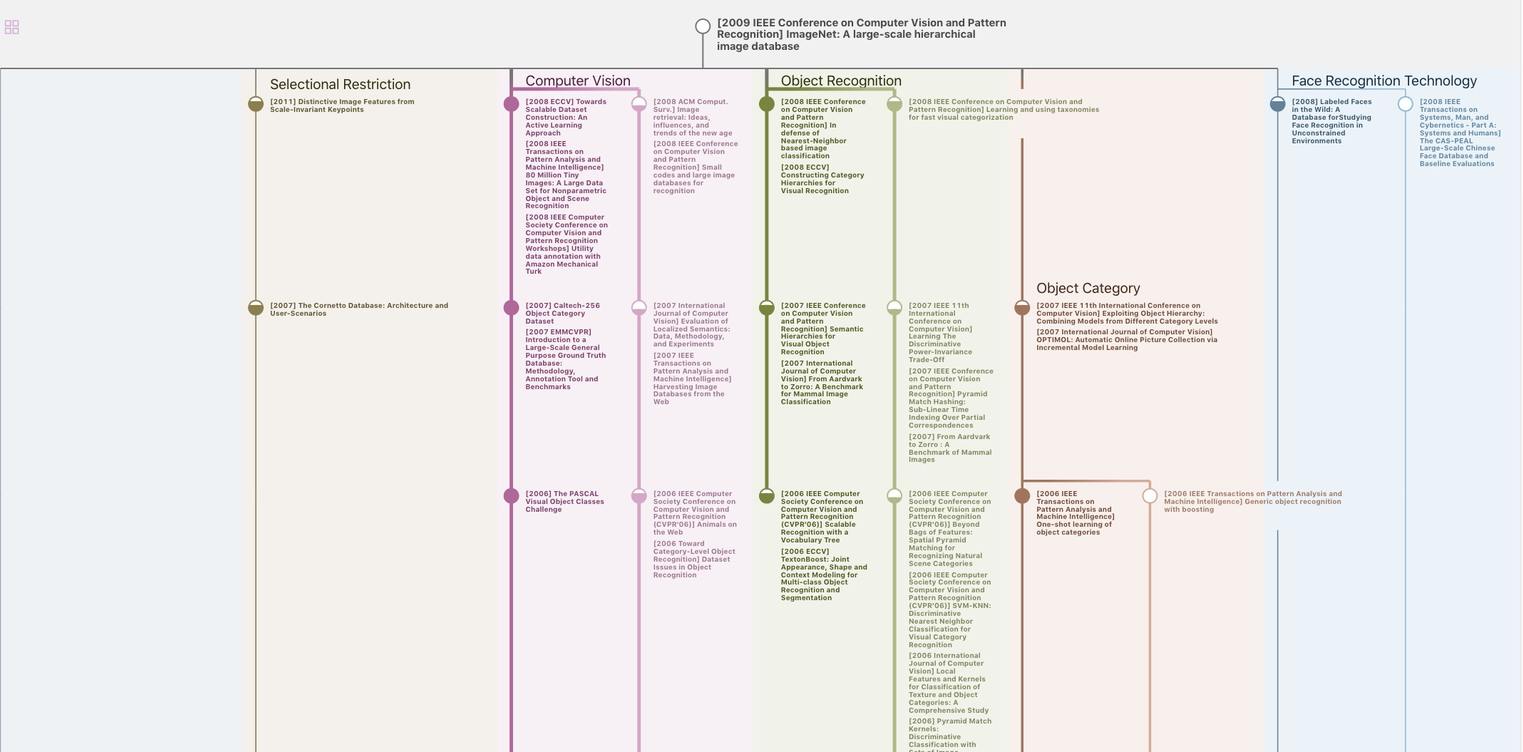
生成溯源树,研究论文发展脉络
Chat Paper
正在生成论文摘要